Probabilistic machine learning ensures accurate ambient denoising in droplet-based single-cell omics
semanticscholar(2022)
摘要
Droplet-based single-cell omics, including single-cell RNA sequencing (scRNAseq), single-cell CRISPR perturbations (e.g., CROP-seq), and single-cell protein and transcriptomic profiling (CITE-seq) hold great promise for comprehensive cell profiling and genetic screening at the single-cell resolution. However, these technologies suffer from substantial noise, among which ambient signals present in the cell suspension may be the predominant source. Current models to address this issue are highly technology-specific and relatively scRNAseq-centric. while a universal model to describe the noise across these technologies may reveal this common source, improving the denoising accuracy. To this end, we explicitly examined these unexpected signals in multiple datasets across droplet-based technologies, summarised a predictable pattern, and developed single-cell Ambient Remover (scAR) – a hypothesis-driven machine learning model to predict and remove ambient signals (including mRNA counts, protein counts, and sgRNA counts) at the molecular level. We benchmarked scAR on three technologies – single-cell CRISPR screens, CITEseq, and scRNAseq along with the state-of-the-art single-technology-specific approaches. scAR showed high denoising accuracy for each type of contamination ratios. However, droplet I and II lead to too high estimation of human-sourced contamination, as much as ~3x of mouse source. This may be explained by the over-representation of human transcripts in droplet I and II ( d ). The higher human transcripts in ambient frequencies as input, the more counts to be identified as background noise by scAR. The best estimate of ambient frequencies should be drawn from the population of cell-free droplets, as the estimated noise ratios are in a reasonable range in both cell lines – ambient signals from human sources are slightly stronger than mouse sources in both cell lines. These observations also suggest that compositions in these droplets are clearly different, e.g., droplet I and II may contain more human cell debris.
更多查看译文
关键词
accurate ambient denoising,probabilistic machine learning,machine learning,droplet-based,single-cell
AI 理解论文
溯源树
样例
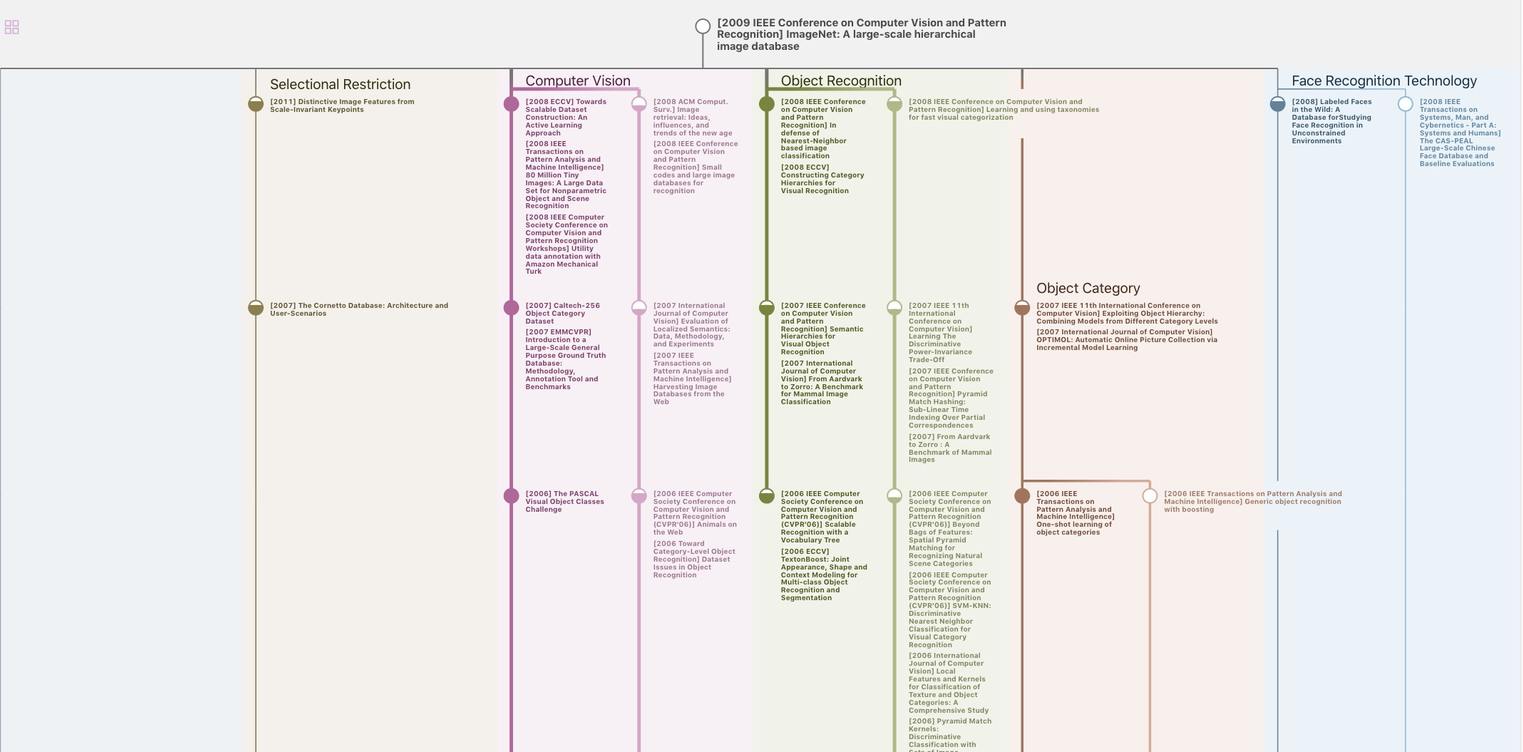
生成溯源树,研究论文发展脉络
Chat Paper
正在生成论文摘要