Probing Compositional Inference in Natural and Artificial Agents
semanticscholar(2022)
摘要
People can easily evoke previously encountered concepts, compose them, and apply the result to novel contexts in a zero-shot manner. What computational mechanisms underpin this ability? To study this question, we propose an extension to the structured multi-armed bandit paradigm, which has been used to probe human function learning in previous works. This new paradigm involves a learning curriculum where agents first perform two sub-tasks in which rewards were sampled from differently structured reward functions, followed by a third sub-task in which rewards were set to a composition of the previously encountered reward functions. This setup allows us to investigate how people reason compositionally over learned functions, while still being simple enough to be tractable. Human behavior in such tasks has been predominantly modeled by computational models with hard-coded structures such as Bayesian grammars. We indeed find that such a model performs well on our task. However, they do not explain how people learn to compose reward functions via trial and error but have, instead, been hand-designed to generalize compositionally by expert researchers. How could the ability to compose ever emerge through trial and error? We propose a model based on the principle of meta-learning to tackle this challenge and find that – upon training on the previously described curriculum – meta-learned agents exhibit characteristics comparable to those of a Bayesian agent with compositional priors. Model simulations suggest that both models can compose earlier learned functions to generalize in a zero-shot manner. We complemented these model simulations results with a behavioral study, in which we investigated how human participants approach our task. We find that they are indeed able to perform zero-shot compositional reasoning as predicted by our models. Taken together, our study paves a way for studying compositional reinforcement learning in humans, symbolic, and sub-symbolic agents.
更多查看译文
AI 理解论文
溯源树
样例
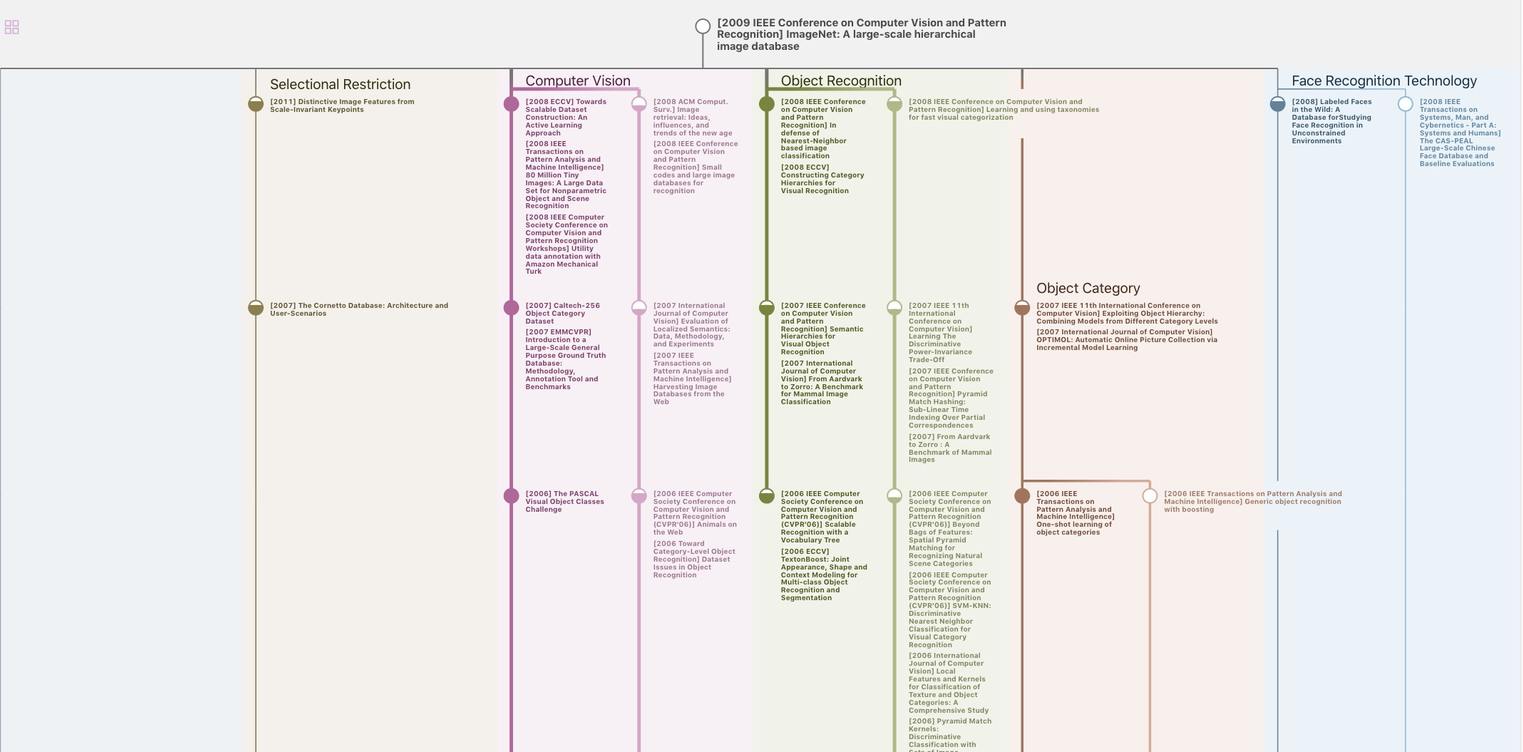
生成溯源树,研究论文发展脉络
Chat Paper
正在生成论文摘要