External control arm analysis: an evaluation of propensity score approaches, G-computation, and doubly debiased machine learning
medRxiv(2022)
摘要
Background An external control arm is a cohort of control patients that are collected from data external to a single-arm trial. To provide an unbiased estimation of efficacy, the clinical profiles of patients from single and external arms should be aligned, typically using propensity score approaches. There are alternative approaches to infer efficacy based on comparisons between outcomes of single-arm patients and machine-learning predictions of control patient outcomes. These methods include G-computation and Doubly Debiased Machine Learning (DDML) and their evaluation for External Control Arms (ECA) analysis is insufficient. Methods We consider both numerical simulations and a trial replication procedure to evaluate the different statistical approaches: propensity score matching, Inverse Probability of Treatment Weighting (IPTW), G-computation, and DDML. The replication study relies on five type 2 diabetes randomized clinical trials granted by the Yale University Open Data Access (YODA) project. From the pool of five trials, observational experiments are artificially built by replacing a control arm from one trial by an arm originating from another trial and containing similarly-treated patients. Results Among the different statistical approaches, numerical simulations show that DDML has the smallest bias followed by G-computation. In terms of mean squared error, G-computation usually minimizes mean squared error. Compared to other methods, DDML has varying Mean Squared Error performances that improves with increasing sample sizes. For hypothesis testing, all methods control type I error and DDML is the most conservative. G-computation is the best method in terms of statistical power, and DDML has comparable power at n=1000 but inferior ones for smaller sample sizes. The replication procedure also indicates that G-computation minimizes mean squared error whereas DDML has intermediate performances in between G-computation and propensity score approaches. The confidence intervals of G-computation are the narrowest whereas confidence intervals obtained with DDML are the widest for small sample sizes, which confirms its conservative nature. Conclusions For external control arm analyses, methods based on outcome prediction models can reduce estimation error and increase statistical power compared to propensity score approaches.
更多查看译文
关键词
Observational study,Average treatment effect,Propensity score,Confounding variables,Replication study,Counterfactual,Doubly robust
AI 理解论文
溯源树
样例
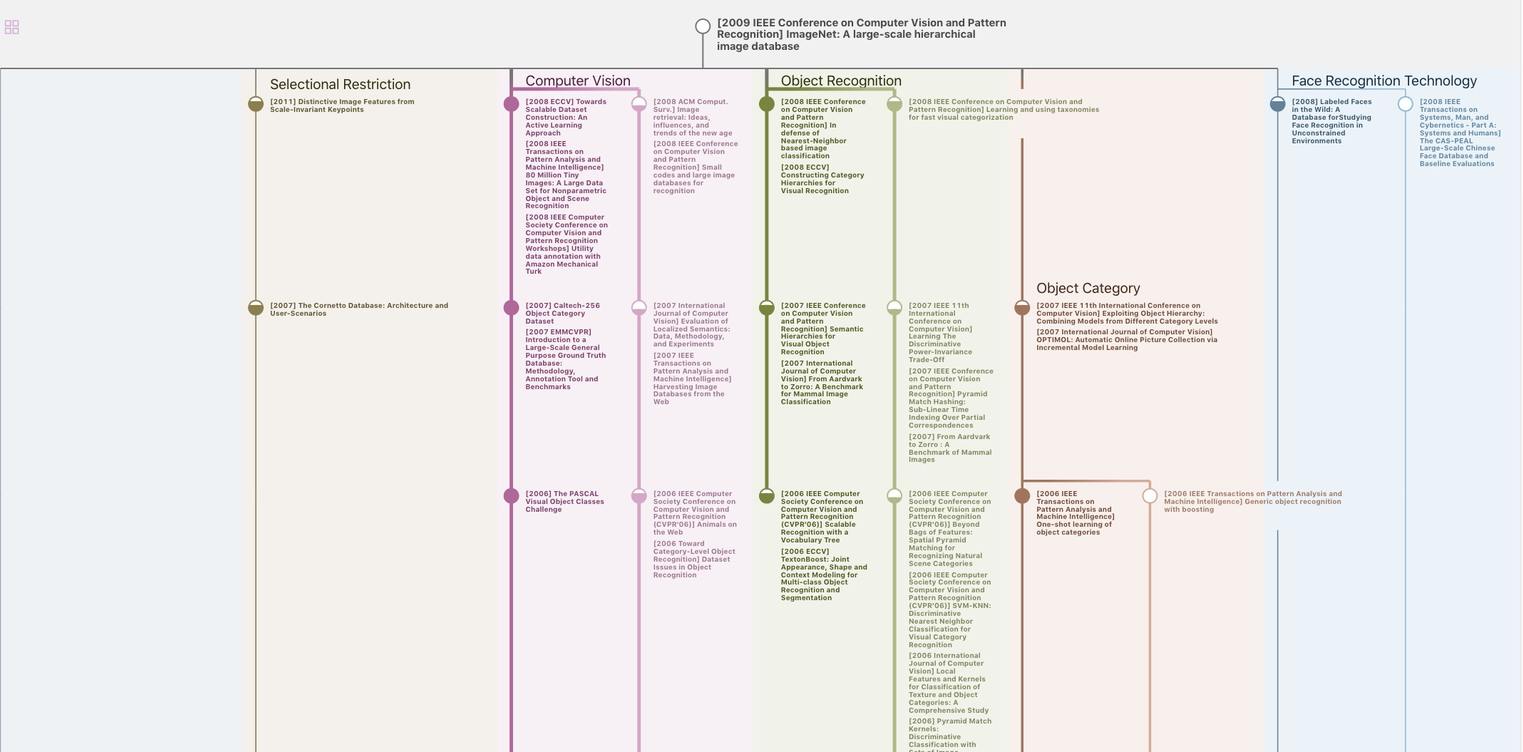
生成溯源树,研究论文发展脉络
Chat Paper
正在生成论文摘要