Distinguishing Lewy Body Dementia from Alzheimer's Disease using Machine Learning on Heterogeneous Data: A Feasibility Study.
2022 44th Annual International Conference of the IEEE Engineering in Medicine & Biology Society (EMBC)(2022)
摘要
Dementia with Lewy Bodies (DLB) is the second most common form of dementia, but diagnostic markers for DLB can be expensive and inaccessible, and many cases of DLB are undiagnosed. This work applies machine learning techniques to determine the feasibility of distinguishing DLB from Alzheimer's Disease (AD) using heterogeneous data features. The Repeated Incremental Pruning to Produce Error Reduction (RIPPER) algorithm was first applied using a Leave-One-Out Cross-Validation protocol to a dataset comprising DLB and AD cases. Then, interpretable association rule-based diagnostic classifiers were obtained for distinguishing DLB from AD. The various diagnostic classifiers generated by this process had high accuracy over the whole dataset (mean accuracy of 94%). The mean accuracy in classifying their out-of-sample case was 80.5%. Every classifier generated consisted of very simple structure, each using 1-2 classification rules and 1-3 data features. As a group, the classifiers were heterogeneous and used several different data features. In particular, some of the classifiers used very simple and inexpensive diagnostic features, yet with high diagnostic accuracy. This work suggests that opportunities may exist for incorporating accessible diagnostic assessments while improving diagnostic rate for DLB. Clinical Relevance- Simple and interpretable high-performing machine learning algorithms identified a variety of readily available clinical assessments for differential diagnosis of dementia offering the opportunities to incorporate various simple and inexpensive screening tests for DLB and addressing the problem of DLB underdiagnosis.
更多查看译文
关键词
lewy body dementia,alzheimer,machine learning,heterogeneous data
AI 理解论文
溯源树
样例
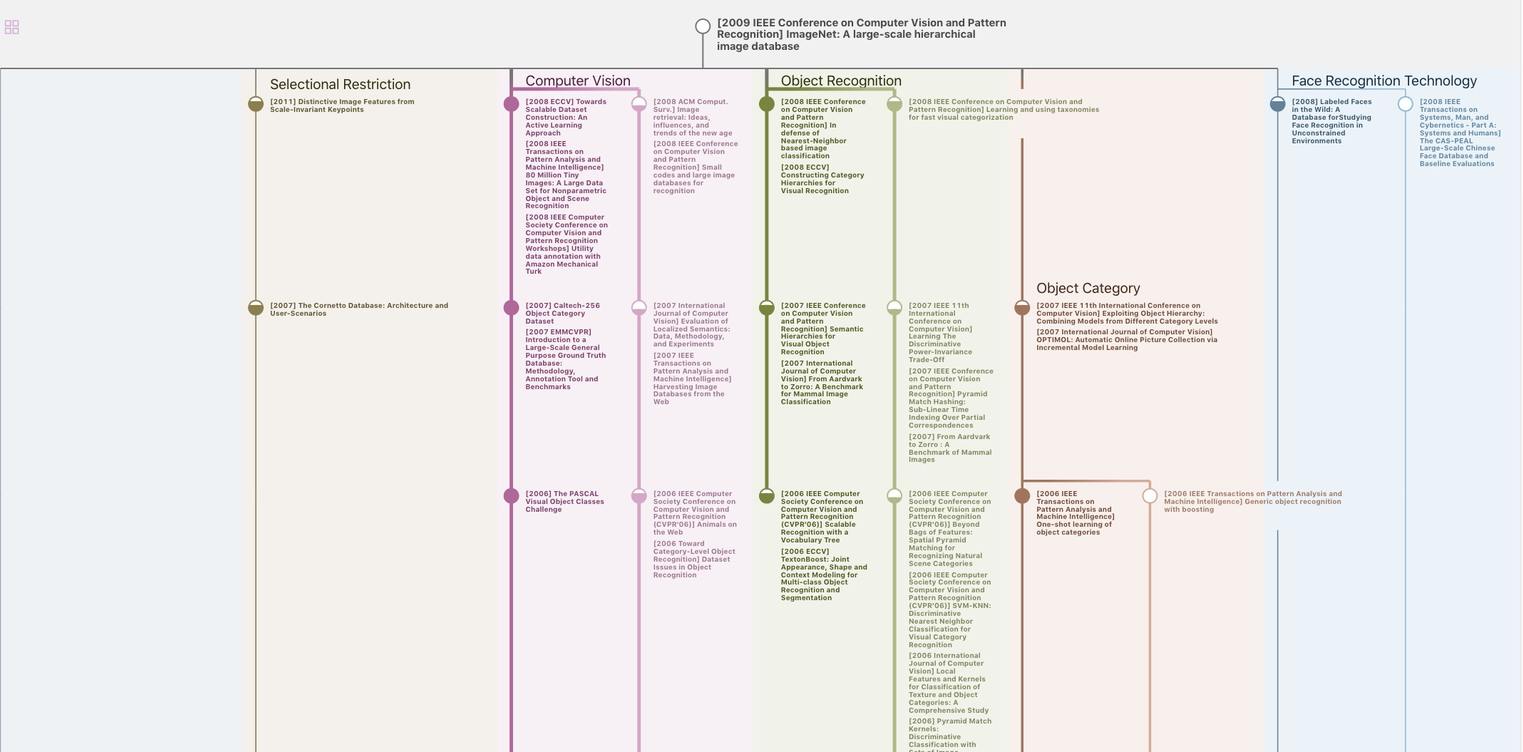
生成溯源树,研究论文发展脉络
Chat Paper
正在生成论文摘要