Complex Video Action Reasoning via Learnable Markov Logic Network
2022 IEEE/CVF CONFERENCE ON COMPUTER VISION AND PATTERN RECOGNITION (CVPR 2022)(2022)
摘要
Profiting from the advance of deep convolutional networks, current state-of-the-art video action recognition models have achieved remarkable progress. Nevertheless, most of existing models suffer from low interpretability of the predicted actions. Inspired by the observation that temporally-configured human-object interactions often serve as a key indicator of many actions, this work crafts an action reasoning framework that performs Markov Logic Network (MLN) based probabilistic logical inference. Crucially, we propose to encode an action by first-order logical rules that correspond to the temporal changes of visual relationships in videos. The main contributions of this work are two-fold: 1) Different from existing black-box models, the proposed model simultaneously implements the localization of temporal boundaries and the recognition of action categories by grounding the logical rules of MLN in videos. The weight associated with each such rule further provides an estimate of confidence. These collectively make our model more explainable and robust. 2) Instead of using hand-crafted logical rules in conventional MLN, we develop a data-driven instantiation of the MLN. In specific, a hybrid learning scheme is proposed. It combines MLN's weight learning and reinforcement learning, using the former's results as a self-critic for guiding the latter's training. Additionally, by treating actions as logical predicates, the proposed framework can also be integrated with deep models for further performance boost. Comprehensive experiments on two complex video action datasets (Charades & CAD-120) clearly demonstrate the effectiveness and explainability of our proposed method.
更多查看译文
关键词
Video analysis and understanding,Explainable computer vision
AI 理解论文
溯源树
样例
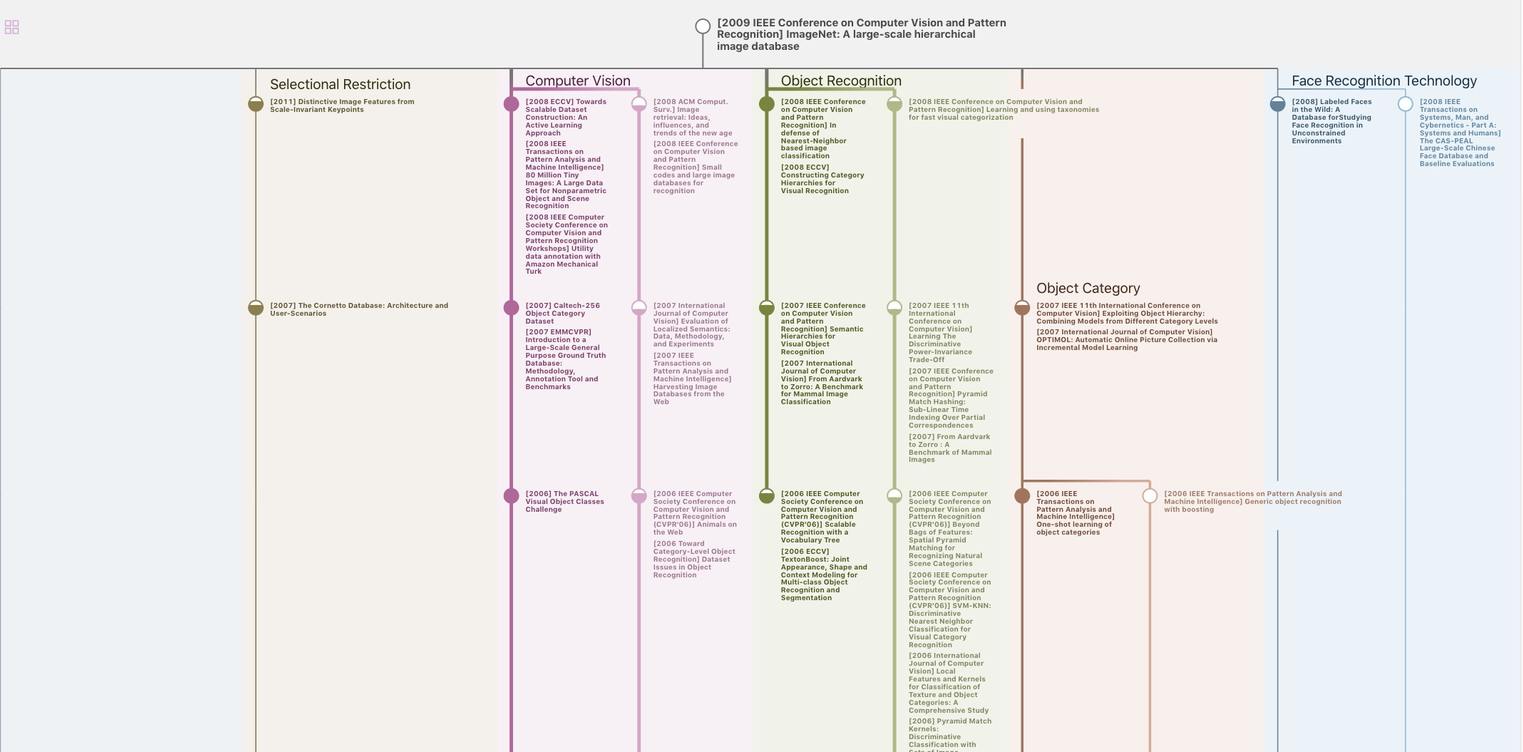
生成溯源树,研究论文发展脉络
Chat Paper
正在生成论文摘要