Fault Diagnosis Based on Attention Collaborative LSTM Networks for NPC Three-Level Inverters
IEEE TRANSACTIONS ON INSTRUMENTATION AND MEASUREMENT(2022)
摘要
To address the problem, it is difficult to extract fault features for neutral-point-clamped (NPC) three-level inverters under nonstationary conditions. This article proposes a multi-information feature fusion diagnosis method based on attention collaborative stacked long short-term memory (ASLSTM) neural networks. First, parallel structural stacked LSTM networks are constructed, which are used to automatically extract features from multisource time-series data. Then, the attention mechanism is applied to weight these features adaptively. Finally, the fault is identified by features that integrate multiple sources of information. In addition, the quantum particle swarm optimization (QPSO) algorithm is used to intelligently tune the hyperparameters of ASLSTM to improve the reasonableness of hyperparameter selection for the diagnostic model. The simulation results of the fault diagnosis of the NPC three-level inverter circuit show that the proposed method is able to extract high-discriminative features from raw data and has better diagnostic results under various conditions compared with other schemes.
更多查看译文
关键词
Circuit faults,Feature extraction,Fault diagnosis,Inverters,Data mining,Mathematical models,Integrated circuit modeling,Attention mechanism,fault diagnosis,neutral-point-clamped (NPC) three-level inverters,parallel structural stacked long short-term memory (LSTM) networks,quantum particle swarm algorithm (QPSO)
AI 理解论文
溯源树
样例
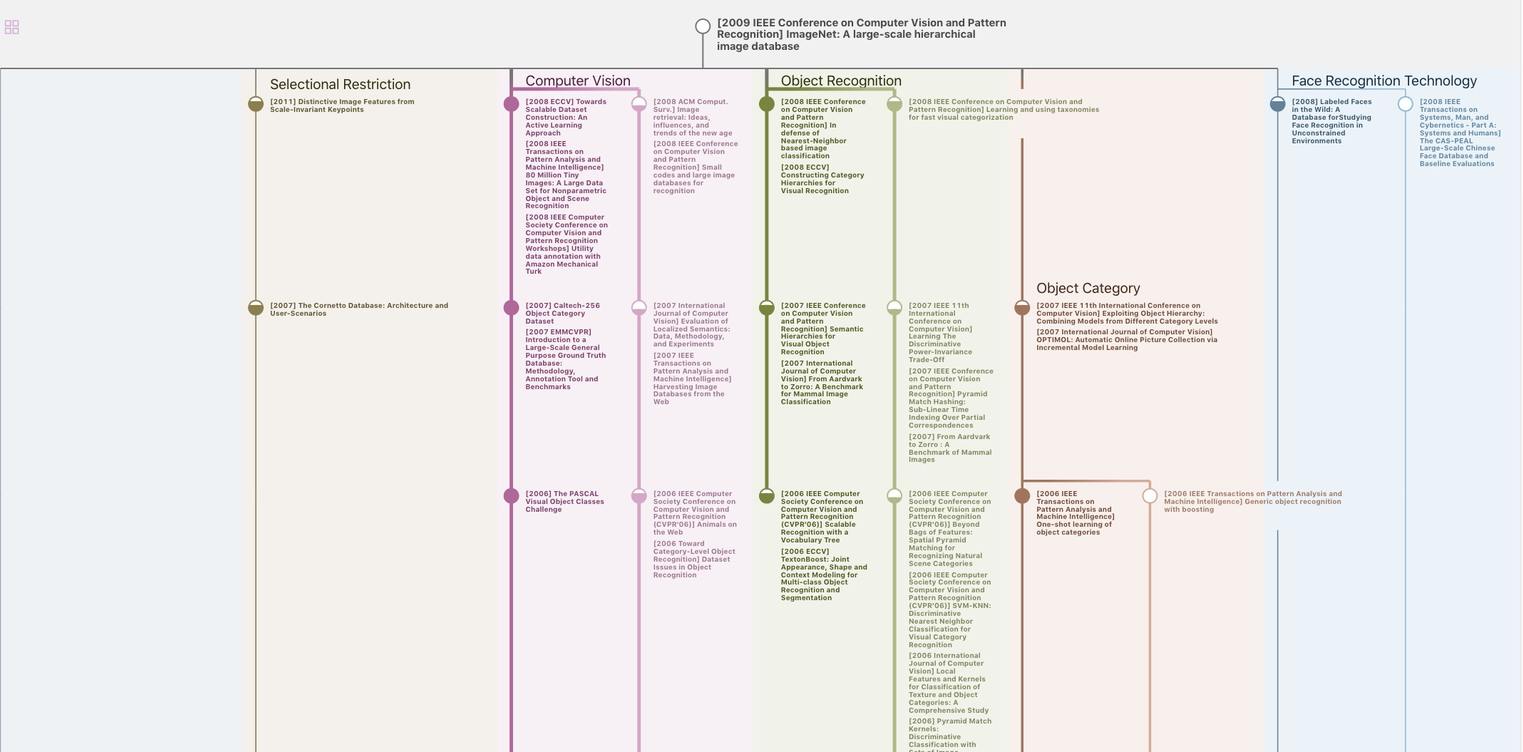
生成溯源树,研究论文发展脉络
Chat Paper
正在生成论文摘要