Abstra: Toward Generic Abstractions for Data of Any Model.
International Conference on Information and Knowledge Management (CIKM)(2022)
摘要
Digital data sharing leads to unprecedented opportunities to develop data-driven systems for supporting economic activities, the social and political life, and science. Many open-access datasets are RDF (Linked Data) graphs, but others are JSON or XML documents, CSV files, Neo4J property graphs, etc. Potential users need to understand a dataset in order to decide if it is useful for their goal. While some published datasets come with a schema and/or documentation, this is not always the case. We demonstrate Abstra, a dataset abstraction system, which applies on a large variety of data models. Abstra computes a description meant for humans, and integrates Information Extraction to classify dataset content among a set of categories of interest to the user. Our abstractions are conceptually close to Entity-Relationship diagrams, but our entities can have deeply nested structure.
更多查看译文
关键词
generic abstractions,model,data
AI 理解论文
溯源树
样例
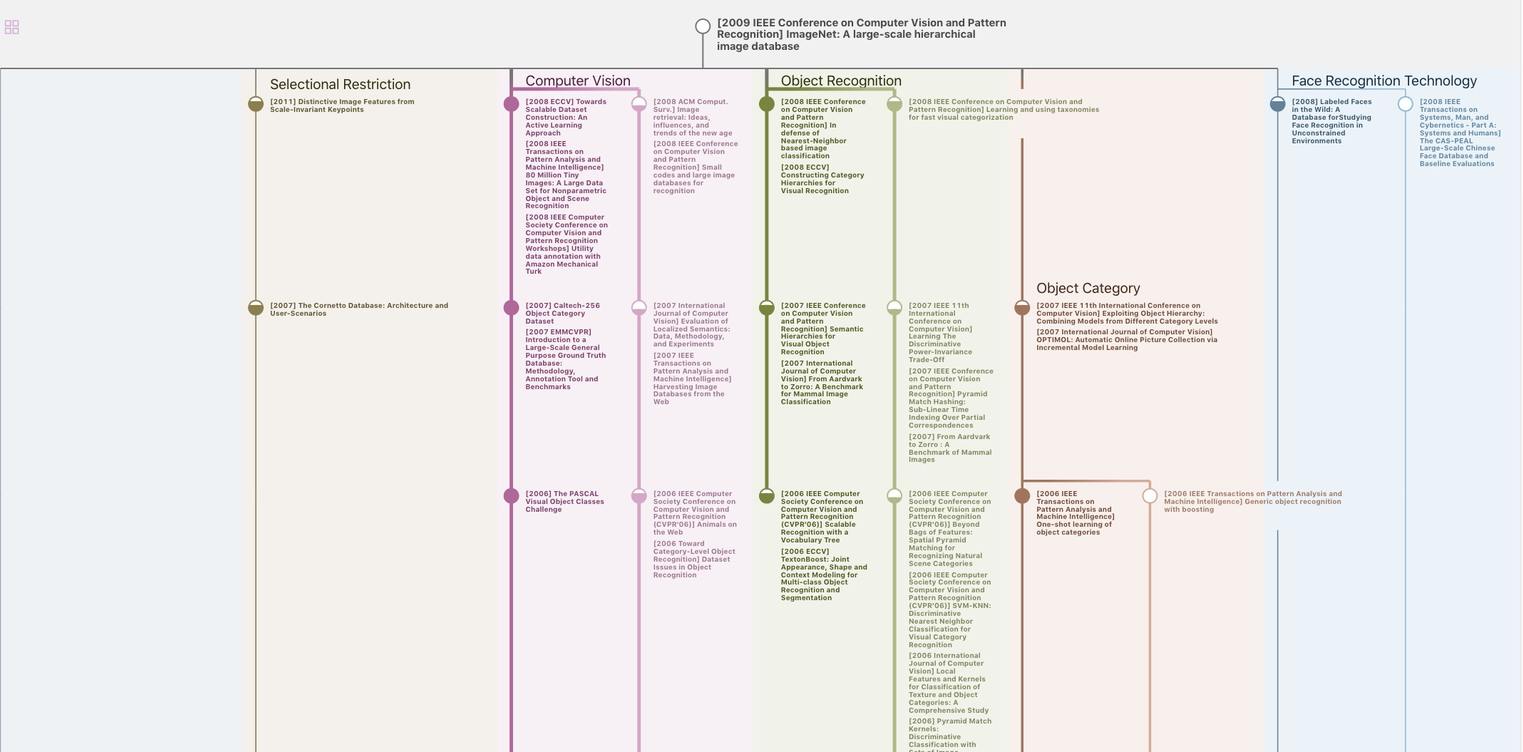
生成溯源树,研究论文发展脉络
Chat Paper
正在生成论文摘要