FlowShark: Sampling for High Flow Visibility in SDNs
IEEE Conference on Computer Communications (INFOCOM)(2022)
摘要
As the scale and speed of modern networks continue to increase, traffic sampling has become an indispensable tool in network management. While there exist a plethora of sampling solutions, they either provide limited flow visibility or have poor scalability in large networks. This paper presents the design and evaluation of FlowShark, a high-visibility per-flow sampling system for Software-Defined Networks (SDNs). The key idea in FlowShark is to separate sampling decisions on short and long flows, whereby sampling short flows is managed locally on edge switches, while a central controller optimizes sampling decisions on long flows. To this end, we formulate flow sampling as an optimization problem and design an online algorithm with a bounded competitive ratio to solve the problem efficiently. To show the feasibility of our design, we have implemented FlowShark in a small OpenFlow network using Mininet. We present experimental results of our Mininet implementation as well as performance benchmarks obtained from packet-level simulations in larger networks. Our experiments with a machine learning based Traffic Classifier application show up to 27% and 19% higher classification recall and precision, respectively, with FlowShark compared to existing sampling approaches.
更多查看译文
关键词
high flow visibility,traffic sampling,network management,per-flow sampling system,software-defined networks,separate sampling decisions,optimization problem,bounded competitive ratio,OpenFlow network,FlowShark,SDN,traffic classifier application
AI 理解论文
溯源树
样例
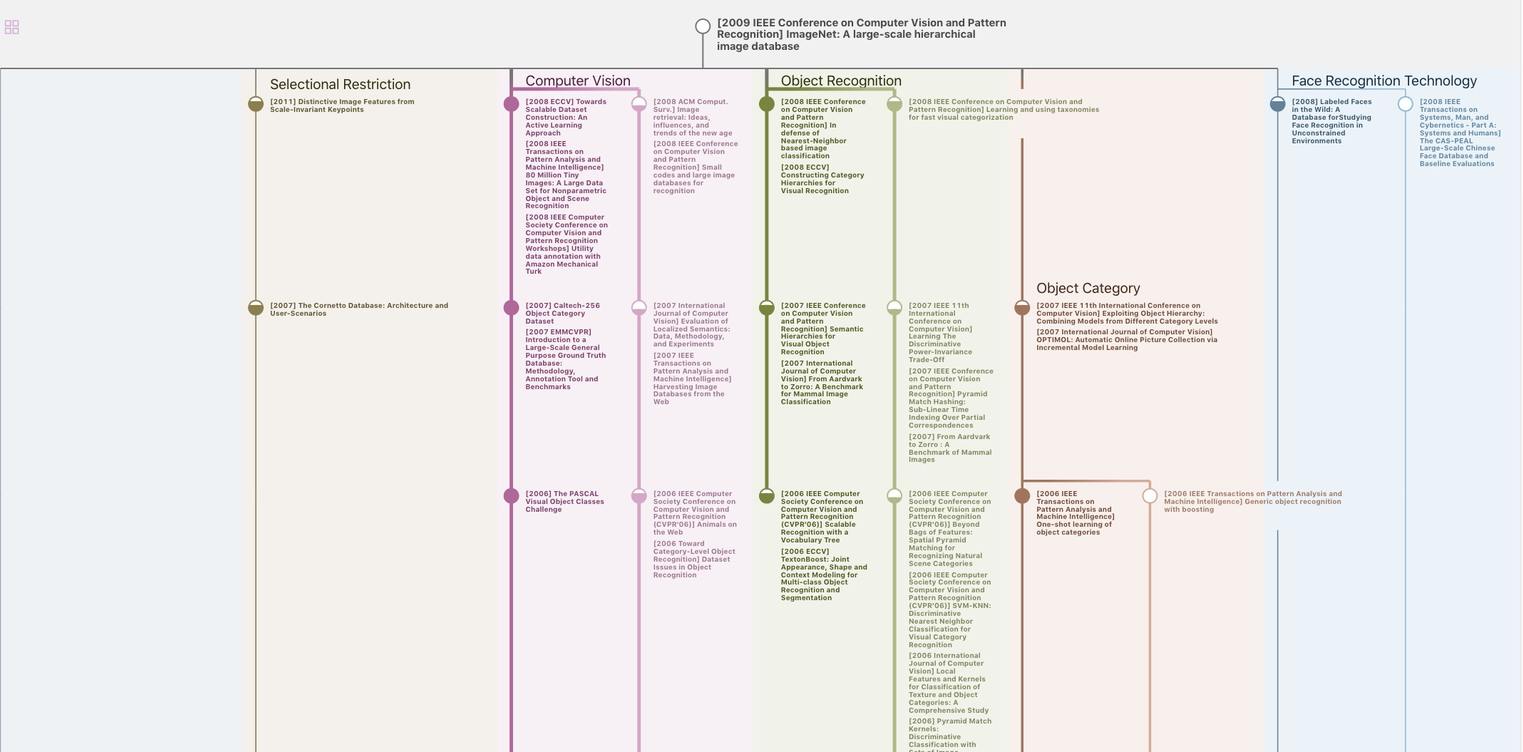
生成溯源树,研究论文发展脉络
Chat Paper
正在生成论文摘要