Toward Generative Adversarial Networks for the Industrial Internet of Things
IEEE Internet of Things Journal(2022)
摘要
Machine learning, as a viable way of conducting data analytics, has been successfully applied to a number of areas. Nonetheless, the lack of sufficient data is one critical issue for applying machine learning in Industrial Internet of Things (IIoT) systems. Insufficient data raises could negatively affect the accuracy of machine learning models. To tackle this issue, we design a framework to systematically investigate the impacts of insufficient data on model training. This framework employs the generative adversarial network (GAN) and continuous learning to generate and engage new data in model training, enabling us to study the security risks of introducing new data in the model training process and develop countermeasures to mitigate these risks. To validate the efficacy of our framework, we consider a representative IIoT scenario, in which a variety of industrial components needs to be recognized by convolutional neural networks (CNNs), and design and implement three evaluation scenarios that are based on a real-world IIoT data set. Our experimental results confirm that insufficient data can have a significant impact on the model accuracy, but that new data generated by GAN and continuous learning can greatly improve the model accuracy. Our experimental results also show that the data poisoning threat posed by the GAN can significantly reduce the model accuracy. However, our proposed defensive mechanism is capable of securing the model learning process. We conclude this article by discussing some emerging issues that need to be addressed in future work.
更多查看译文
关键词
Generative adversarial network (GAN),Industrial Internet of Things (IIoT),machine learning
AI 理解论文
溯源树
样例
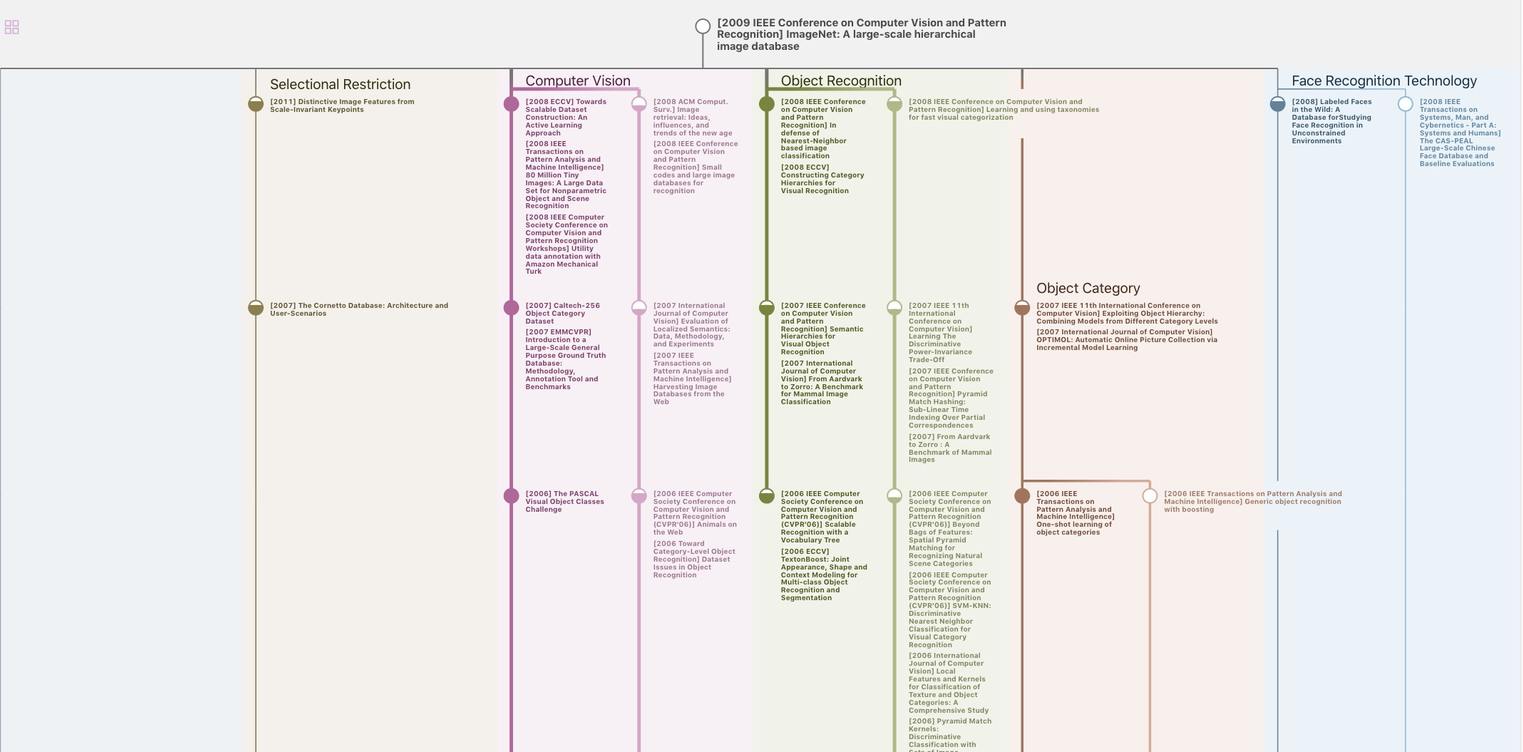
生成溯源树,研究论文发展脉络
Chat Paper
正在生成论文摘要