Joint Extraction of Long-Distance Entity Relation by Aggregating Local- and Semantic-Dependent Features
WIRELESS COMMUNICATIONS & MOBILE COMPUTING(2022)
摘要
The relations among long-distance entities are important for text understanding. Long-distance entities mean a pair of entities separated by more words in the text. Most current work of the joint extraction of entity relation is between entities that are close together. These methods are not suitable for long-distance entity relation extraction for they usually ignore the interaction between long-distance entities. In this paper, we propose Long-Distance Feature Aggregation Model (LDFAM), an end-to-end long-distance entity relation joint extraction model, which uses a Bi-GRU and weighted graph neural network to model text and learn its local- and semantic-dependent features. The long-distance dependence between entities is obtained by aggregating these two types of features. For entity recognition, we use conditional random fields to further obtain the dependency information between tags. For relation extraction, we fuse entity tag information for biaffine pairwise scoring. Experimental results on NYT and SemEval2010-Task8 datasets show that our model has achieved significant improvements compared to the baseline model. Finally, we apply the model to the field of material science to extract entity relationship knowledge from material literature. Experimental results show that benefiting from the long-distance entity relation extraction, the proposed method can get more useful knowledge from material literature and improve the knowledge coverage of material domain knowledge acquisition.
更多查看译文
AI 理解论文
溯源树
样例
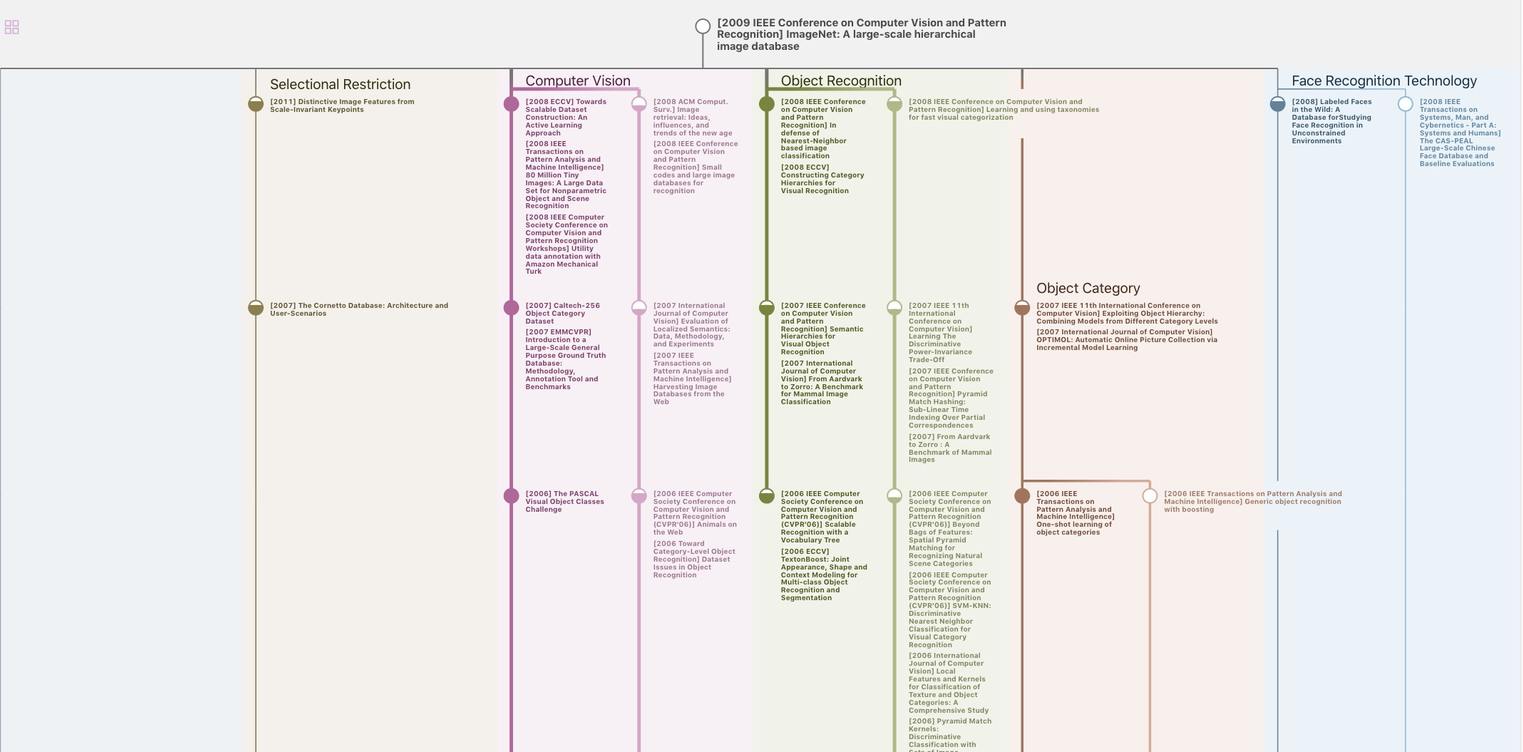
生成溯源树,研究论文发展脉络
Chat Paper
正在生成论文摘要