Predicting Stock Return Variance in a Large Cross Section
semanticscholar(2021)
摘要
The accuracy of variance prediction depends both on the validity of the specification and the accuracy of parameter estimation. Facing the unique challenges of predicting stock return variance in a large and varying universe, this paper proposes a conditional pooling approach that balances the need for reducing estimation errors while also allowing dynamics variation both cross-sectionally and over time. We start by specifying a cross-sectional forecasting relation at each date to better match the large and varying nature of the stock universe. We allow the relation to vary across names through conditionally weighted cross-sectional regressions. We show that the variance dynamics vary across different phases of a company’s information cycle and we use the historical variance term structure shape as conditioning variables to capture the phase differences in the information cycles of different names. Furthermore, we use the aggregate market variance term structure shape to capture the market condition and allow the forecasting dynamics to vary with market conditions via conditionally weighted averaging of historical coefficient estimates. The proposed estimation procedure reduces estimation errors via two-dimensional pooling, while also accommodates dynamics variation across both dimensions. A historical analysis of nearly a century worth of the US stock market data highlights the importance of two-dimensional pooling in reducing estimation errors, while also identifies large time-series and cross-sectional variations in the stock return variance dynamics. Our conditional pooling approach captures the benefits of both worlds, and greatly enhances the out-of-sample variance forecasting performance against standard benchmarks. JEL Classification: C13; C21; C51; C52; C53; C55
更多查看译文
AI 理解论文
溯源树
样例
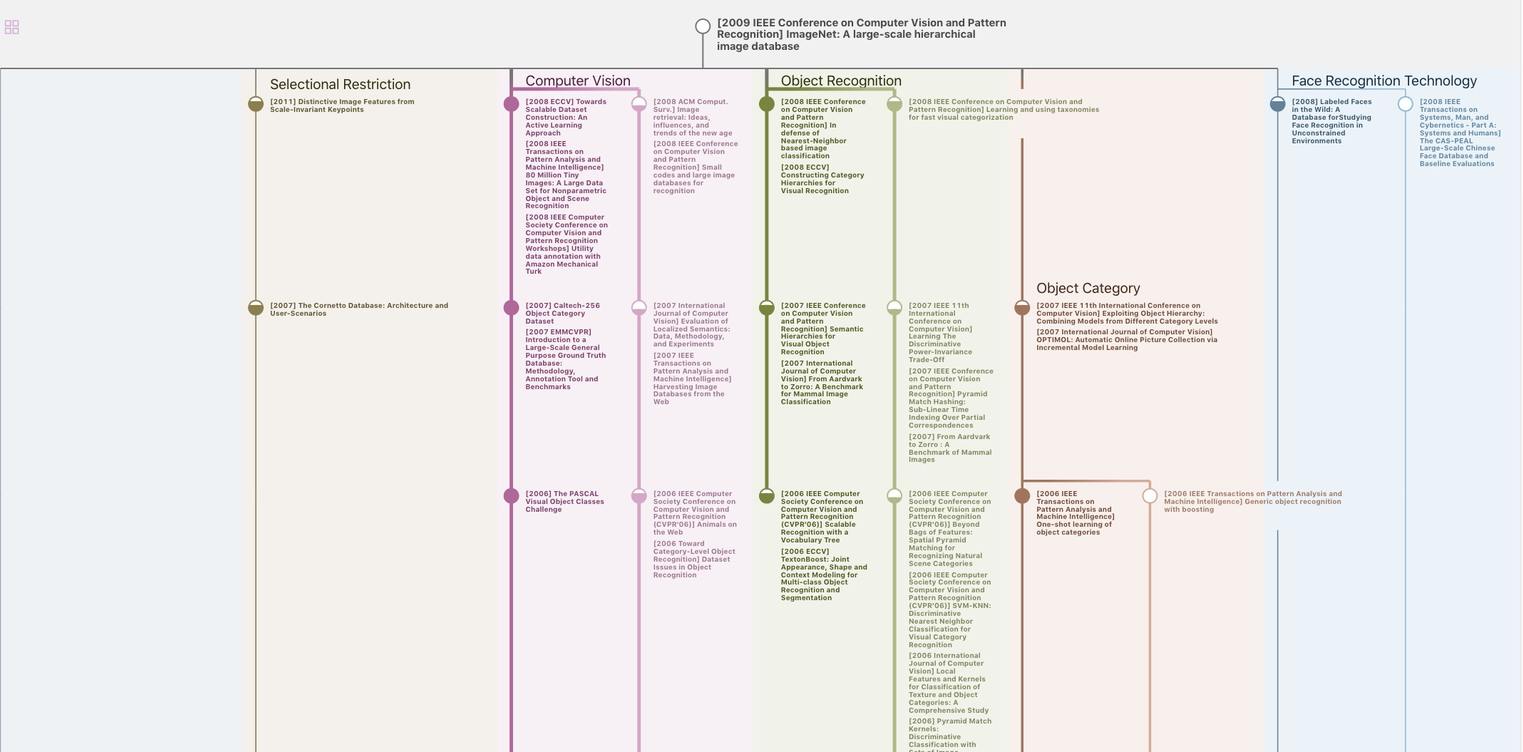
生成溯源树,研究论文发展脉络
Chat Paper
正在生成论文摘要