Cross-domain feature enhancement for unsupervised domain adaptation
Applied Intelligence(2022)
摘要
Till the present, the domain adaptation has been widely researched by transferring the knowledge from a labeled source domain to an unlabeled target domain. Adversarial adaptation methods have achieved great success, learning domain-invariant representations with category semantic information. Although domain-invariant representation is obtained, domain-specific variation is suppressed, which may distort the original feature distribution. In this paper, we propose a novel method called Cross-domain Feature Enhancement Domain Adaptation (CFEDA) which fills in the domain discrepancy to address the challenge of original domain feature information damage. Specifically, by leveraging the cross-domain and intra-domain prototype representations that are extracted through clustering, the features of both source and target domains can be enhanced. As a result, similar source domain and similar target domain features can be produced in the feature space to fill in the domain discrepancy. Since the target domain feature is unlabeled and can not be directly adopted for training, we exploit a feature consistency loss on it. Moreover, extensive experiments are conducted to demonstrate that CFEDA achieves significant performance improvements.
更多查看译文
关键词
Transfer learning, Domain adaptation, Image classification, Feature enhancement
AI 理解论文
溯源树
样例
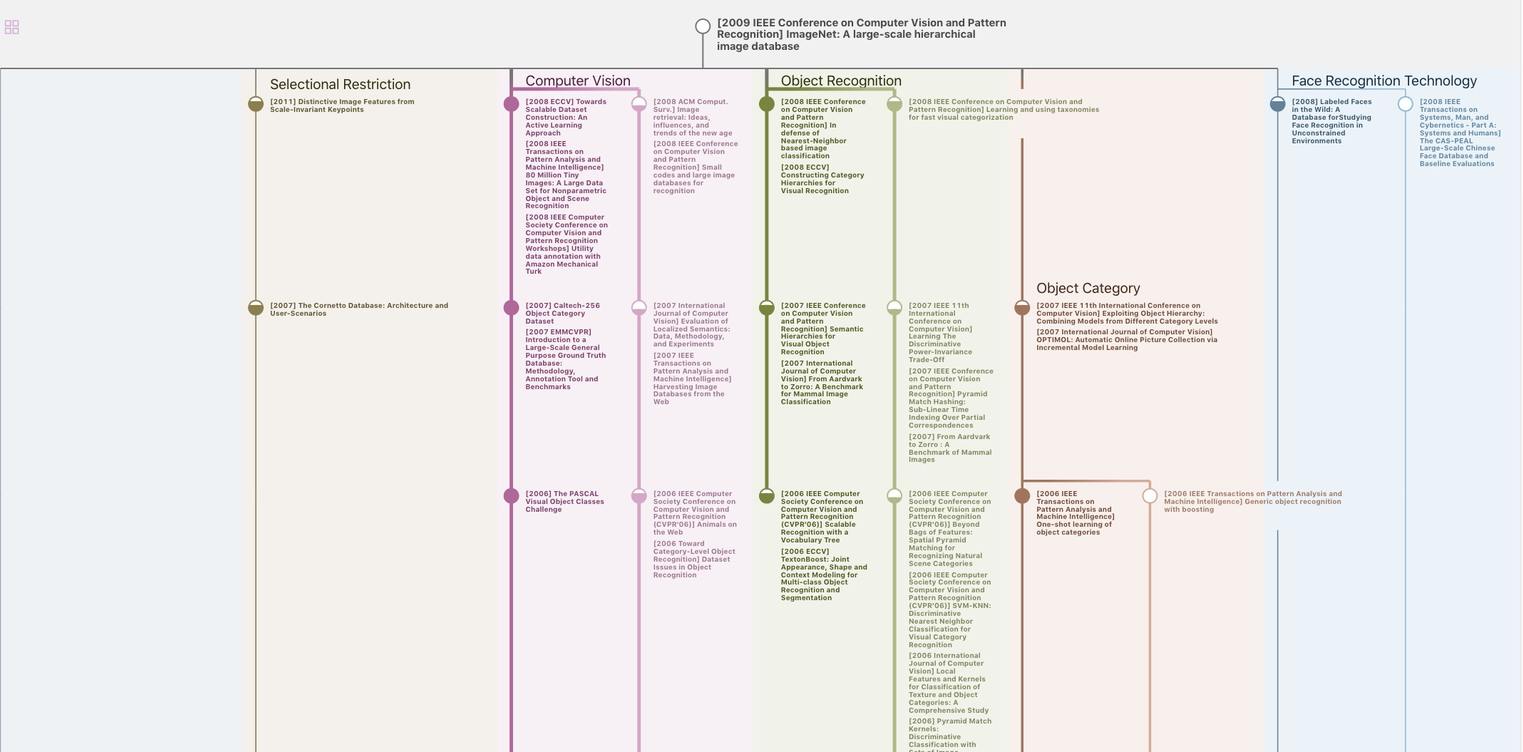
生成溯源树,研究论文发展脉络
Chat Paper
正在生成论文摘要