Bespoke data augmentation and network construction enable developmental morphological classification on limited microscopy datasets
bioRxiv(2022)
摘要
Deep learning is a powerful tool for image classification, yet training deep learning models often requires large datasets, which are not always available in biological research. Here we use a small dataset to train an accurate classifier by employing data augmentation regimes and Bayesian optimisation. We establish a new staging system for the Hamburger-Hamilton stage 10 chick embryo brain, based on morphology, identifying three sub-stages uncoupled from somite number, the usual metric used to stage chick embryos. Incorporating biologically informed, data-driven preprocessing, we train a deep convolutional neural network to classify our sub-stages. We find that augmenting our images with a combination of rotation, blur, shear, and cutout transformations is effective in training an accurate classifier on a small microscopy dataset. We then apply our approach to an unrelated small microscopy dataset comprising images of limb buds, finding good agreement with the results from brain classification. Through saliency analysis, we ensure that relevant features are being used by our classifier, gain insight into the efficacy of different data augmentations, and identify sub-stage specific features. In summary, we apply biological domain expertise to design effective data augmentation regimes for training a neural network-based image classifier on small, specialised datasets, classifying the developing brains and limbs with up to 90.9% and 94.4% accuracy respectively. These reliable classifiers can inform future experiments.
更多查看译文
AI 理解论文
溯源树
样例
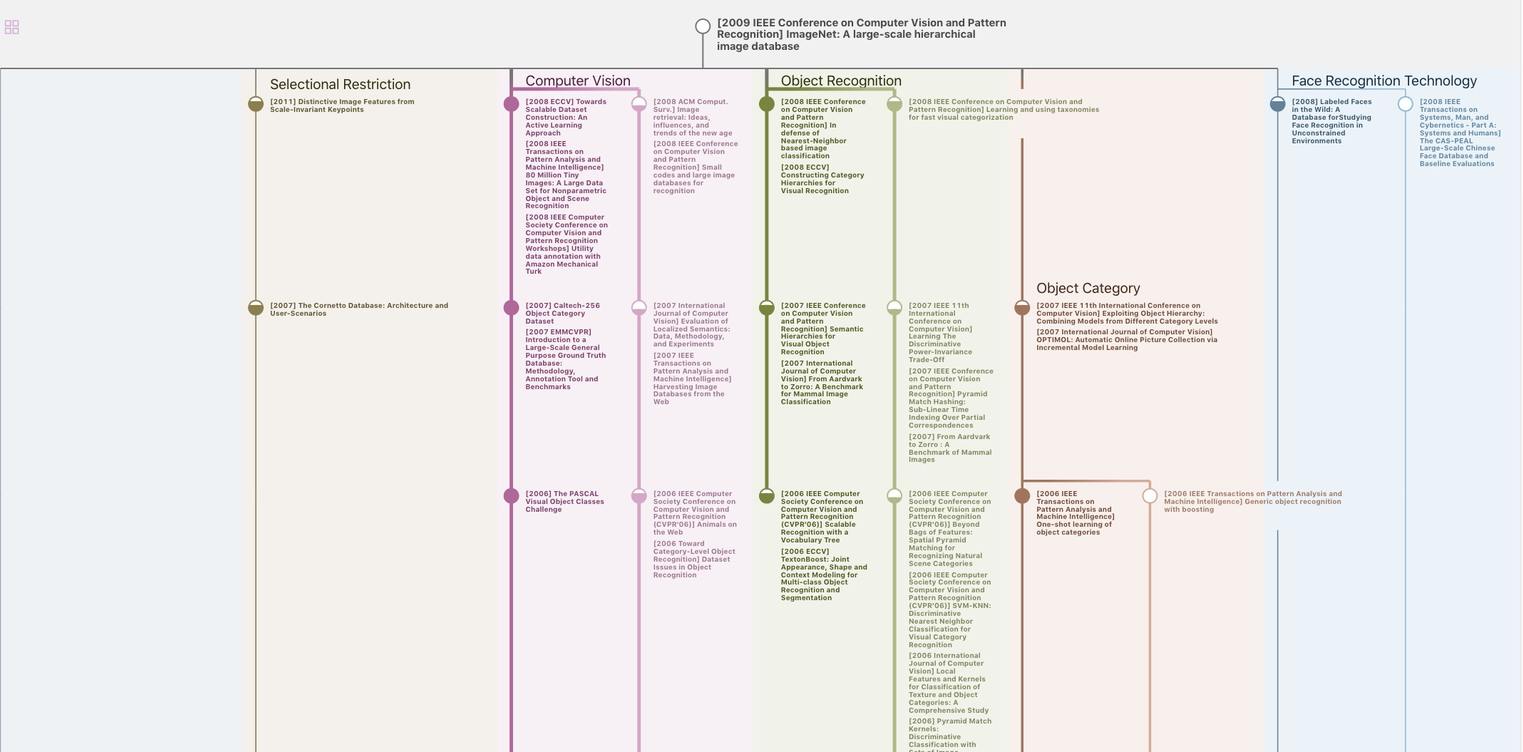
生成溯源树,研究论文发展脉络
Chat Paper
正在生成论文摘要