Can accurate demographic information about people who use prescription medications non-medically be derived from Twitter big data?
medRxiv(2022)
摘要
Traditional surveillance mechanisms for nonmedical prescription medication use (NPMU) involve substantial lags. Social media-based approaches have been proposed for conducting close-to-real-time surveillance, but such methods typically cannot provide fine-grained statistics about subpopulations. We address this gap by developing methods for automatically characterizing a large Twitter NPMU cohort (n=288,562) in terms of age-group, race, and gender. Our methods achieved 0.88 precision (95%-CI: 0.84-0.92) for age-group, 0.90 (95%-CI: 0.85-0.95) for race, and 0.94 accuracy (95%-CI: 0.92-0.97) for gender. We compared the automatically-derived statistics for the NPMU of tranquilizers, stimulants, and opioids from Twitter to statistics reported in traditional sources (eg., the National Survey on Drug Use and Health). Our estimates were mostly consistent with the traditional sources, except for age-group-related statistics, likely caused by differences in reporting tendencies and representations in the population. Our study demonstrates that subpopulation-specific estimates about NPMU may be automatically derived from Twitter to obtain early insights.
更多查看译文
关键词
prescription medications,demographic information,twitter,non-medically
AI 理解论文
溯源树
样例
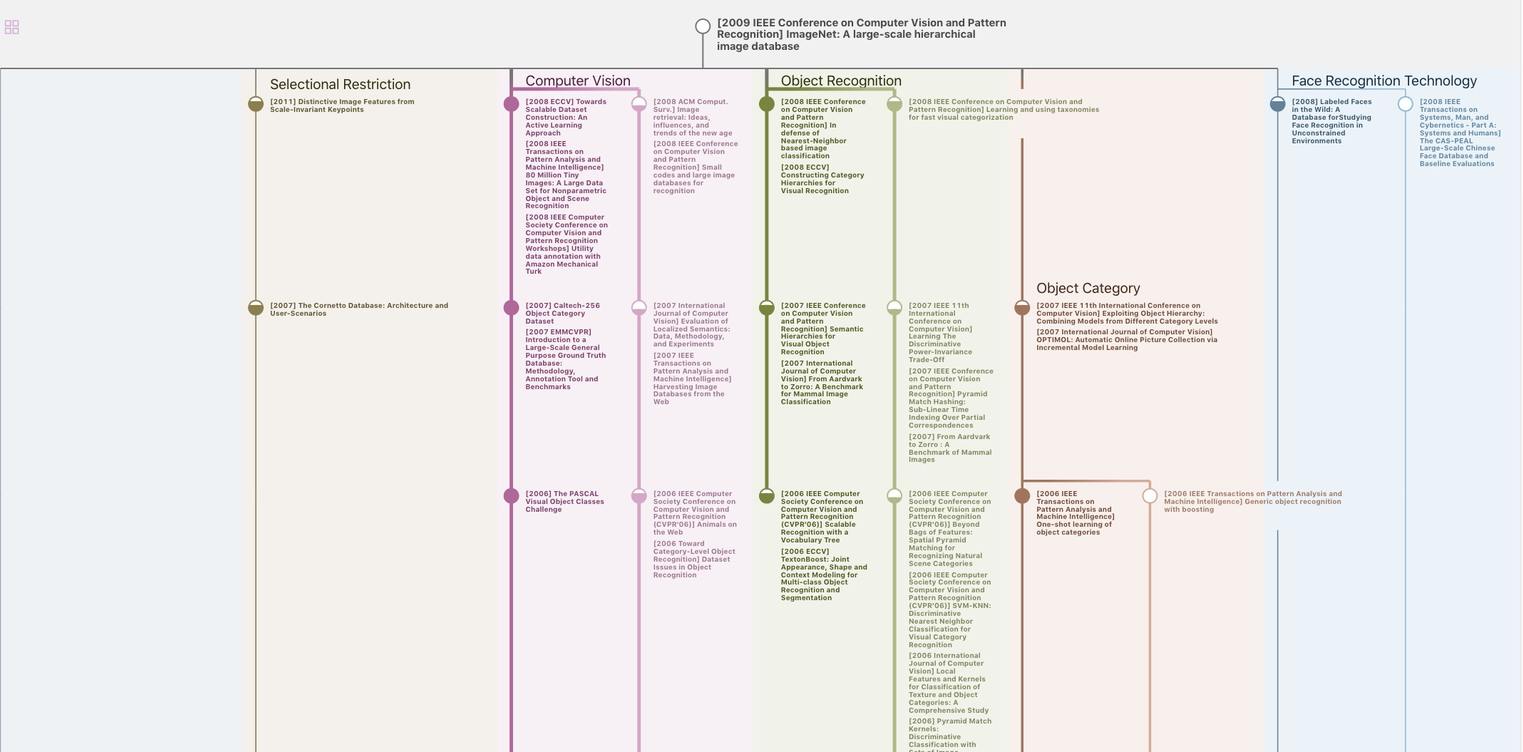
生成溯源树,研究论文发展脉络
Chat Paper
正在生成论文摘要