Error Fields: Robotic training forces that forgive occasional movement mistakes
semanticscholar(2022)
摘要
Control of movement uses error feedback during practice to predict actions for the next movement. We have shown that augmenting error can enhance motor learning, while such findings are encouraging, new methods are needed to accommodate a person's individual reactions to error. The current study demonstrates the design we call error fields (EF), where we temper the augmentation when errors are less likely. We tested the ability of healthy participants (n=21) to adapt to a visual transformation, and we enhanced the training with error fields. We found that training with error fields led to the fastest learning and greatest reduction in error. EF training reduced error more and faster than controls who practiced without error fields (50% more and 46% faster in the target direction; 21% more and 67% faster in the direction perpendicular to that). Moreover, EF was also significantly greater and faster than our previous error augmentation (EA) technique. Hence, clear advantages exist no mater how this is measured or compared to controls. These findings represent an effective teaching method for enhanced training that leverages the statistics of error.
更多查看译文
关键词
robotic training forces,occasional movement mistakes
AI 理解论文
溯源树
样例
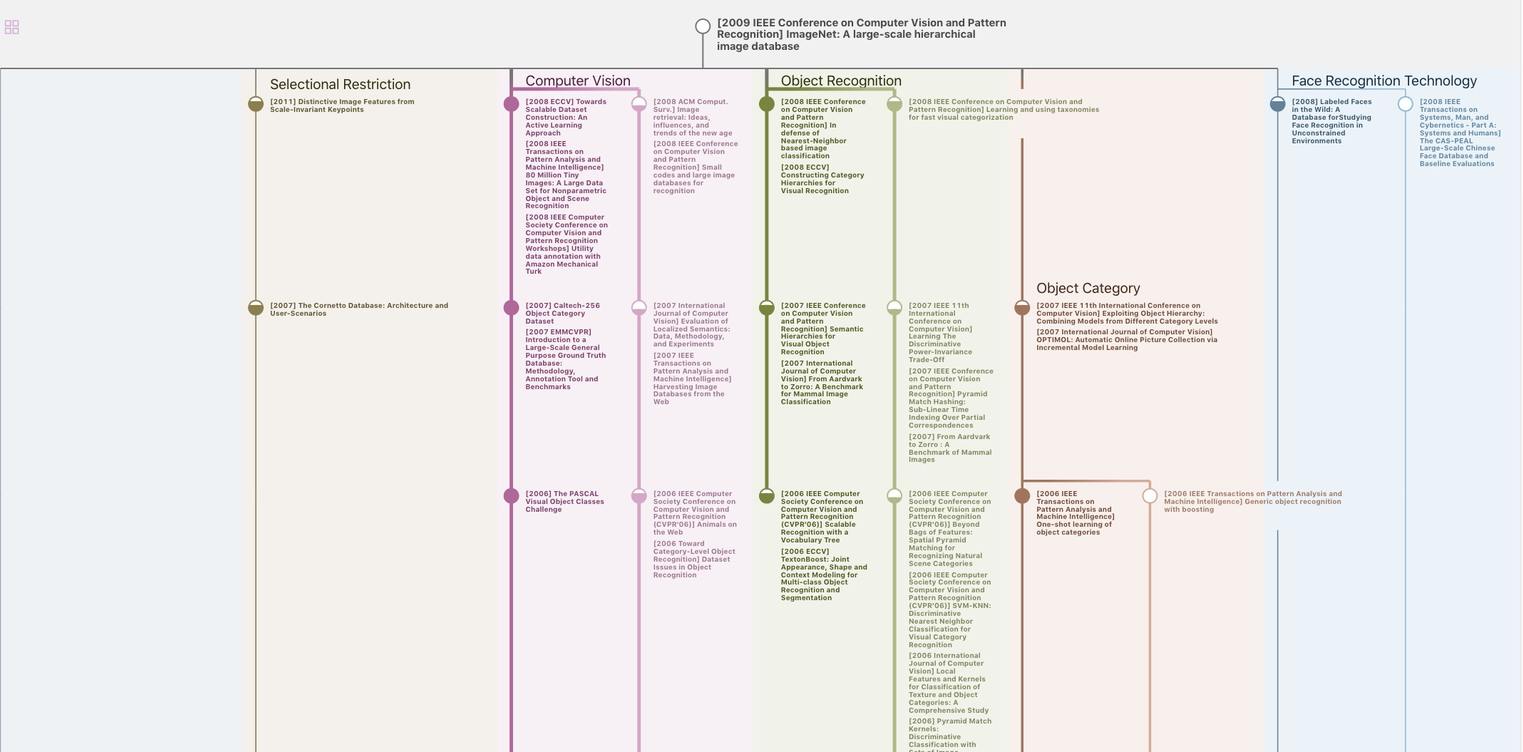
生成溯源树,研究论文发展脉络
Chat Paper
正在生成论文摘要