Autodist: a composable and automated synchronization system for distributed deep learning
semanticscholar(2021)
摘要
Efficient data-parallel distributed training has been a key driver behind recent innovations in deep learning (DL). However, achieving satisfactory distributed performance involves making difficult system-level decisions related to diverse synchronization aspects. We present AutoDist, which automatically composes parallel synchronization strategies for DL models by rewriting their original dataflow graphs into parallel versions. Unlike existing training systems with fixed strategies, AutoDist adaptively composes strategies by jointly optimizing multiple aspects, each applied to different parts of the DL model. Compared to other graph rewriting systems, AutoDist deliberately breaks seemingly distinct synchronization optimizations into atomic graph rewriting kernels, and allows mechanically assembling them to express new strategies that extrapolate to new models and clusters. We show that AutoDist can find high-performance strategies quickly, and enables model training 1.2x to 1.6x faster than hand-optimized baselines. Critically, AutoDist does not require manual tuning when faced with new DL models or cluster configurations.
更多查看译文
AI 理解论文
溯源树
样例
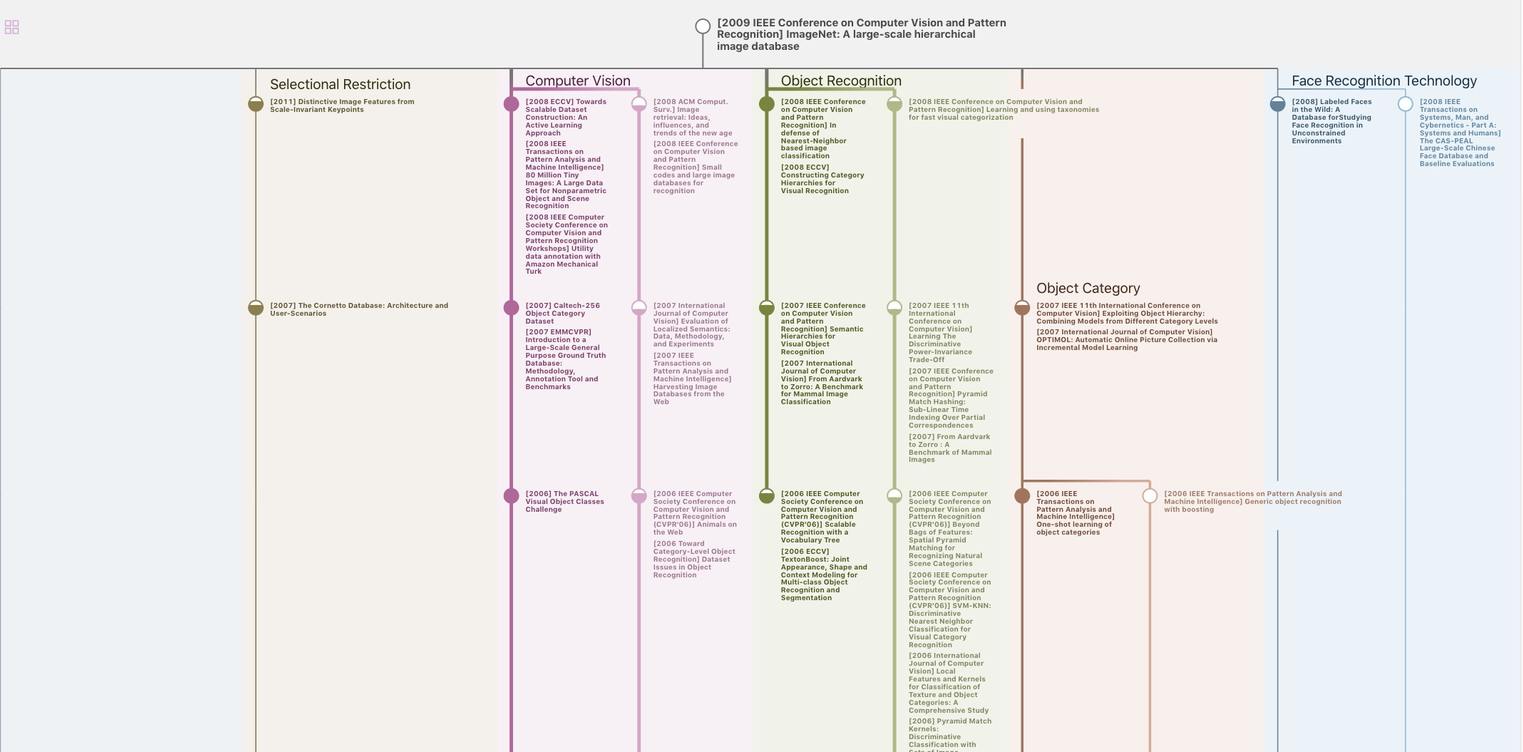
生成溯源树,研究论文发展脉络
Chat Paper
正在生成论文摘要