Ensemble Policy Distillation in Deep Reinforcement Learning
semanticscholar(2020)
摘要
Policy distillation in deep reinforcement learning transfers the knowledge learned by a large teacher model to a compact student model, which reduces the inference time and power consumption. However, the compression ratio and the long training time are not always satisfactory. One promising approach is for the teacher’s training and student’s distillation to occur simultaneously, so that the latest learned policy is distilled in real time. However, an intrinsic problem arises when the teacher provides unstable supervision, as this may misdirect the distillation process and lead to failure. Until now, only few research works have addressed the problem of instability and distillation performance. In this work, we propose a policy distillation mechanism that applies ensemble distillation in a new way, which makes more high-quality and reliable supervisions available for the student to realize full distillation. In addition, the validity of ensemble distillation has been demonstrated for the improvement of generalization, which enhances the student model’s robustness. We verify our algorithm in the OpenAI Atari game domain. The results show that the proposed approach achieves nearly full distillation and even greater performance on some tasks.
更多查看译文
AI 理解论文
溯源树
样例
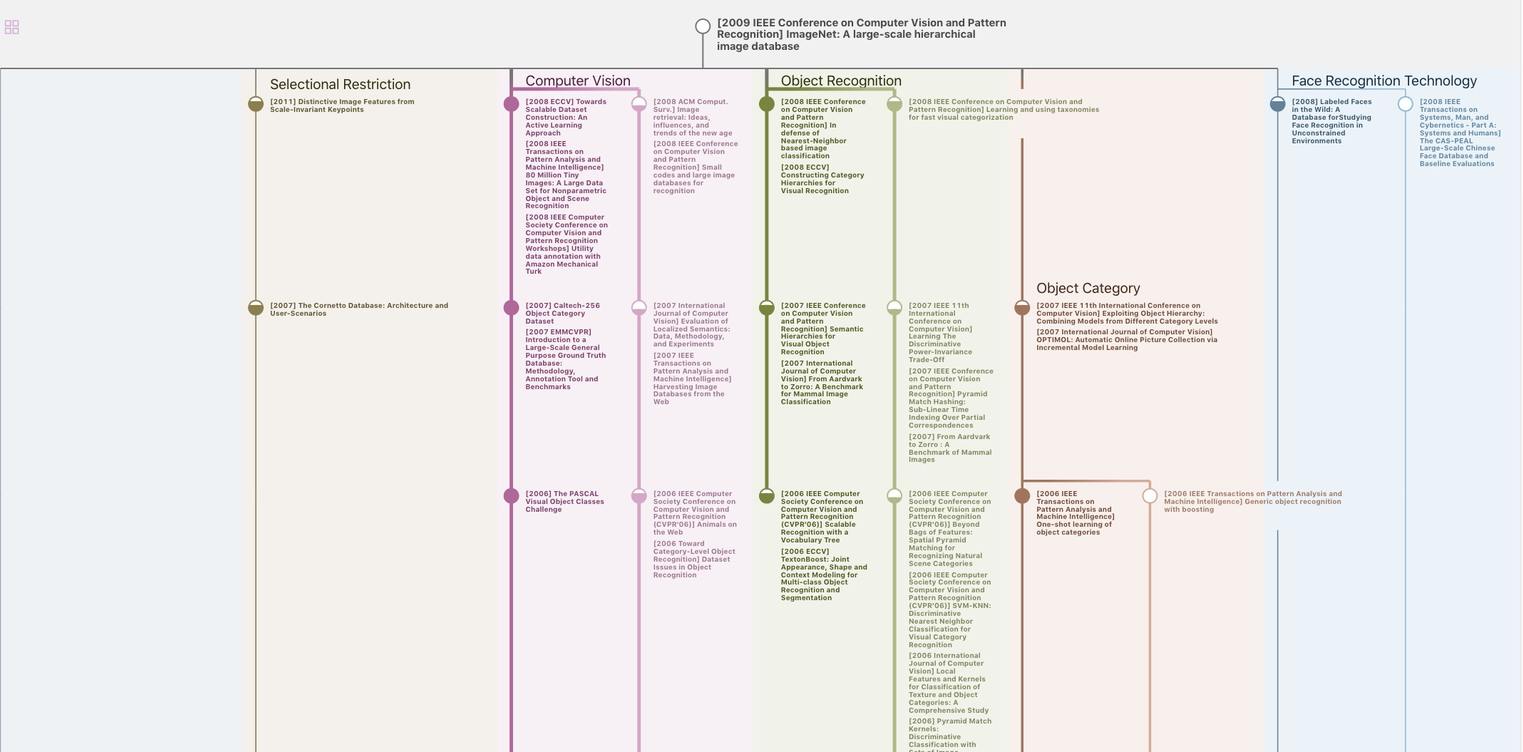
生成溯源树,研究论文发展脉络
Chat Paper
正在生成论文摘要