A multi-level feature integration network for image inpainting
MULTIMEDIA TOOLS AND APPLICATIONS(2022)
摘要
Deep learning-based methods have shown great potential in image inpainting, especially when dealing with large missing regions. However, the inpainted results often suffer from blurring, and improper textures can be created without an understanding of semantic information. In order to extract more features from the known regions, we propose a multi-level feature integration (MFI) network for image inpainting. We complete hole regions by two generators. For each generator, we use the MFI network to fill the hole region with multi-level skip connections. With multi-level feature integration, the network gains more knowledge about the global semantic structures and local fine details. Moreover, instead of a deconvolution layer or an interpolation algorithm, we adopt a sub-pixel layer to up-sample feature maps and produce more coherent results. We use PatchGAN to support the refinement generator network to produce more discriminative detail. Our experiments done with the Paris StreetView, CelebA-HQ and Places2 datasets demonstrate the effectiveness of our MFI network for producing visually pleasing results with semantically ordered textures.
更多查看译文
关键词
Image inpainting,Multi-level,Feature integration,Skip connection
AI 理解论文
溯源树
样例
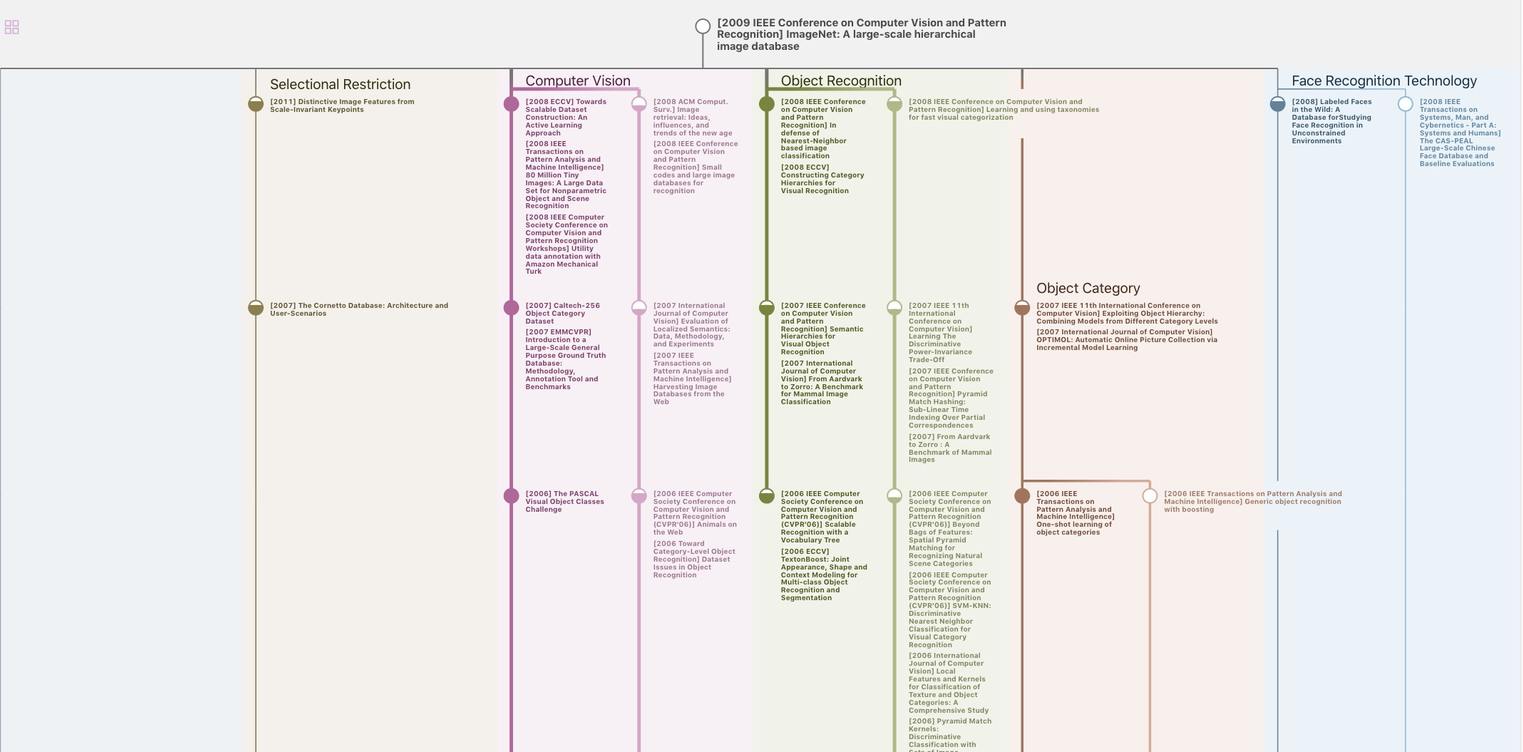
生成溯源树,研究论文发展脉络
Chat Paper
正在生成论文摘要