Adaptive e-learning recommendation model based on the knowledge level and learning style
semanticscholar(2021)
摘要
E-learning systems facilitate the process of education and interaction between teachers and learners during minimizing a lot of temporal or spatial restrictions. Recently, many learners prefer to use electronic devices to achieve everyday jobs. The process of using the learner style, learning goals, and learner characteristics, and electronic devices and systems are called Adaptive e-learning. The importance of Adaptive E-learning Systems (AES) is to help the teachers to choose and recommend some materials to the learner and to increase the knowledge level. This paper develops a new adaptive e-learning recommender model using learning style and Knowledge Level Modeling (AERM-KLLS). The proposed model adapted automatically to the requirements, interests, and levels of knowledge of the learners by analyzing learner style using a fast questionnaire, representing the knowledge level and all the course questions in the learner model as a knowledge overlay model, which helps the proposed model to recommend objects from the points that have the least scores at the pre-test. The analysis of AERM-KLLS performance measured by making two tests and comparing the two test performance; the performance of using the AERM-KLLS algorithm used in the second test for 321 participated learners increases the overall performance for the students with an accuracy of 90.97%. The proposed model provided to the learners for an English course in the Faculty of Computer Science, October 6University, to make the best use of the E-learning advantage and increasing the performance, in COVID 19 restrictions.
更多查看译文
AI 理解论文
溯源树
样例
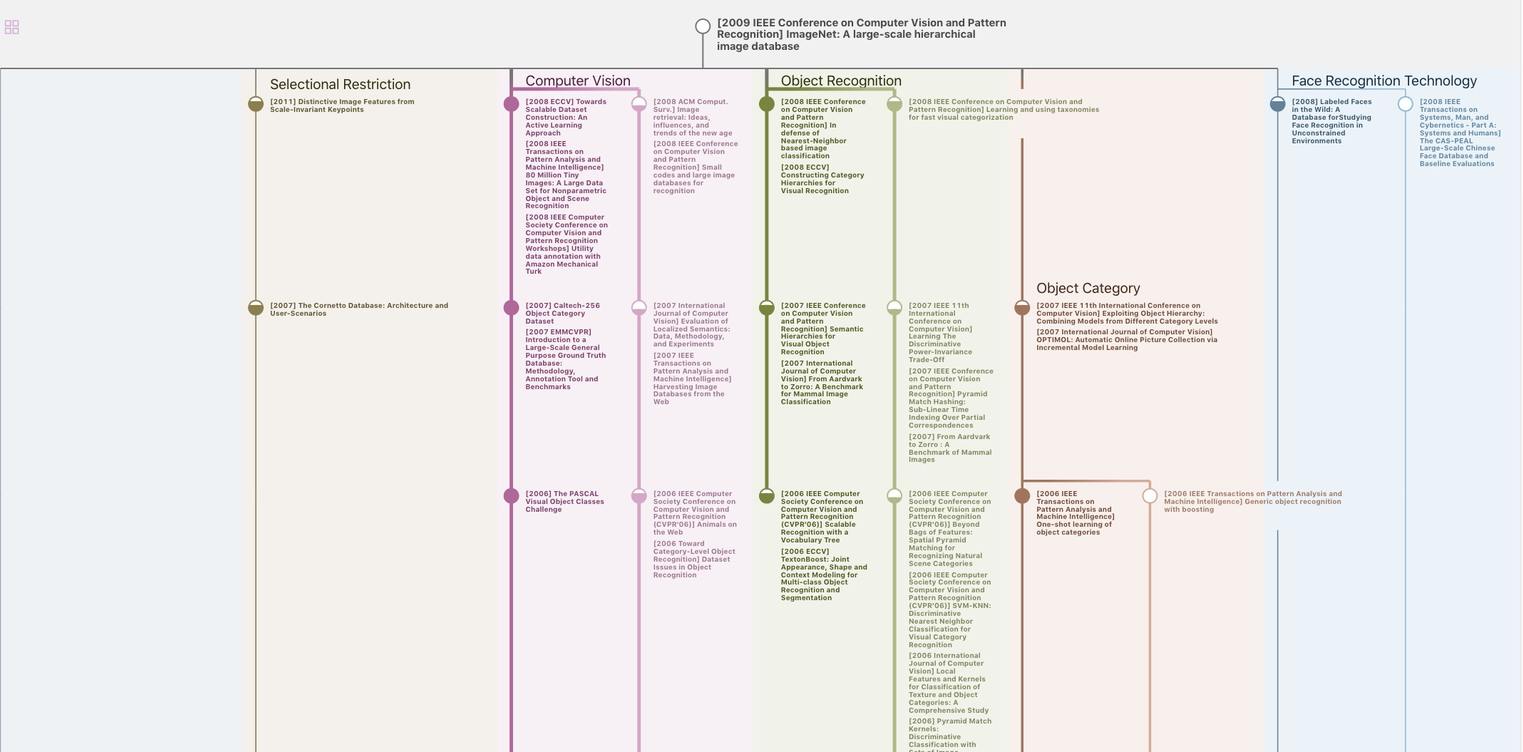
生成溯源树,研究论文发展脉络
Chat Paper
正在生成论文摘要