TG-OUT: temporal outlier patterns detection in Twitter attribute induced graphs
WORLD WIDE WEB-INTERNET AND WEB INFORMATION SYSTEMS(2022)
摘要
Given a network of Twitter users, can we capture their posting behavior over time, identify patterns that could probably describe, model or predict their activity? Can we identify temporal connectivity patterns that emerge from the use of specific attributes? More challengingly, are there particular attribute usage patterns which indicate an inherent anomaly? This work provides solid answers to all these questions, extending previous work employed on other social networks and attribute types. We propose TG-OUT , a pipeline of methods which : (a) model the temporal evolution of attribute induced graphs to detect peculiar attributes, (b) identify temporal patterns in attribute distributions, (c) investigate differences in patterns emerging from bot and/or non-bot accounts, (d) extract tailored sets of exploitable features. Experimental results show that: most of the individual attribute distributions remain stable over time following mostly power laws norm; the temporal evolution of attribute induced graphs obey certain laws and deviations are outliers; we discover that patterns present deviations which depend on the type of accounts which use each attribute; finally, we show that careful selection of only two features which are used to train a simple machine learning algorithm, produces a model which efficiently identifies attributes mainly used by bots.
更多查看译文
关键词
Graph theory,Social networks,Twitter,Anomaly detection,Temporal graphs,Node attributed graphs,Bot detection
AI 理解论文
溯源树
样例
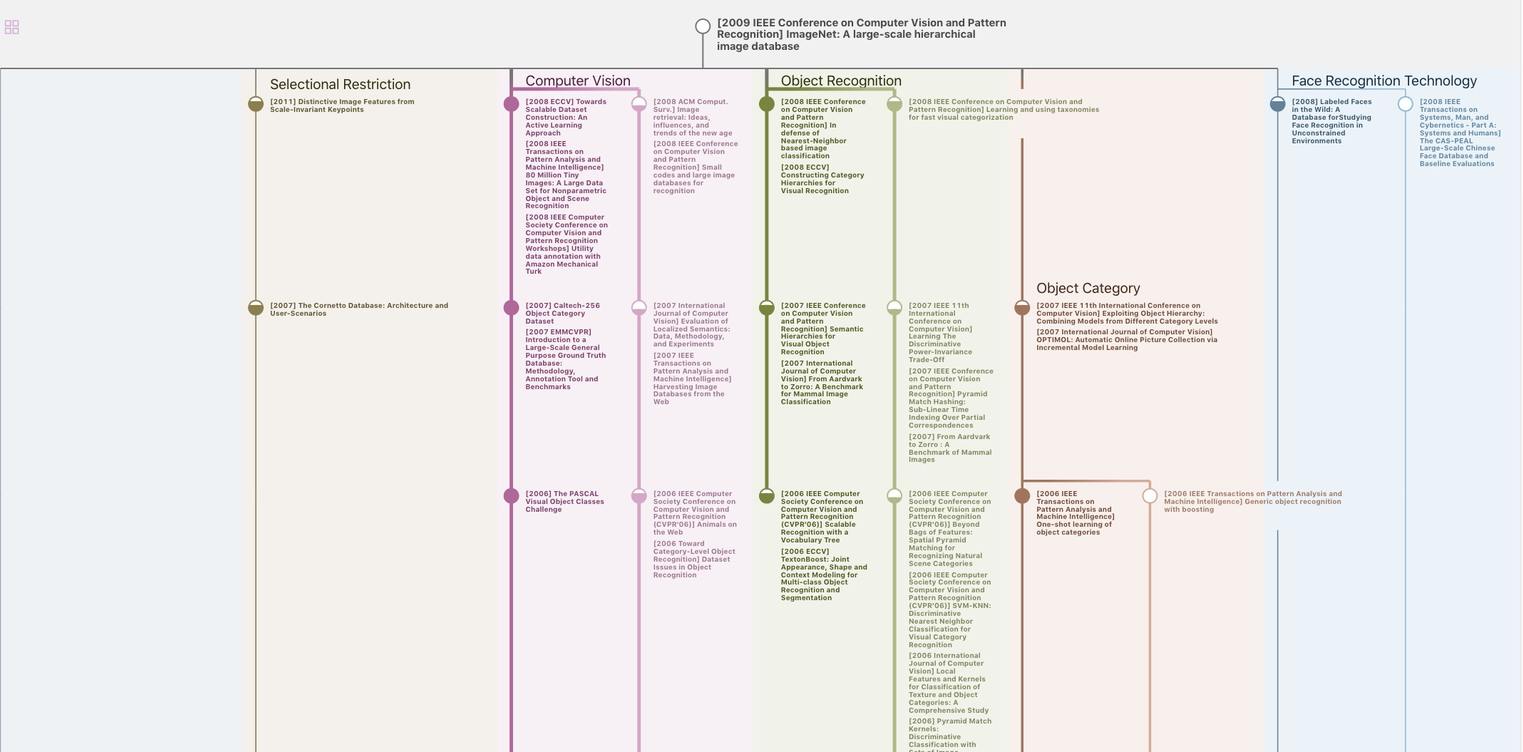
生成溯源树,研究论文发展脉络
Chat Paper
正在生成论文摘要