Fair automated assessment of noncompliance in cargo ship networks
EPJ Data Science(2022)
摘要
Cargo ships navigating global waters are required to be sufficiently safe and compliant with international treaties. Governmental inspectorates currently assess in a rule-based manner whether a ship is potentially noncompliant and thus needs inspection. One of the dominant ship characteristics in this assessment is the ‘colour’ of the flag a ship is flying, where countries with a positive reputation have a so-called ‘white flag’. The colour of a flag may disproportionately influence the inspector, causing more frequent and stricter inspections of ships flying a non-white flag, resulting in confirmation bias in historical inspection data. In this paper, we propose an automated approach for the assessment of ship noncompliance, realising two important contributions. First, we reduce confirmation bias by using fair classifiers that decorrelate the flag from the risk classification returned by the model. Second, we extract mobility patterns from a cargo ship network, allowing us to derive meaningful features for ship classification. Crucially, these features model the behaviour of a ship, rather than its static properties. Our approach shows both a higher overall prediction performance and improved fairness with respect to the flag. Ultimately, this work enables inspectorates to better target noncompliant ships, thereby improving overall maritime safety and environmental protection.
更多查看译文
关键词
Data-driven inspection,Fair classification,Mobility patterns,Port state control,Cargo ship network,Ship risk profile,Preventing confirmation bias
AI 理解论文
溯源树
样例
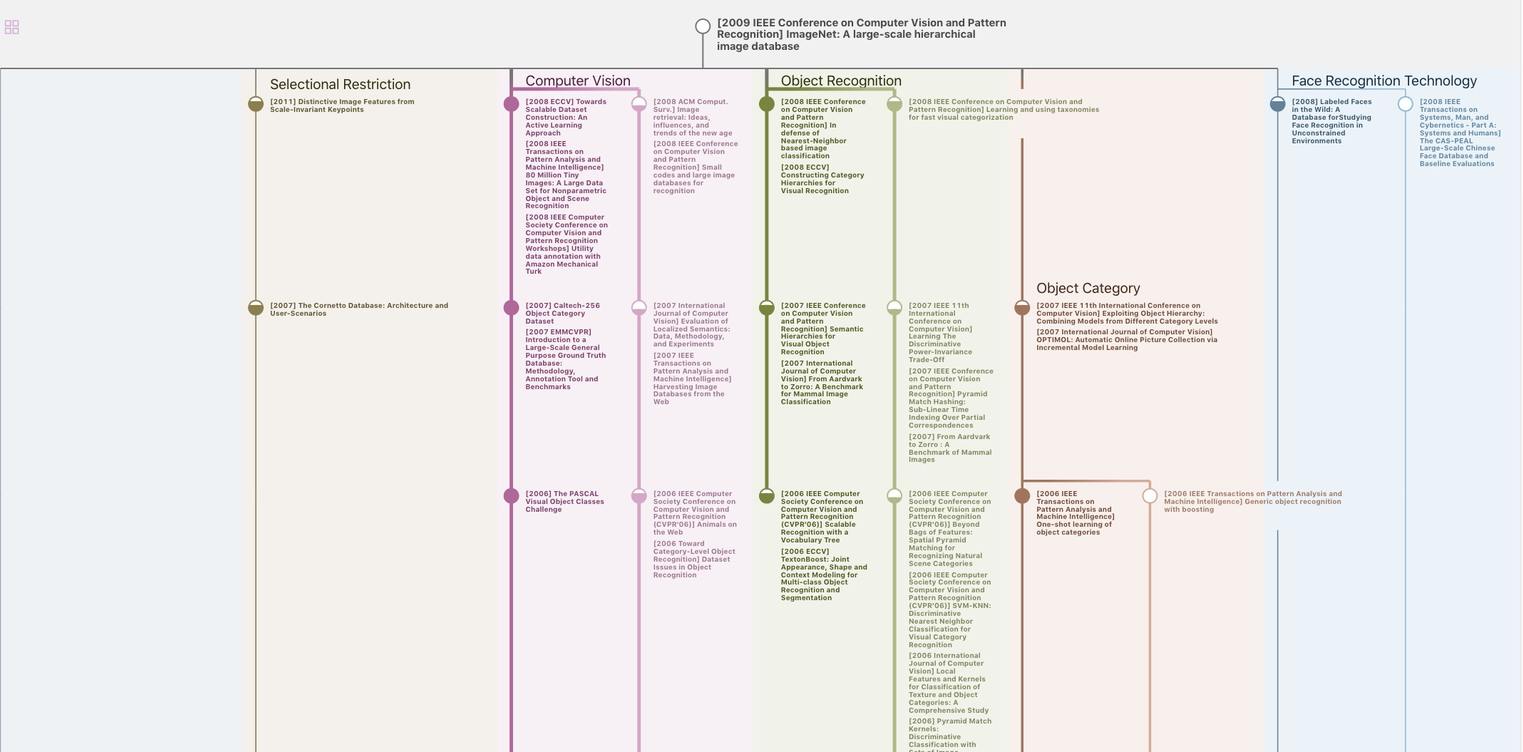
生成溯源树,研究论文发展脉络
Chat Paper
正在生成论文摘要