A 65nm 73Kb SRAM-Based Computing-In-Memory Macro with Dynamic-Sparsity Controlling
IEEE Transactions on Circuits and Systems Ii-express Briefs(2022)
摘要
For neural network (NN) applications at the edge of AI, computing-in-memory (CIM) demonstrates promising energy efficiency. However, when the network size grows while fulfilling the accuracy requirements of increasingly complicated application scenarios, significant memory consumption becsomes an issue. Model pruning is a typical compression approach for solving this problem, but it does not fully exploit the energy efficiency advantage of conventional CIMs, because of the dynamic distribution of sparse weights and the increased data movement energy consumption of reading sparsity indexes from outside the chip. Therefore, we propose a vector-wise dynamic-sparsity controlling and computing in-memory structure (DS-CIM) that accomplishes both sparsity control and computation of weights in SRAM, to improve the energy efficiency of the vector-wise sparse pruning model. Implemented in a 65 nm CMOS process, the measurement results show that the proposed DS-CIM macro can save up to 50.4% of computational energy consumption, while ensuring the accuracy of vector-wise pruning models. The test chip can also achieve 87.88% accuracy on the CIFAR-10 dataset at 4-bit precision in inputs and weights, and it achieves 530.2TOPS/W (normalized to 1 bit) energy efficiency.
更多查看译文
关键词
SRAM,computing-in-memory (CIM),regular pruning,dynamic sparsity,energy efficiency
AI 理解论文
溯源树
样例
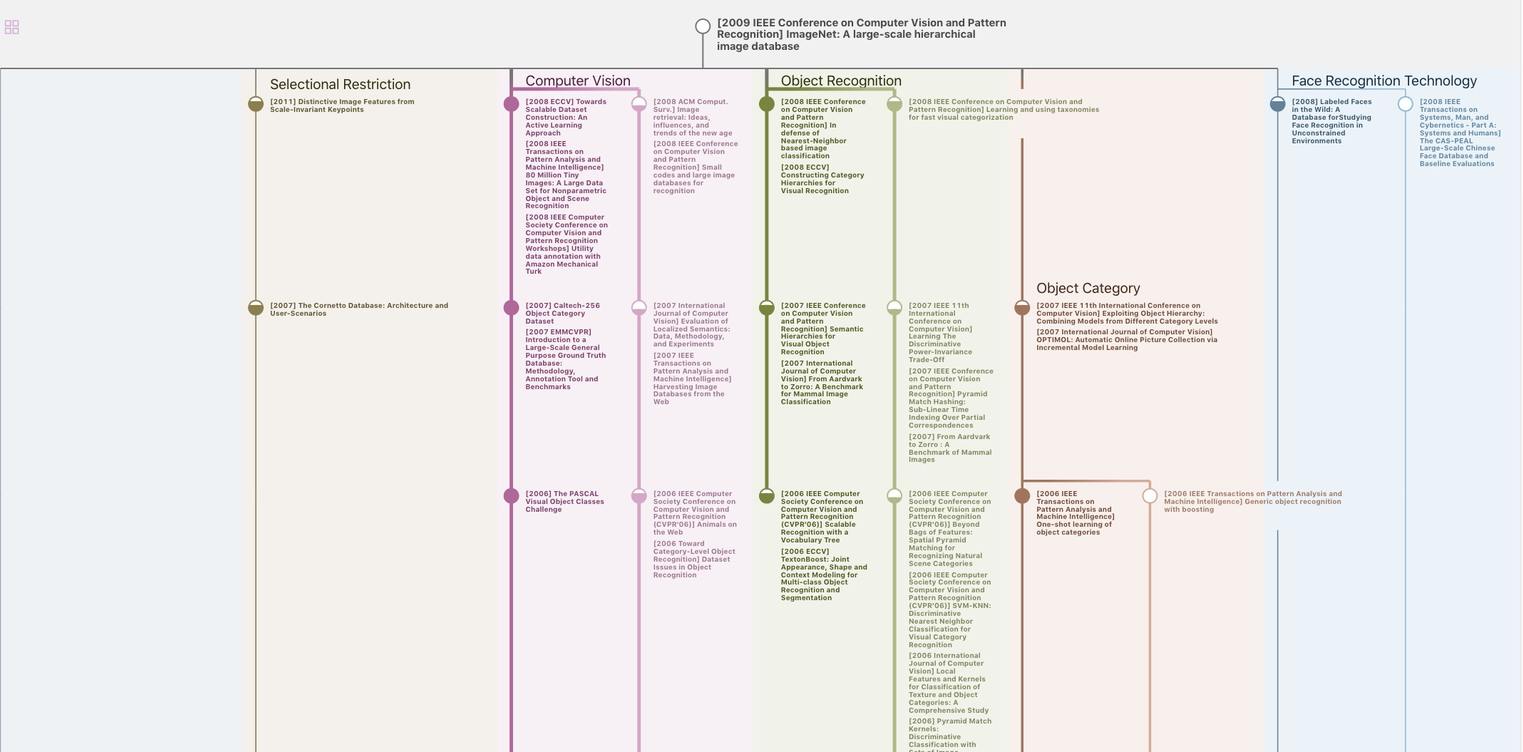
生成溯源树,研究论文发展脉络
Chat Paper
正在生成论文摘要