Broad and deep neural network for high-dimensional data representation learning
Information Sciences(2022)
摘要
Limited by the shallow structure, broad learning system (BLS) suffers from the high-dimensional data classification difficulty. To this end, the cascade of the convolutional feature mappings and enhancement mappings broad learning system (CCFEBLS) framework is proposed from the perspective of representation learning in this article. Firstly, convolution kernels are exploited to construct the convolutional feature nodes and enhancement nodes instead of using sparse auto-enocder or linear combination in the BLS. Secondly, we design a novel broad and deep architecture which cascades the feature mappings and enhancement mappings as the broad and deep representations to connect the output directly for the CCFEBLS framework. This architecture utilizes all representations thoroughly and improves the representation learning capability. Moreover, to boost the robustness of the CCFEBLS, the weighted hyper-parameters and the group regularization are developed to adjust the broad and deep representations and require the group output directly approximate the label, respectively. And the experimental results on several synthetic and real world datasets have demonstrated that CCFEBLS models outperform the baselines with better performance, less parameters and training time, which are validated to be consistent with the model design and analysis.
更多查看译文
关键词
Broading learning system,Broad and deep architecture,Representation learning,High-dimensional data
AI 理解论文
溯源树
样例
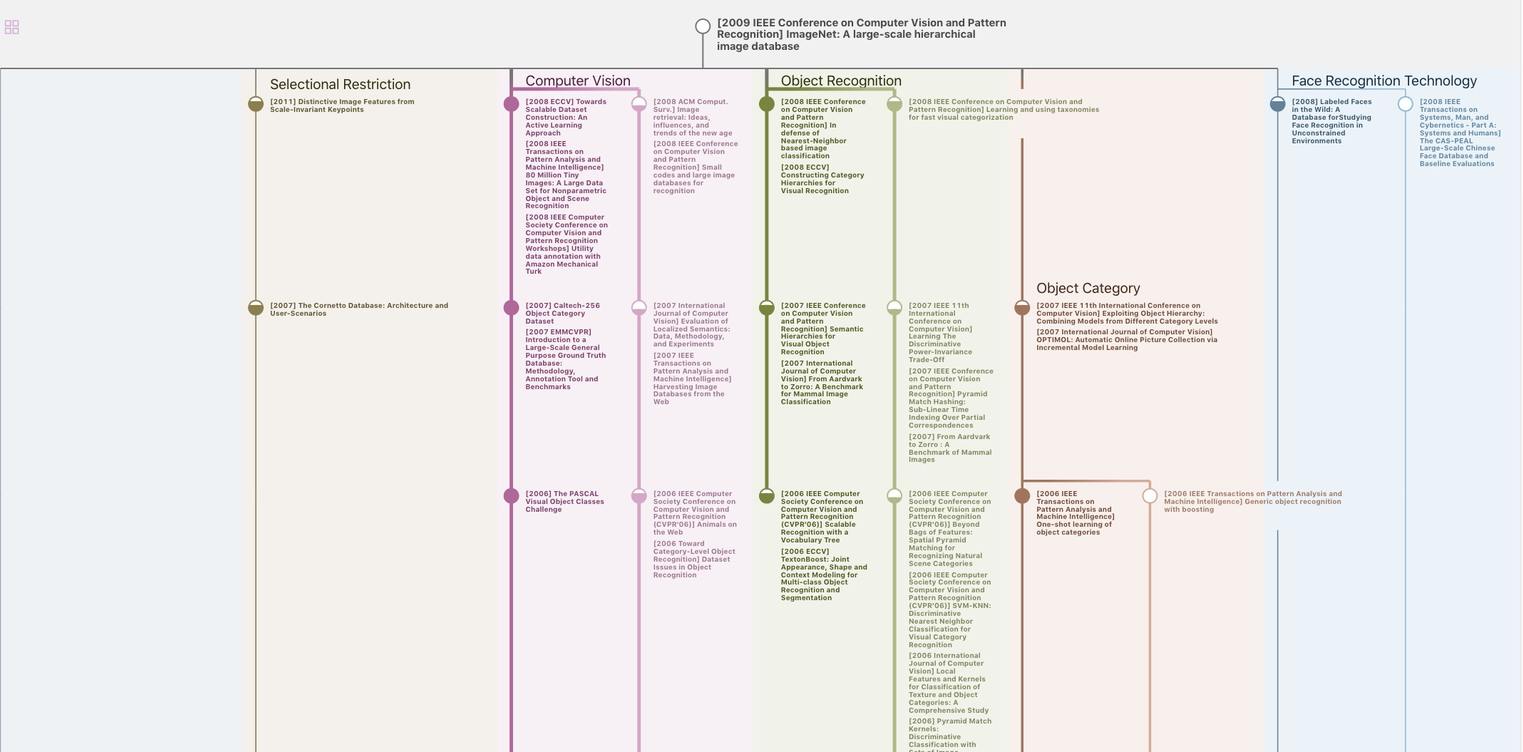
生成溯源树,研究论文发展脉络
Chat Paper
正在生成论文摘要