CPDGA: Change point driven growing auto-encoder for lifelong anomaly detection
Knowledge-Based Systems(2022)
摘要
Lifelong learning addresses the challenge of acquiring new knowledge and tackling new tasks in a continually evolving environment. Although this thread of research has recently received increased interest, most lifelong machine learning approaches proposed thus far focus on object recognition or classification tasks. In contrast, lifelong approaches for anomaly detection are still unexplored. This paper presents a method for lifelong anomaly detection loosely based on biological principles, which can adapt to the environment and efficiently recall old information from its memory bank. Inspired by the interaction between the cortex and the hippocampus in biology, we combine deep learning with statistical change point detection. Our method induces concepts from its environment and organizes them in a semantically coherent forest structure in an unsupervised manner. At runtime, we analyze new objects, one by one, with respect to the current forest of concepts. If a new object fits an existing concept, it is added to the pool of objects representing that concept. Otherwise, it is further analyzed to determine whether it represents a new concept, a new sub-concept, or it is an anomaly. Experiments conducted over different applied settings show that the synergic interaction of change point detection with an evolving forest of concepts yields a higher anomaly detection performance than state-of-the-art methods.
更多查看译文
关键词
Anomaly detection,Lifelong learning,Auto-encoders,Neural networks,Unsupervised learning
AI 理解论文
溯源树
样例
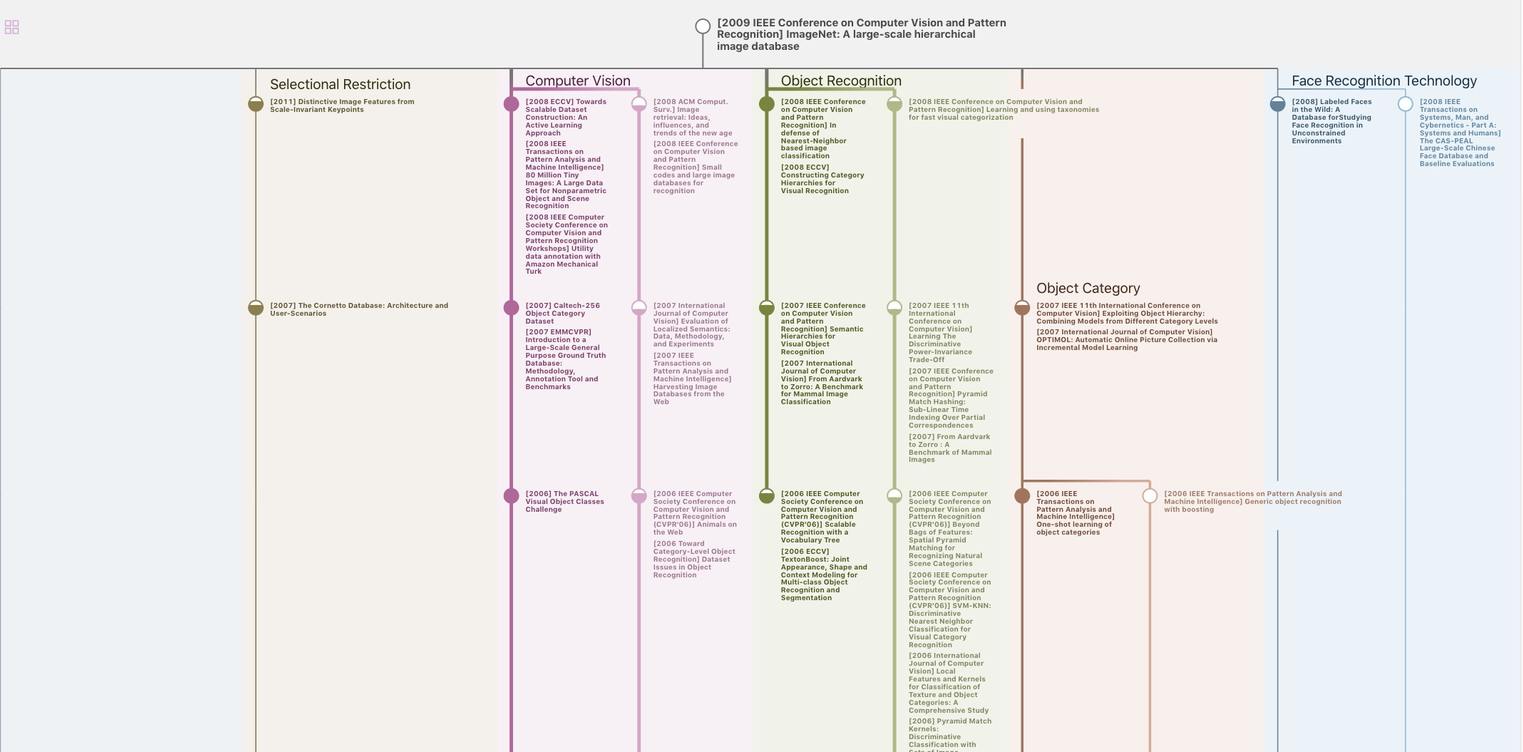
生成溯源树,研究论文发展脉络
Chat Paper
正在生成论文摘要