Frequency-domain digital predistortion for Massive MU-MIMO-OFDM Downlink.
GLOBECOM(2022)
摘要
Digital predistortion (DPD) is a method commonly used to compensate for the nonlinear effects of power amplifiers (PAs). However, the computational complexity of most DPD algorithms becomes an issue in the downlink of massive multi-user (MU) multiple-input multiple-output (MIMO) orthogonal frequency division multiplexing (OFDM), where potentially up to several hundreds of PAs in the base station (BS) require linearization. In this paper, we propose a convolutional neural network (CNN)-based DPD in the frequency domain, taking place before the precoding, where the dimensionality of the signal space depends on the number of users, instead of the number of BS antennas. Simulation results on generalized memory polynomial (GMP)-based PAs show that the proposed CNN-based DPD can lead to very large complexity savings as the number of BS antenna increases at the expense of a small increase in power to achieve the same symbol error rate (SER).
更多查看译文
关键词
base station,BS antenna increases,BS antennas,CNN-based DPD,computational complexity,convolutional neural network,convolutional neural network-based DPD,DPD algorithms,frequency domain,frequency-domain digital predistortion,generalized memory polynomial-based PA,massive MU-MIMO-OFDM downlink,massive multiuser multiple-input multiple-output,nonlinear effects,orthogonal frequency division multiplexing,power amplifiers,precoding,signal space,symbol error rate
AI 理解论文
溯源树
样例
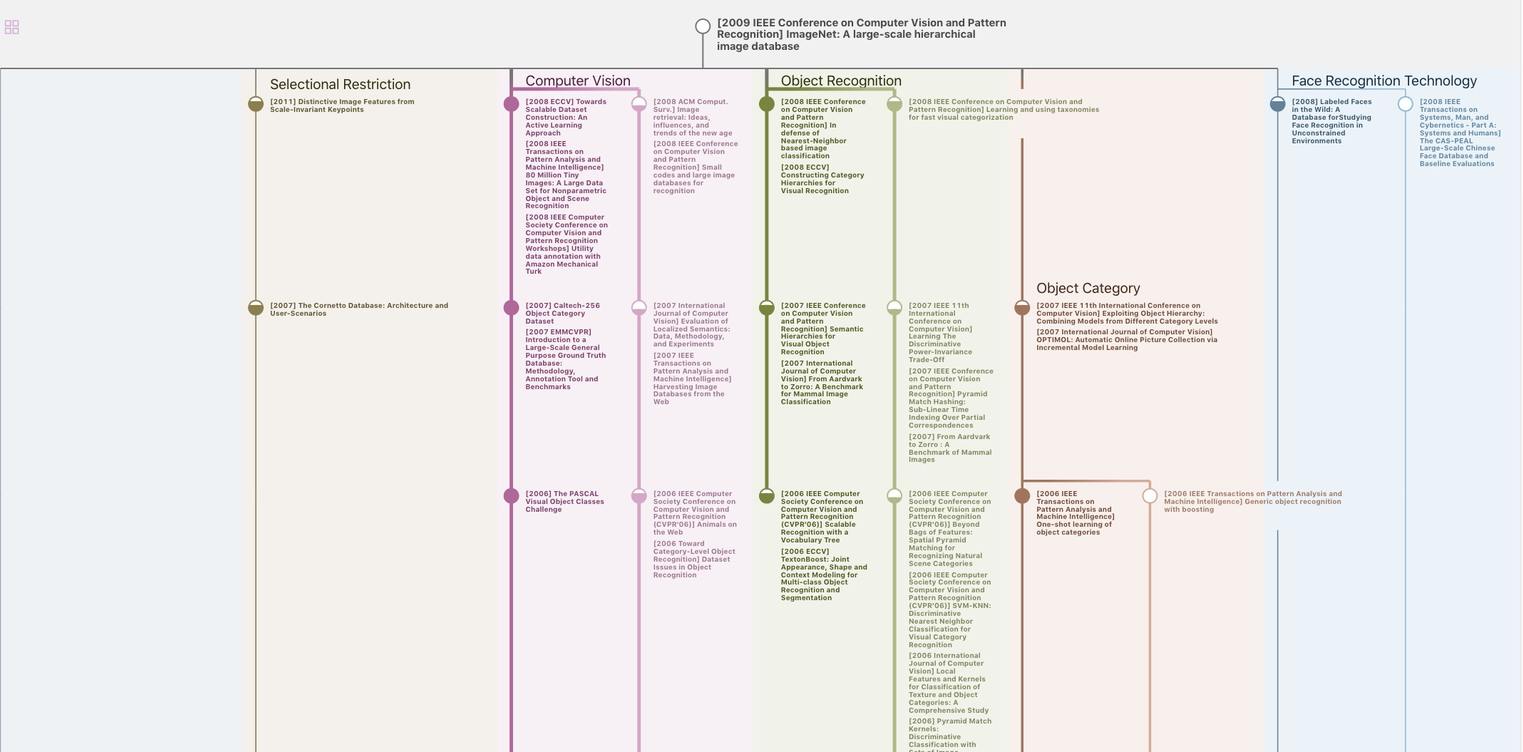
生成溯源树,研究论文发展脉络
Chat Paper
正在生成论文摘要