Triple-discriminator GAN for semi-supervised generalized zero-shot learning
Neurocomputing(2022)
摘要
Generalized zero-shot learning (GZSL) is a newly rising topic that has been attracting an increasing amount of attention. However, there is currently a paradox in existing semi-supervised GZSL methods, in that most of them are transductive. This requires prior knowledge of unseen classes, which violates the premise that unseen class samples cannot be obtained in GZSL. In this paper, we consider a more practical setting, the semi-supervised inductive problem. This setting only requires the data of seen classes in the training stage, and each class only needs to label a few samples. After that, we migrate some transductive methods to this the new setting and reevaluate their accuracy. However, most of these methods perform poorly in new setting. We analyze their pros and cons deduce a more reasonable model, and finally, use a trainable network to instantiate it. The model has a dual structure of mutual learning, which can make full use of both labeled data and unlabeled data. Extensive experiments demonstrate the state-of-the-art performance of our model in transductive and semi-supervised inductive settings.
更多查看译文
关键词
00-01,99-00
AI 理解论文
溯源树
样例
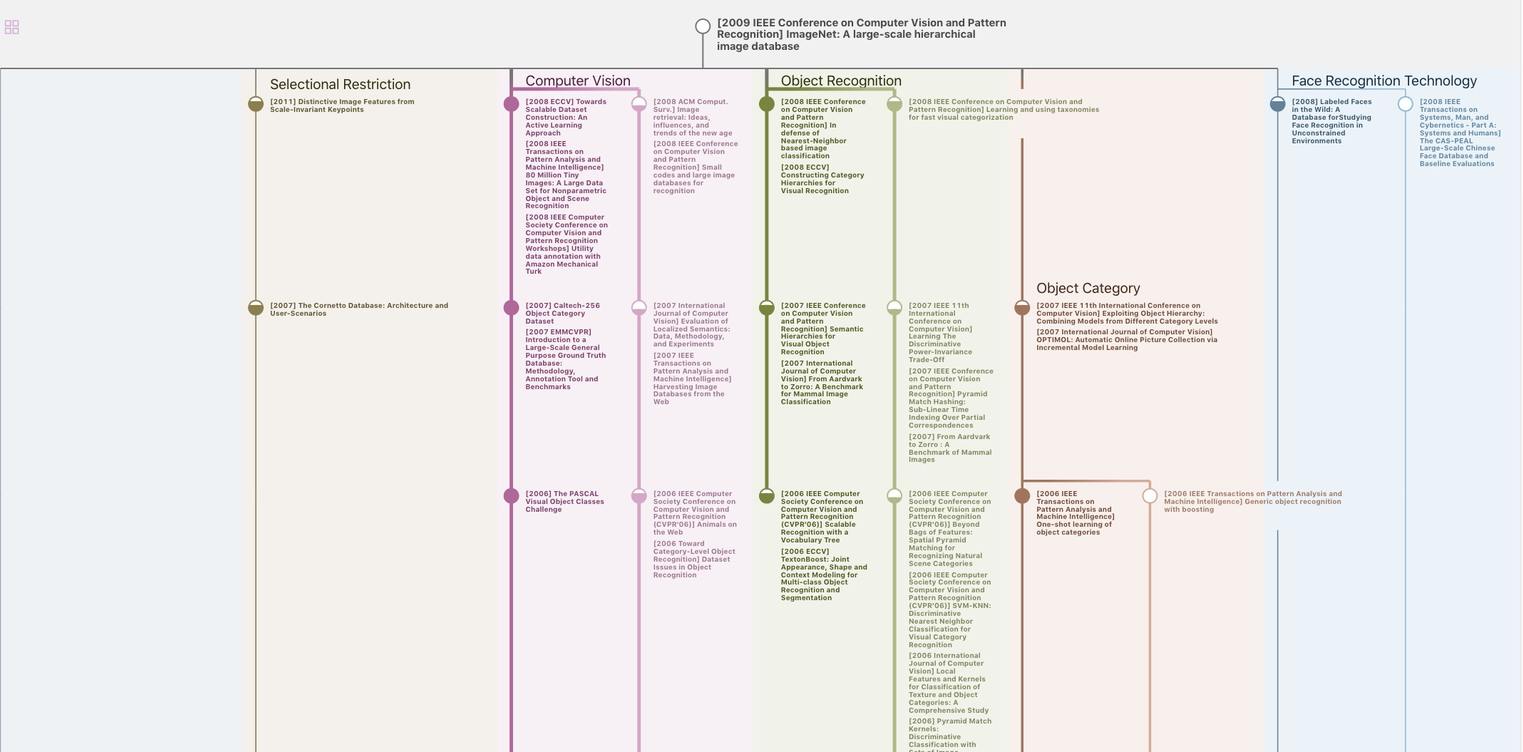
生成溯源树,研究论文发展脉络
Chat Paper
正在生成论文摘要