Top-of-line corrosion via physics-guided machine learning: A methodology integrating field data with theoretical models
Journal of Petroleum Science and Engineering(2022)
摘要
An artificial learning method for predicting top-of-line corrosion (TOLC) in oil/gas production from an existing oil field production data was presented, along with verifications and a few real-world applications. The developed algorithm was aimed to boost fundamental understanding of the day-to-day unit operations and periodic maintenance and inspection of oil/gas pipelines via a particular inline inspection technique (magnetic-flux-leakage measurements) and corrosion inhibitor applications. A recurrent neural network was utilized to establish a model for oil field production while a series of small neural networks representing the fundamental physics of TOLCs were included. The relevant physical models are those relating to (i) electrochemistry of corrosion mechanisms, (ii) gas–liquid phase flows and atomizations, and (iii) the condensation rates at the top of the lines. By incorporating these networks into the operation data, the developed learning algorithm gives accurate TOLC prediction for the complex oil field structures. The results show that the estimation models based on the physics-guided artificial neural network present the root-mean-square error within 10%. The developed software also offers insights into the essential parameters for the better prevention of the TOLC in pipelines.
更多查看译文
关键词
Top-of-line corrosion,Water condensation,Corrosion inhibitors,Machine learning
AI 理解论文
溯源树
样例
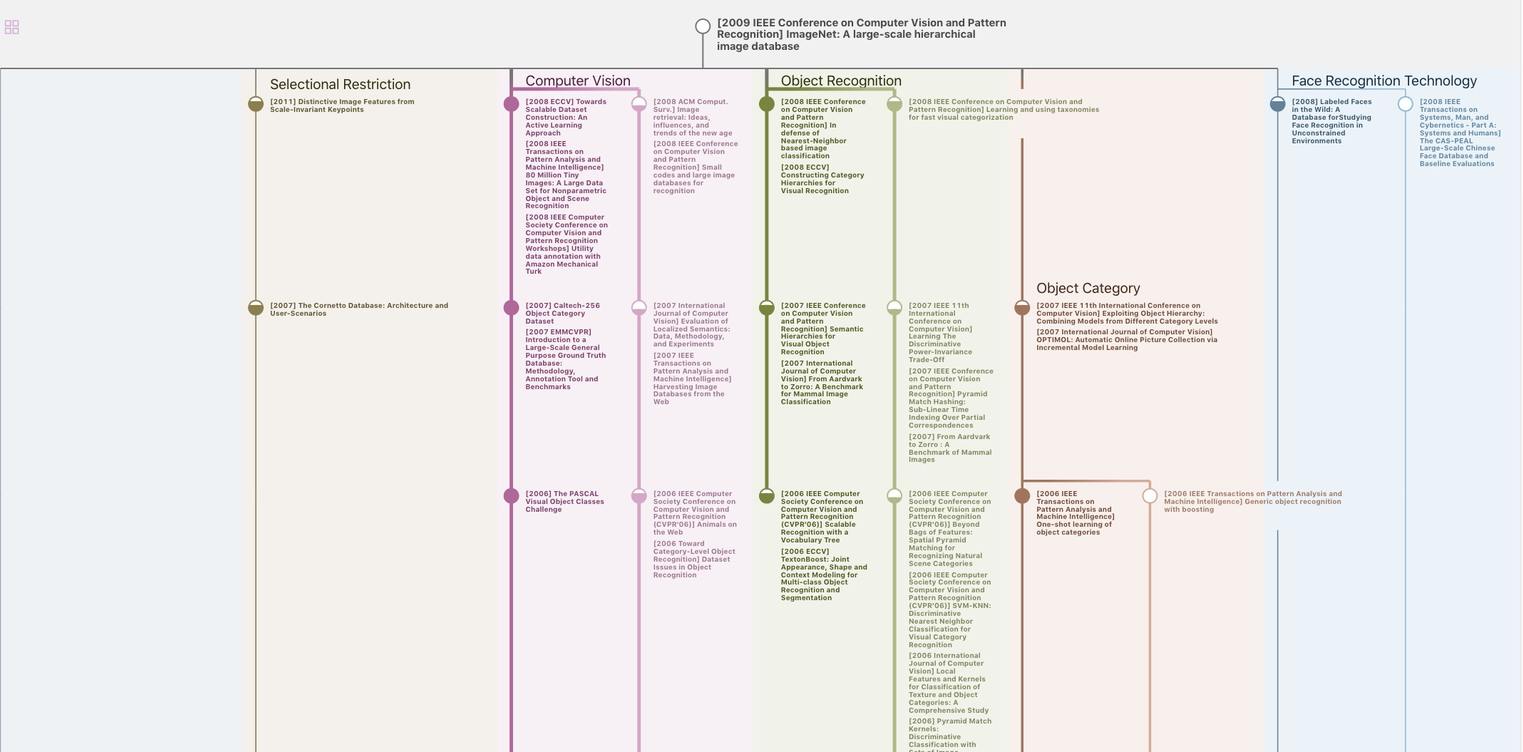
生成溯源树,研究论文发展脉络
Chat Paper
正在生成论文摘要