Data-Driven Approximations of Chance Constrained Programs in Nonstationary Environments
IEEE CONTROL SYSTEMS LETTERS(2022)
摘要
We study sample average approximations (SAA) of chance constrained programs. SAA methods typically approximate the actual distribution in the chance constraint using an empirical distribution constructed from random samples assumed to be independent and identically distributed according to the actual distribution. In this letter, we consider a nonstationary variant of this problem, where the random samples are assumed to be independently drawn in a sequential fashion from an unknown and possibly time-varying distribution. This nonstationarity may be driven by changing environmental conditions present in many real-world applications. To account for the potential nonstationarity in the data generation process, we propose a novel robust SAA method exploiting information about the Wasserstein distance between the sequence of data-generating distributions and the actual chance constraint distribution. As a key result, we obtain distribution-free estimates of the sample size required to ensure that the robust SAA method will yield solutions that are feasible for the chance constraint under the actual distribution with high confidence.
更多查看译文
关键词
Upper bound, Optimization, Random variables, Uncertainty, Symbols, Probability distribution, Measurement, Chance constrained programs, data-driven optimization, nonstationary environments, wasserstein metric
AI 理解论文
溯源树
样例
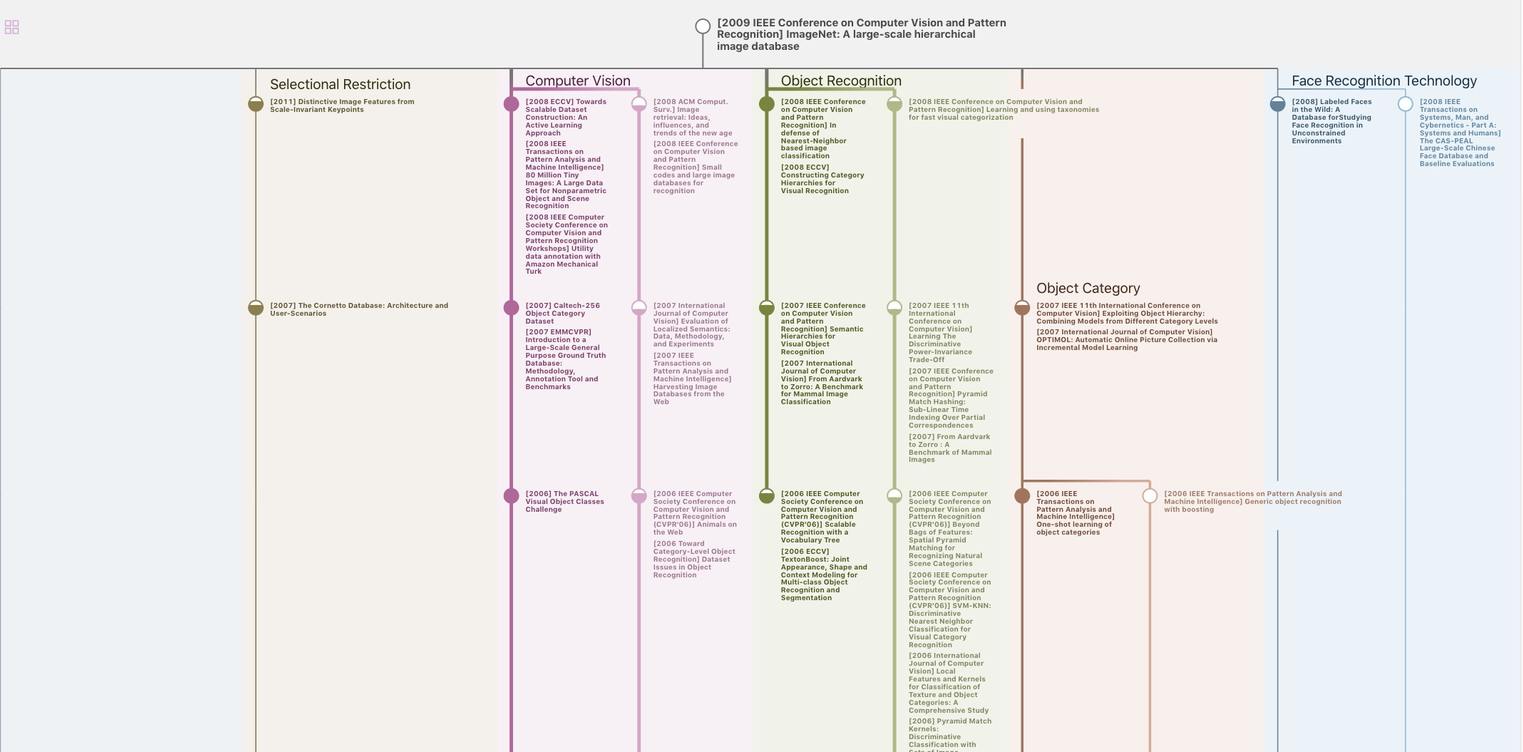
生成溯源树,研究论文发展脉络
Chat Paper
正在生成论文摘要