Graph-level Semantic Matching model for Knowledge base Aggregate Question Answering.
International Workshop on Multimodal Human Understanding for the Web and Social Media(2022)
摘要
In knowledge base question answering, complex question always has long-distance dependencies, especially aggregate question, which affects query graph matching. Many previous approaches have made conspicuous progress in complex question answering. However, they mostly only compare based on the textual similarity of the predicate sequences, ignoring the degree of semantic information either questions or query graphs. In this paper, we propose a Graph-level Semantic Matching (GSM) model to obtain the global semantics representation. Due to the structural complexity of query graphs, we propose a global semantic model to explicitly encode the structural and relational semantics of query graphs. Then, a question-guiding mechanism is applied to enhance the understanding of question semantics in query graph representation. Finally, GSM outperforms existing question answering models, and exhibits capabilities to deal with aggregate questions, e.g., correctly handling counting and comparison in questions.
更多查看译文
AI 理解论文
溯源树
样例
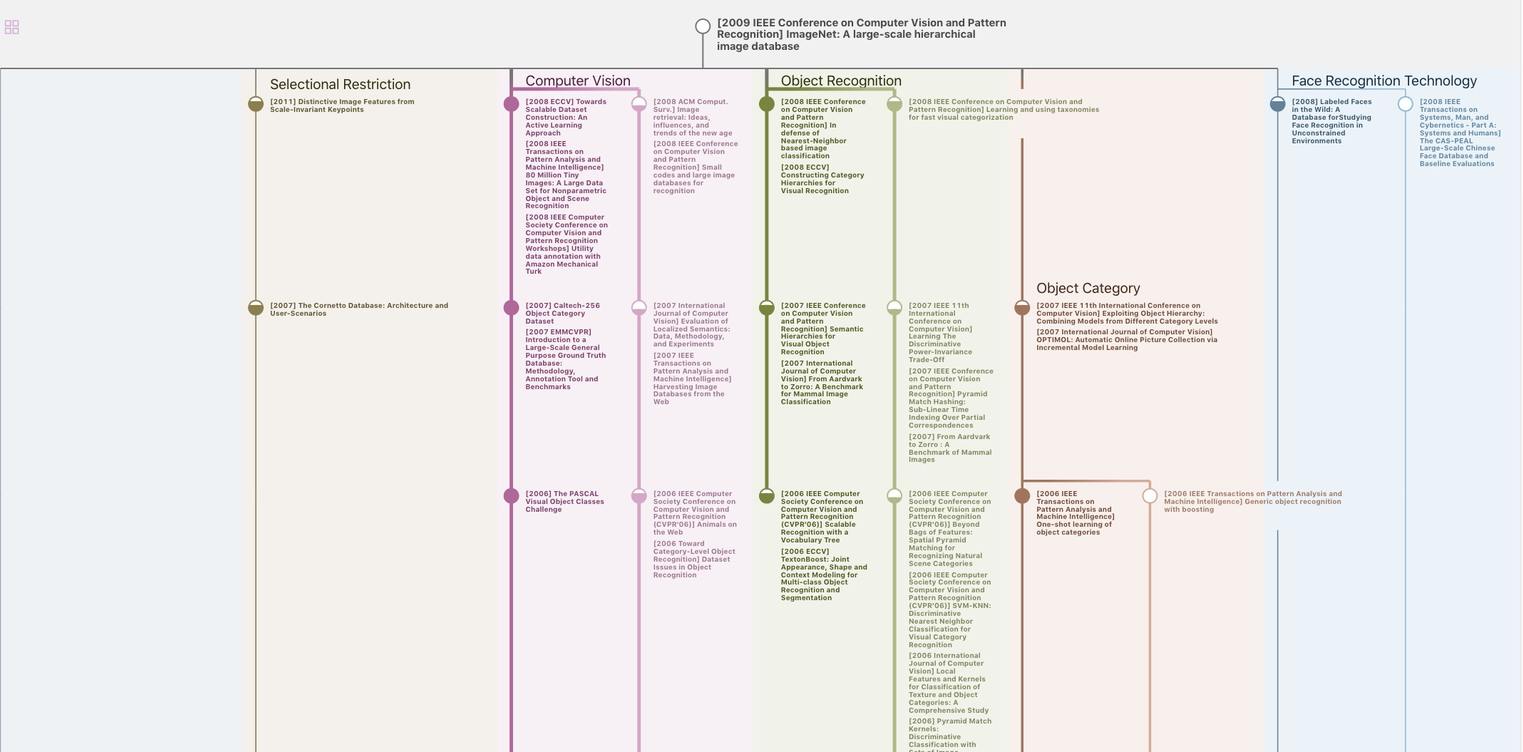
生成溯源树,研究论文发展脉络
Chat Paper
正在生成论文摘要