PNMTA: A Pretrained Network Modulation and Task Adaptation Approach for User Cold-Start Recommendation
International World Wide Web Conference(2022)
摘要
ABSTRACT User cold-start recommendation is a serious problem that limits the performance of recommender systems (RSs). Recent studies have focused on treating this issue as a few-shot problem and seeking solutions with model-agnostic meta-learning (MAML). Such methods regard making recommendations for one user as a task and adapt to new users with a few steps of gradient updates on the meta-model. However, none of those methods consider the limitation of user representation learning imposed by the special task setting of MAML-based RSs. And they learn a common meta-model for all users while ignoring the implicit grouping distribution induced by the correlation differences among users. In response to the above problems, we propose a pretrained network modulation and task adaptation approach (PNMTA) for user cold-start recommendation. In the pretraining stage, a pretrained model is obtained with non-meta-learning methods to achieve better user representation and generalization, which can also transfer the learned knowledge to the meta-learning stage for modulation. During the meta-learning stage, an encoder modulator is utilized to realize the memorization and correction of prior parameters for the meta-learning task, and a predictor modulator is introduced to condition the model initialization on the task identity for adaptation steps. In addition, PNMTA can also make use of the existing non-cold-start users for pretraining. Comprehensive experiments on two benchmark datasets demonstrate that our model can achieve significant and consistent improvements against other state-of-the-art methods.
更多查看译文
关键词
Recommender systems, Cold-start problem, Meta learning, Transfer learning
AI 理解论文
溯源树
样例
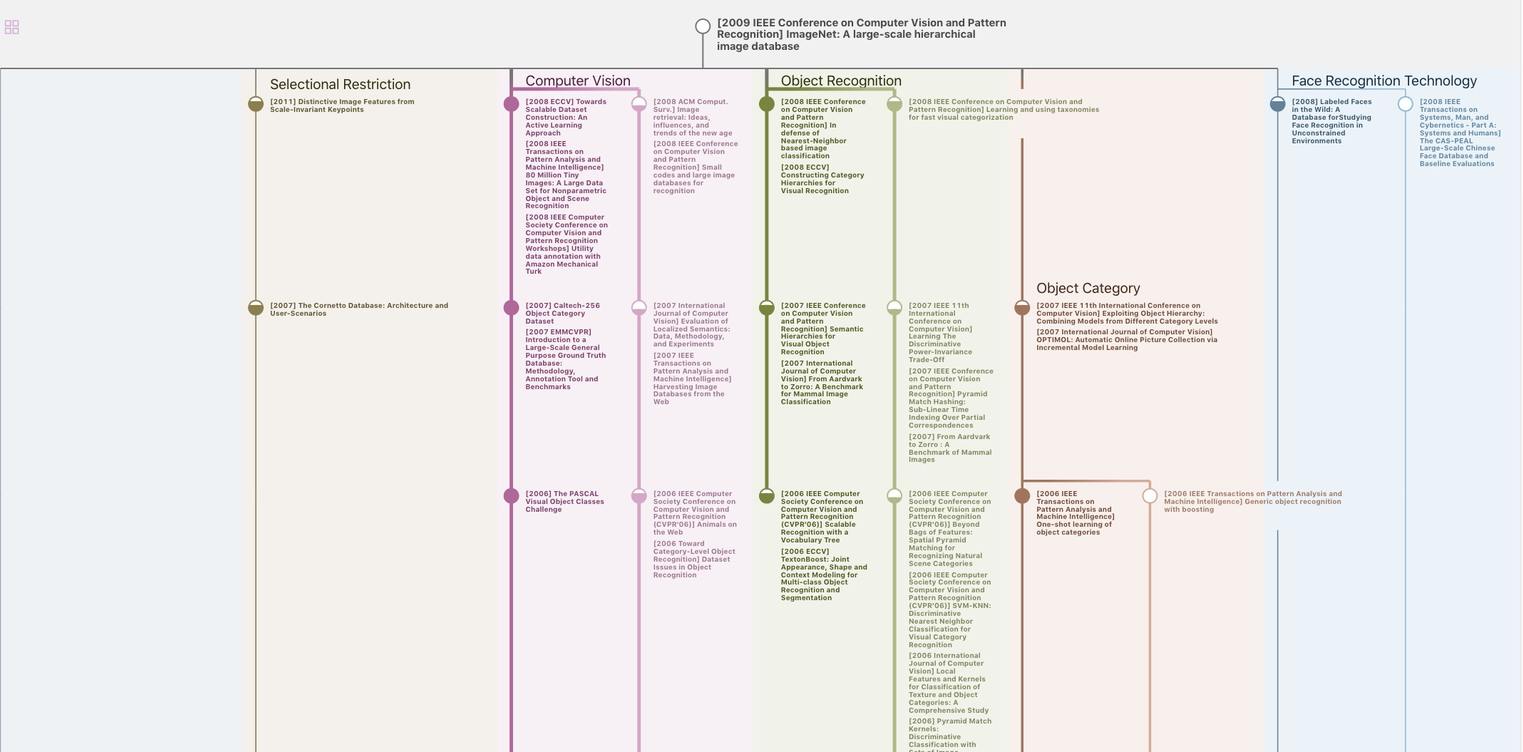
生成溯源树,研究论文发展脉络
Chat Paper
正在生成论文摘要