Geospatial Entity Resolution
International World Wide Web Conference(2022)
摘要
ABSTRACT A geospatial database is today at the core of an ever increasing number of services. Building and maintaining it remains challenging due to the need to merge information from multiple providers. Entity Resolution (ER) consists of finding entity mentions from different sources that refer to the same real world entity. In geospatial ER, entities are often represented using different schemes and are subject to incomplete information and inaccurate location, making ER and deduplication daunting tasks. While tremendous advances have been made in traditional entity resolution and natural language processing, geospatial data integration approaches still heavily rely on static similarity measures and human-designed rules. In order to achieve automatic linking of geospatial data, a unified representation of entities with heterogeneous attributes and their geographical context, is needed. To this end, we propose Geo-ER1, a joint framework that combines Transformer-based language models, that have been successfully applied in ER, with a novel learning-based architecture to represent the geospatial character of the entity. Different from existing solutions, Geo-ER does not rely on pre-defined rules and is able to capture information from surrounding entities in order to make context-based, accurate predictions. Extensive experiments on eight real world datasets demonstrate the effectiveness of our solution over state-of-the-art methods. Moreover, Geo-ER proves to be robust in settings where there is no available training data for a specific city.
更多查看译文
关键词
Entity resolution, neural networks, geospatial data, neighbourhood embedding, graph attention
AI 理解论文
溯源树
样例
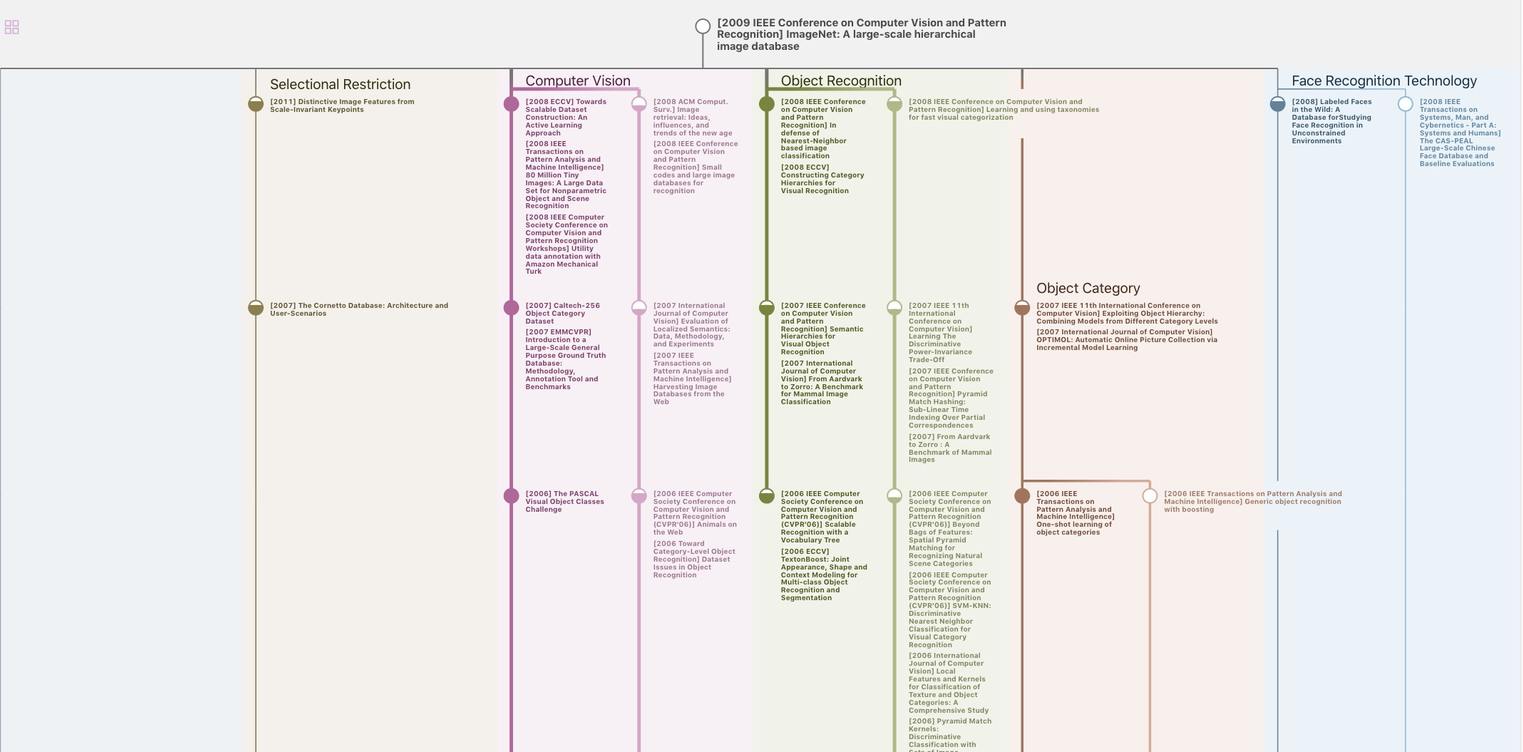
生成溯源树,研究论文发展脉络
Chat Paper
正在生成论文摘要