Knowledge-based Temporal Fusion Network for Interpretable Online Video Popularity Prediction
International World Wide Web Conference(2022)
摘要
ABSTRACT Predicting the popularity of online videos has many real-world applications, such as recommendation, precise advertising, and edge caching strategies. Despite many efforts have been dedicated to the online video popularity prediction, there still exist several challenges: (1) The meta-data from online videos is usually sparse and noisy, which makes it difficult to learn a stable and robust representation. (2) The influence of content features and temporal features in different life cycles of online videos is dynamically changing, so it is necessary to build a model that can capture the dynamics. (3) Besides, there is a great need to interpret the predictive behavior of the model to assist administrators of video platforms in the subsequent decision-making. In this paper, we propose a Knowledge-based Temporal Fusion Network (KTFN) that incorporates knowledge graph representation to address the aforementioned challenges in the task of online video popularity prediction. To be more specific, we design a Tree Attention Network (TAN) to learn the embedding of online video entities in knowledge graphs via selectively aggregating local neighborhood information, thus enabling our model to learn the importance of different entities under the same relation. Besides, an Attention-based Long Short-Term Memory (ALSTM) is utilized to learn the temporal feature representation. Finally, we propose an Adaptively Temporal Feature Fusion (ATFF) scheme to adaptively fuse content features and temporal features, in which a learnable exponential decay function with the global attention mechanism is constructed. We collect two large-scale real-world datasets from the server logs of two popular Chinese online video platforms, and experimental results on the two datasets have demonstrated the superiority and interpretability of KTFN.
更多查看译文
关键词
Video popularity prediction, Knowledge graph, Attention model, Graph neural networks
AI 理解论文
溯源树
样例
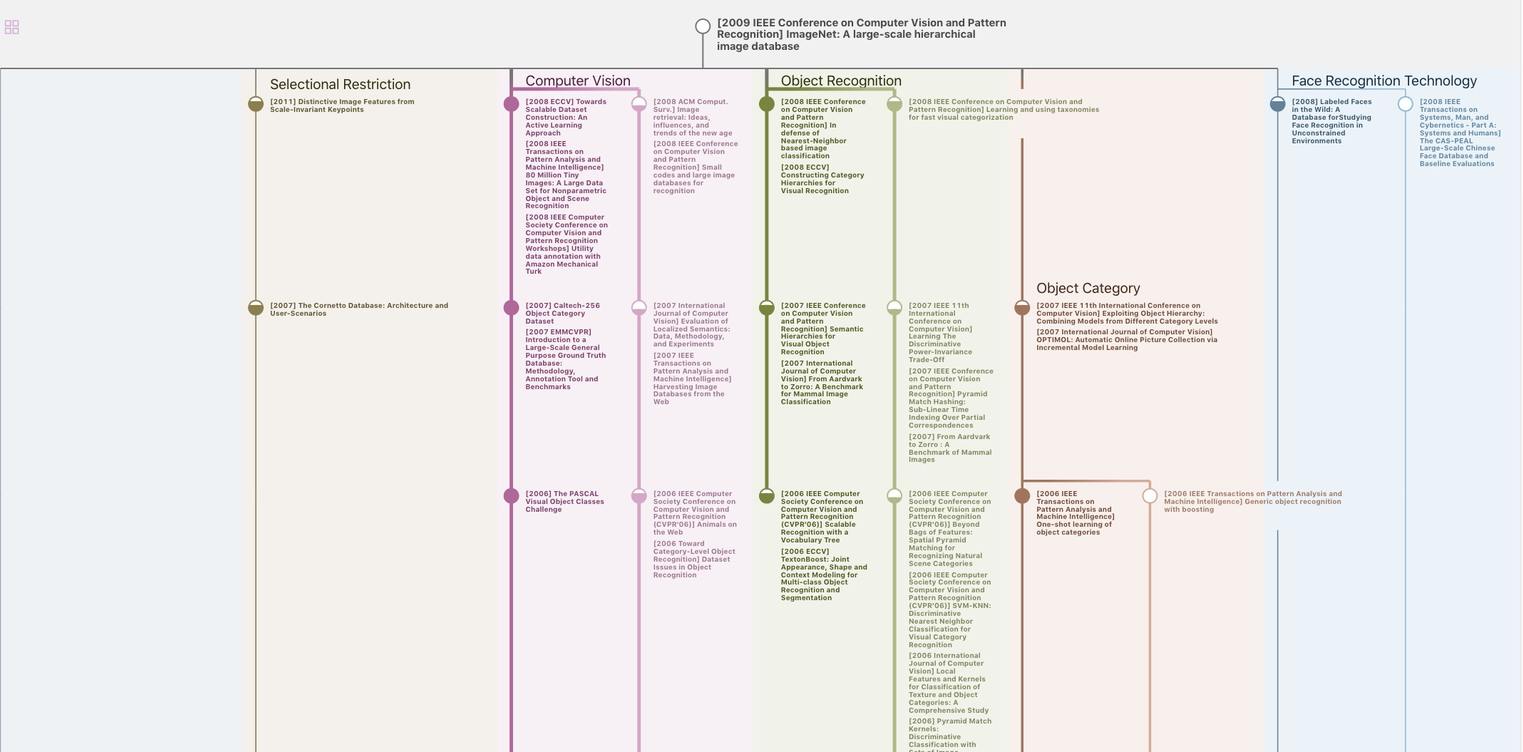
生成溯源树,研究论文发展脉络
Chat Paper
正在生成论文摘要