Can I only share my eyes? A Web Crowdsourcing based Face Partition Approach Towards Privacy-Aware Face Recognition
International World Wide Web Conference(2022)
摘要
ABSTRACT Human face images represent a rich set of visual information for online social media platforms to optimize the machine learning (ML)/AI models in their data-driven facial applications (e.g., face detection, face recognition). However, there exists a growing privacy concern from social media users to share their online face images that will be annotated by unknown crowd workers and analyzed by ML/AI researchers in the model training and optimization process. In this paper, we focus on a privacy-aware face recognition problem where the goal is to empower the facial applications to train their face recognition models with images shared by social media users while protecting the identity of the users. Our problem is motivated by the limitation of current privacy-aware face recognition approaches that mainly prevent algorithmic attacks by manipulating face images but largely ignore the potential privacy leakage related to human activities (e.g., crowdsourcing annotation). To address such limitations, we develop FaceCrowd, a web crowdsourcing based face partition approach to improve the performance of current face recognition models by designing a novel crowdsourced partial face graph generated from privacy-preserved social media face images. We evaluate the performance of FaceCrowd using two real-world human face datasets that consist of large-scale human face images. The results show that FaceCrowd not only improves the accuracy of the face recognition models but also effectively protects the identity information of the social media users who share their face images.
更多查看译文
关键词
Crowdsourcing, Privacy-aware, Face Recognition
AI 理解论文
溯源树
样例
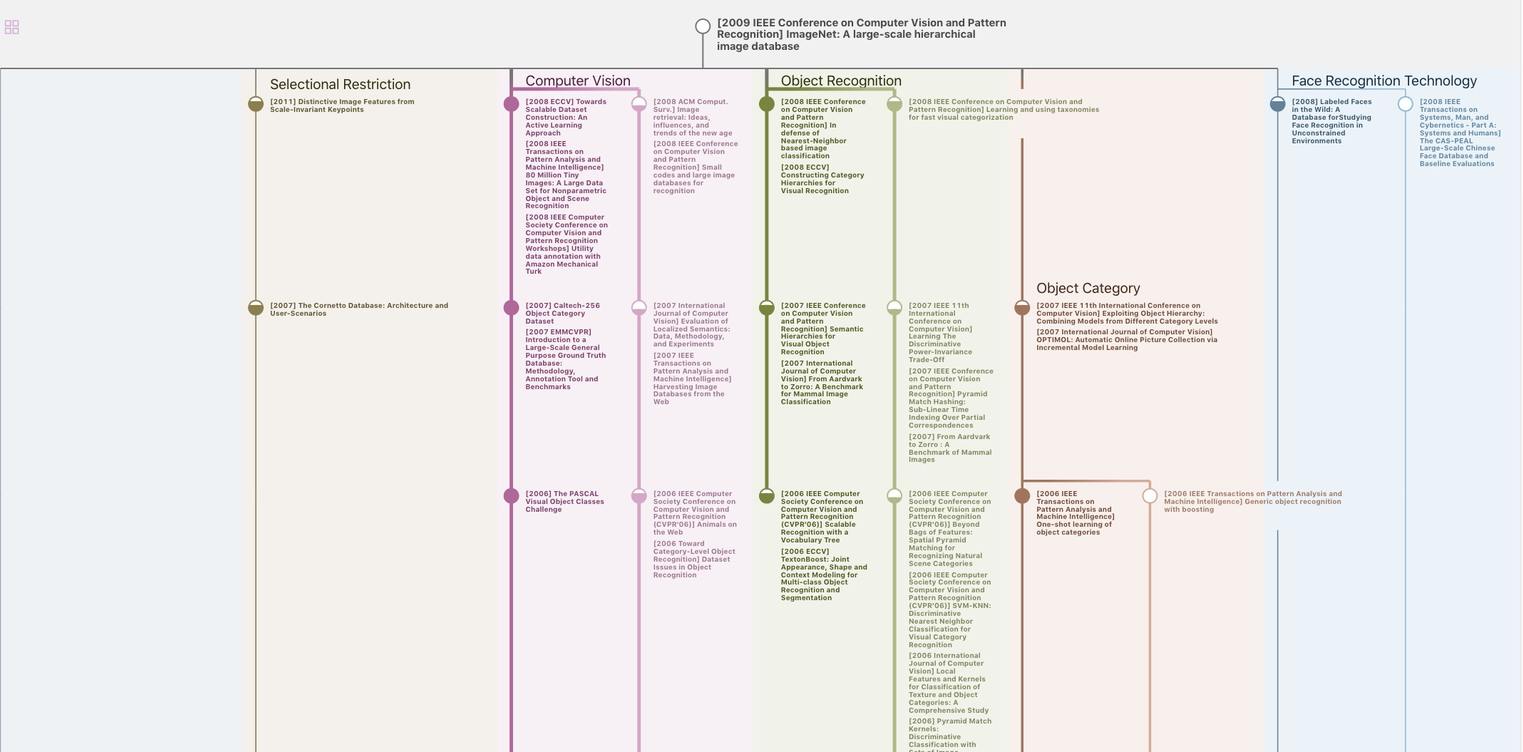
生成溯源树,研究论文发展脉络
Chat Paper
正在生成论文摘要