Preserve Pre-trained Knowledge: Transfer Learning With Self-Distillation For Action Recognition
arxiv(2022)
摘要
Video-based action recognition is one of the most popular topics in computer vision. With recent advances of selfsupervised video representation learning approaches, action recognition usually follows a two-stage training framework, i.e., self-supervised pre-training on large-scale unlabeled sets and transfer learning on a downstream labeled set. However, catastrophic forgetting of the pre-trained knowledge becomes the main issue in the downstream transfer learning of action recognition, resulting in a sub-optimal solution. In this paper, to alleviate the above issue, we propose a novel transfer learning approach that combines self-distillation in fine-tuning to preserve knowledge from the pre-trained model learned from the large-scale dataset. Specifically, we fix the encoder from the last epoch as the teacher model to guide the training of the encoder from the current epoch in the transfer learning. With such a simple yet effective learning strategy, we outperform state-of-the-art methods on widely used UCF101 and HMDB51 datasets in action recognition task.
更多查看译文
AI 理解论文
溯源树
样例
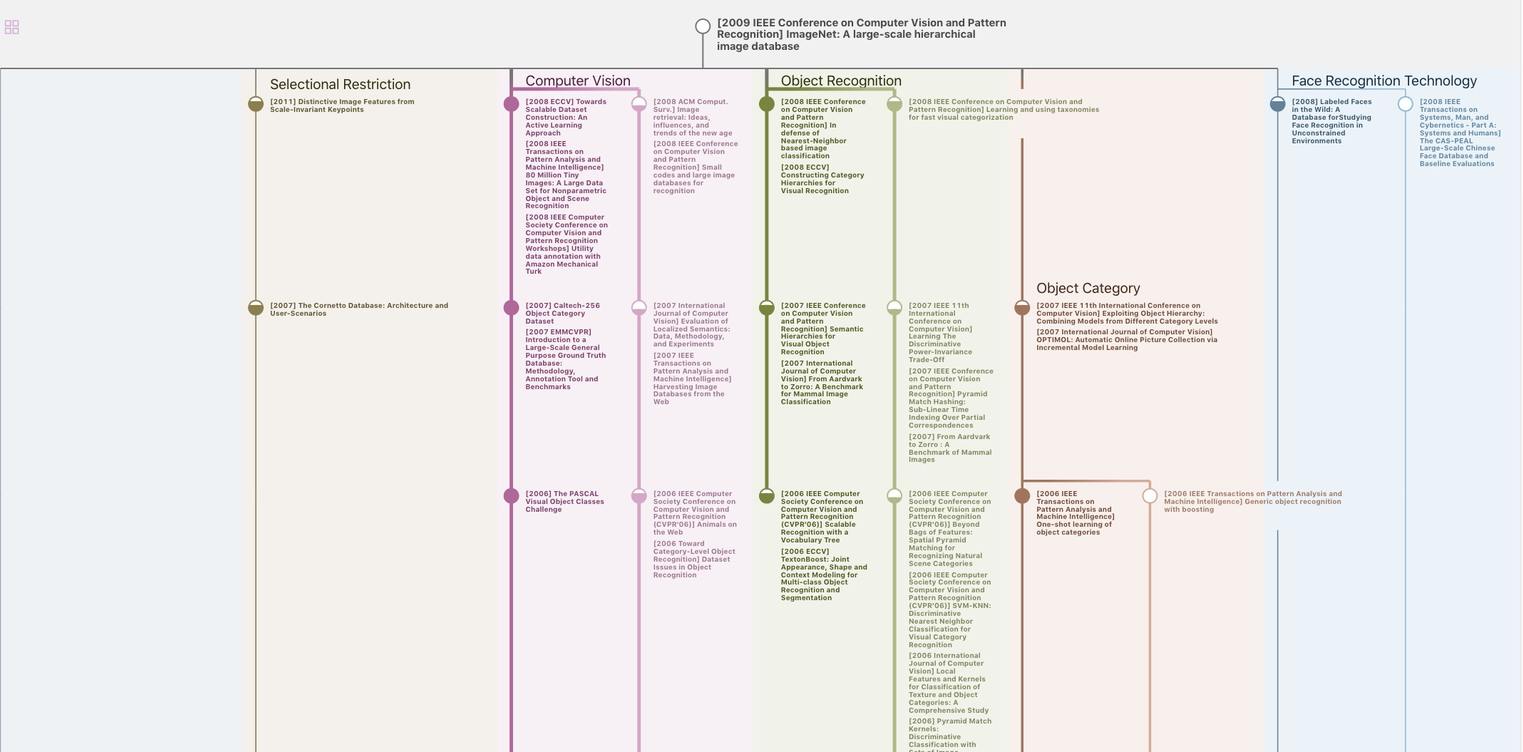
生成溯源树,研究论文发展脉络
Chat Paper
正在生成论文摘要