Size Generalization for Resource Allocation with Graph Neural Networks
arxiv(2022)
摘要
Size generalization is important for learning wireless policies, which are often with dynamic sizes, say caused by time-varying number of users. Recent works of learning to optimize resource allocation empirically demonstrate that graph neural networks (GNNs) can generalize to different problem scales. However, GNNs are not guaranteed to generalize across input sizes. In this paper, we strive to analyze the size generalization mechanism of GNNs when learning permutation equivariant (PE) policies. We find that the aggregation function and activation functions of a GNN play a key role on its size generalization ability. We take the GNN with mean aggregator, called mean-GNN, as an example to demonstrate a size generalization condition, and interpret why several GNNs in the literature of wireless communications can generalize well to problem scales. To illustrate how to design GNNs with size generalizability according to our finding, we consider power and bandwidth allocation, and suggest to select or pre-train activation function in the output layer of mean-GNN for learning the PE policies. Simulation results show that the proposed GNN can generalize well to the number of users, which validate our analysis for the size generalization condition of GNNs when learning the PE policies.
更多查看译文
关键词
resource allocation,size generalization,neural networks
AI 理解论文
溯源树
样例
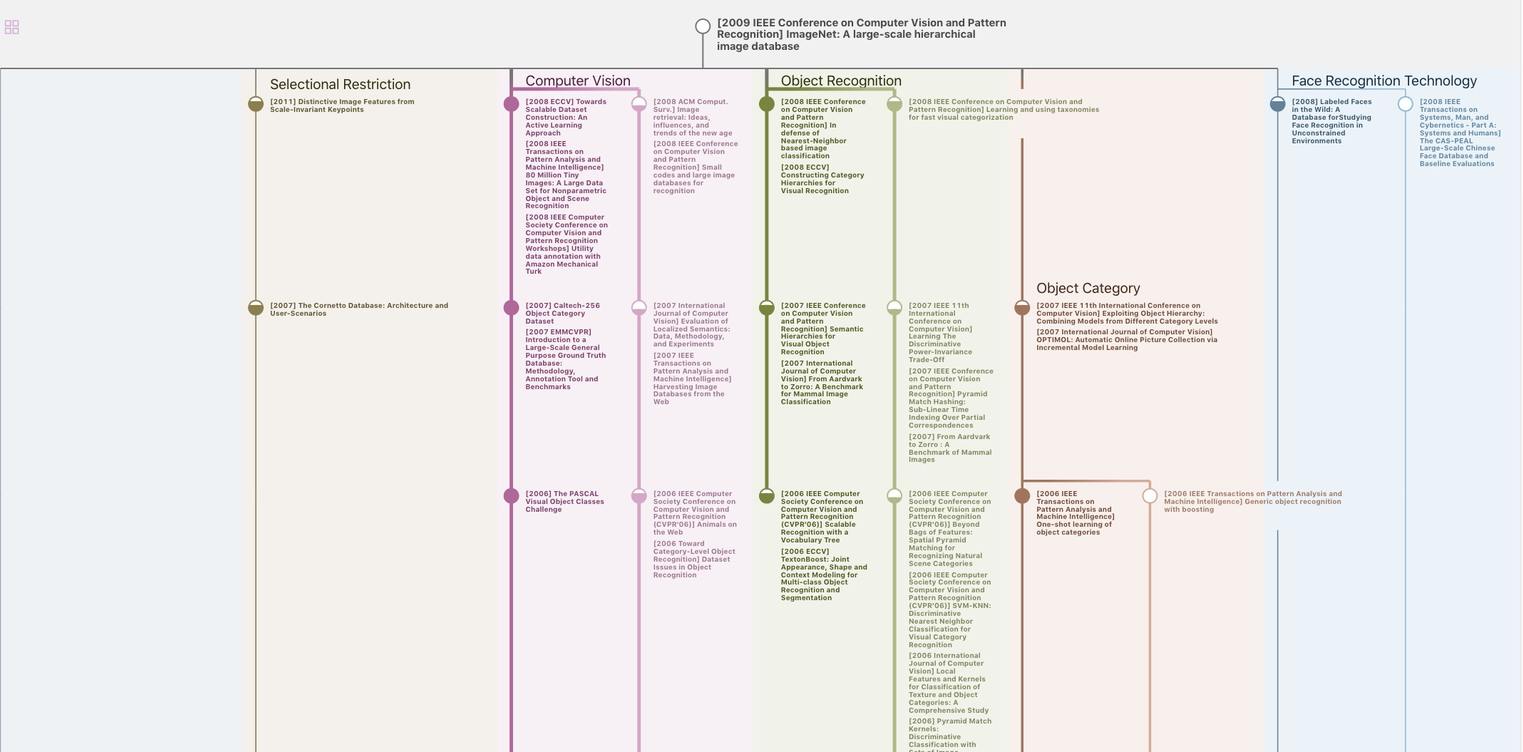
生成溯源树,研究论文发展脉络
Chat Paper
正在生成论文摘要