Noise-reducing attention cross fusion learning transformer for histological image classification of osteosarcoma
BIOMEDICAL SIGNAL PROCESSING AND CONTROL(2022)
摘要
The degree of malignancy of osteosarcoma and its tendency to metastasize/spread mainly depend on the pathological grade (determined by observing the morphology of the tumor under a microscope). The purpose of this study is to use artificial intelligence to classify osteosarcoma histological images and to assess tumor survival and necrosis, which will help doctors reduce their workload, improve the accuracy of osteosarcoma cancer detection, and make a better prognosis for patients. The study proposes a typical transformer image classification framework by integrating noise reduction convolutional autoencoder and feature cross fusion learning (NRCAFCFL) to classify osteosarcoma histological images. Noise reduction convolutional autoencoder could well denoise histological images of osteosarcoma, resulting in more pure images for osteosarcoma classification. Moreover, we introduce feature cross fusion learning, which integrates two scale image patches, to sufficiently explore their interactions by using additional classification tokens. As a result, a refined fusion feature is generated, which is fed to the residual neural network for label predictions. We conduct extensive experiments to evaluate the performance of the proposed approach. The experimental results demonstrate that our method outperforms the traditional and deep learning approaches on various evaluation metrics, with an accuracy of 99.17% to support osteosarcoma diagnosis.
更多查看译文
关键词
Osteosarcoma, Denoise, Transformer, Feature fusion, Framework, Diagnose
AI 理解论文
溯源树
样例
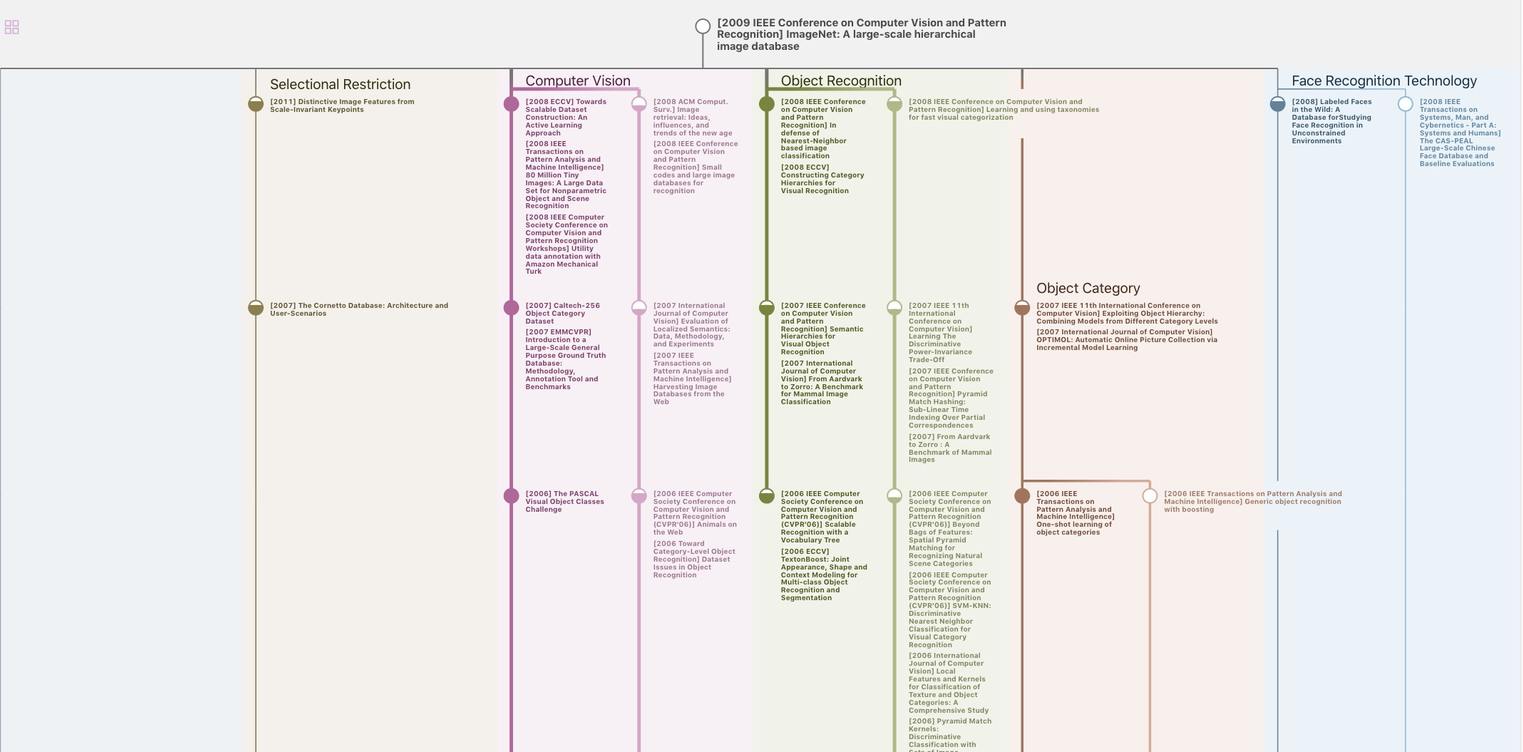
生成溯源树,研究论文发展脉络
Chat Paper
正在生成论文摘要