FPIRM: Floating-point Processing in Racetrack Memories
arxiv(2022)
摘要
Convolutional neural networks (CNN) have become a ubiquitous algorithm with growing applications in mobile and edge settings. We describe a compute-in-memory (CIM) technique called FPIRM using Racetrack Memory (RM) to accelerate CNNs for edge systems. Using transverse read, a technique that can determine the number of '1's multiple adjacent domains, FPIRM can efficiently implement multi-operand bulk-bitwise and addition computations, and two-operand multiplication. We discuss how FPIRM can implement both variable precision integer and floating point arithmetic. This allows both CNN inference and on-device training without expensive data movement to the cloud. Based on these functions we demonstrate implementation of several CNNs with back propagation using RM CIM and compare these to state-of-the-art implementations of CIM inference and training in Field-Programmable Gate Arrays. During training FPIRM improves by 2$\times$ the efficiency, by reducing the energy consumption by at least 27% and increasing the throughput by at least 18% against FPGA.
更多查看译文
关键词
racetrack memories,processing,floating-point
AI 理解论文
溯源树
样例
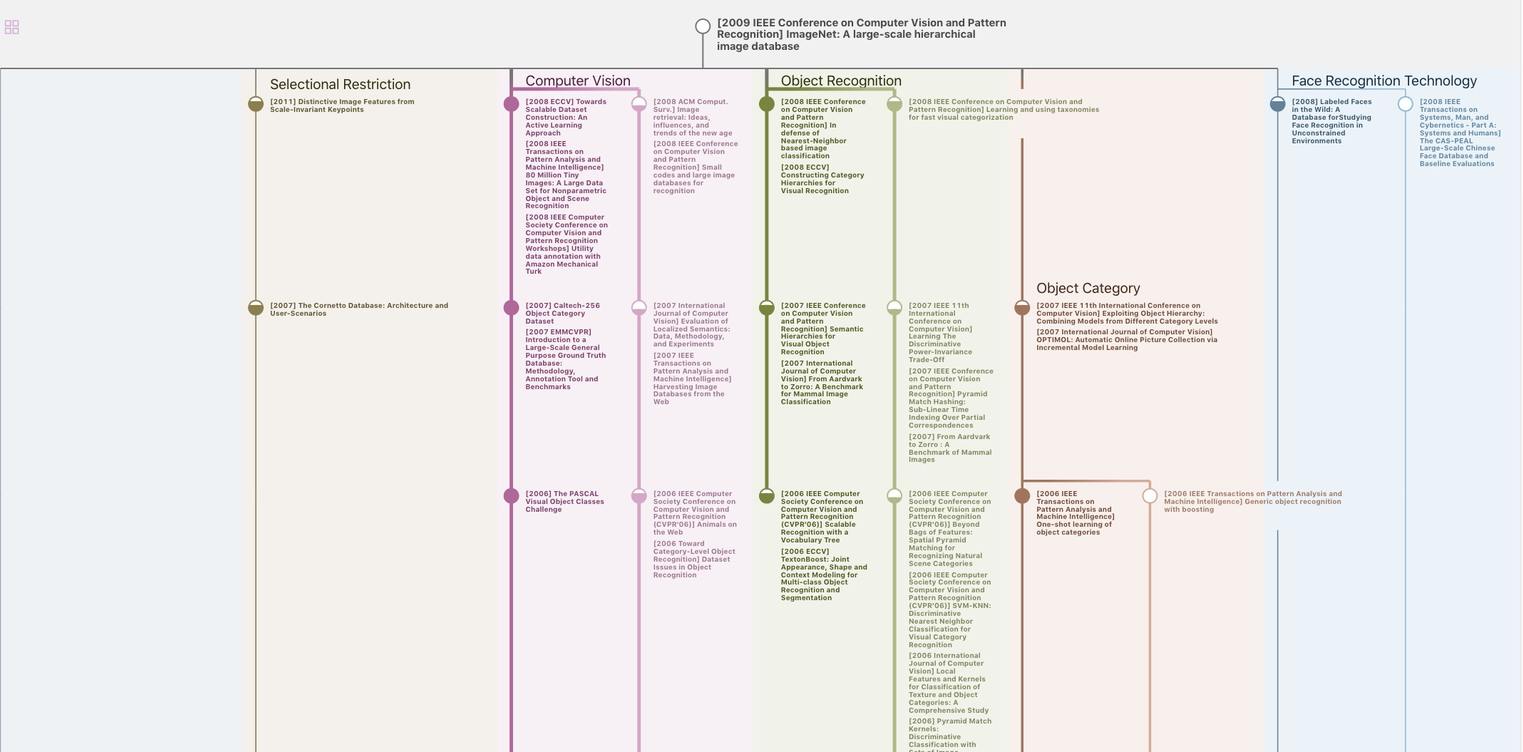
生成溯源树,研究论文发展脉络
Chat Paper
正在生成论文摘要