Channel response-aware photonic neural network accelerators for high-speed inference through bandwidth-limited optics
OPTICS EXPRESS(2022)
摘要
Photonic neural network accelerators (PNNAs) have been lately brought into the spotlight as a new class of custom hardware that can leverage the maturity of photonic integration towards addressing the low-energy and computational power requirements of deep learning (DL) workloads. Transferring, however, the high-speed credentials of photonic circuitry into analogue neuromorphic computing necessitates a new set of DL training methods aligned along certain analogue photonic hardware characteristics. Herein, we present a novel channel response-aware (CRA) DL architecture that can address the implementation challenges of high-speed compute rates on bandwidth-limited photonic devices by incorporating their frequency response into the training procedure. The proposed architecture was validated both through software and experimentally by implementing the output layer of a neural network (NN) that classifies images of the MNIST dataset on an integrated SiPho coherent linear neuron (COLN) with a 3dB channel bandwidth of 7 GHz. A comparative analysis between the baseline and CRA model at 20, 25 and 32GMAC/sec/axon revealed respective experimental accuracies of 98.5%, 97.3% and 92.1% for the CRA model, outperforming the baseline model by 7.9%, 12.3% and 15.6%, respectively. (C) 2022 Optica Publishing Group under the terms of the Optica Open Access Publishing Agreement
更多查看译文
关键词
response-aware,high-speed,bandwidth-limited
AI 理解论文
溯源树
样例
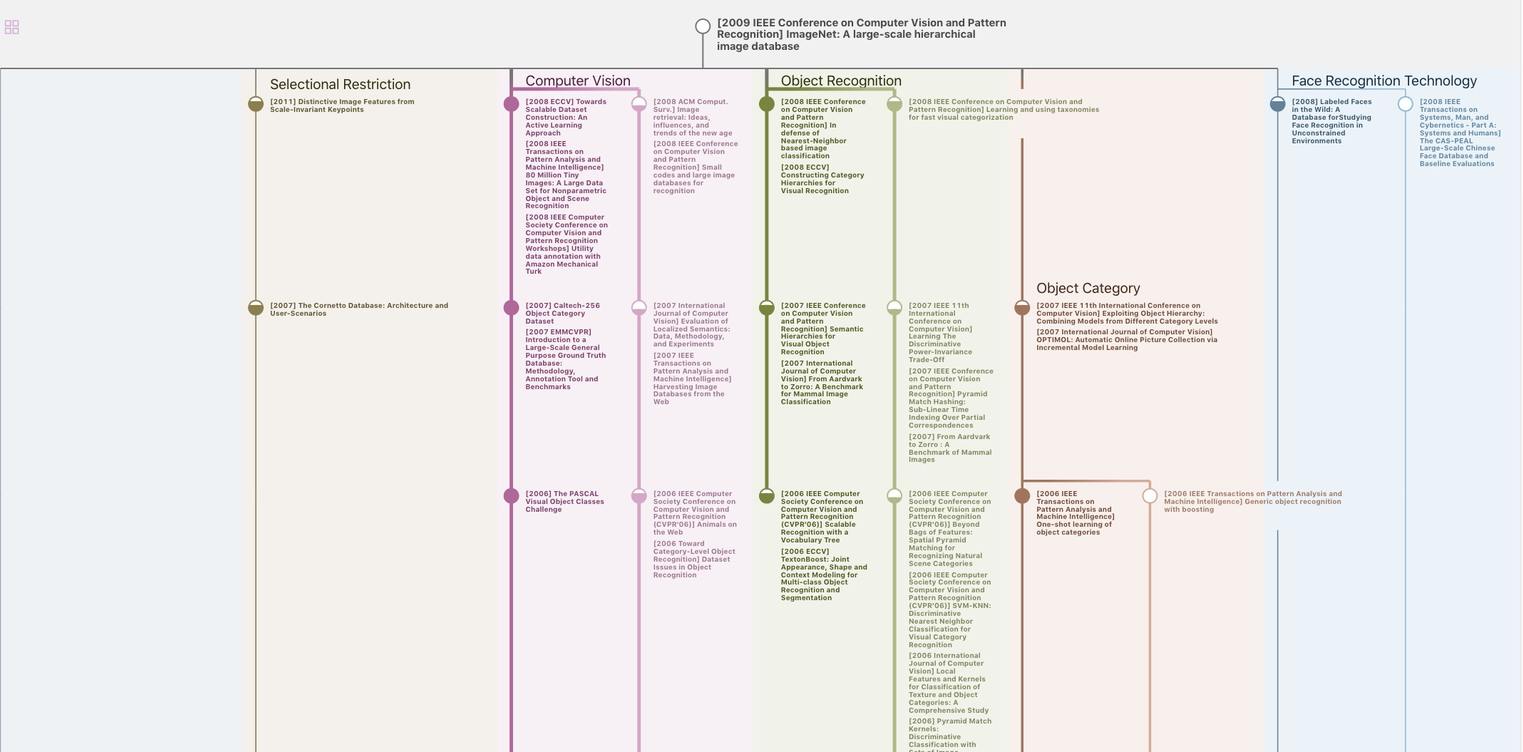
生成溯源树,研究论文发展脉络
Chat Paper
正在生成论文摘要