A survey of deep nonnegative matrix factorization
Neurocomputing(2022)
摘要
Deep Nonnegative Matrix Factorization (Deep NMF) is an effective strategy for feature extraction in recent years. By decomposing the matrix recurrently on account of the NMF algorithms, we obtain a hierarchical neural network structure as well as exploring more interpretable representations of the data. This paper mainly focuses on some theoretical researches with respect to Deep NMF, where the basic models, optimization methods, properties together with its extensions and generalizations are included systematically. We partition the Deep NMF algorithms into five categories: Deep NMF, Constrained Deep NMF, Generalized Deep NMF, Multi-View Deep Matrix Factorization (MF), the association between deep neural network (DNN) and NMF. Besides, we investigate the clustering performance of Deep NMF Algorithms on some face databases. Then the design principles, major steps, relationships, application domains and evolution of Deep NMF methods are comprehensively analyzed. Moreover, some open problems of Deep NMF are discussed.
更多查看译文
关键词
Deep nonnegative matrix factorization,Feature extraction,Deep neural network
AI 理解论文
溯源树
样例
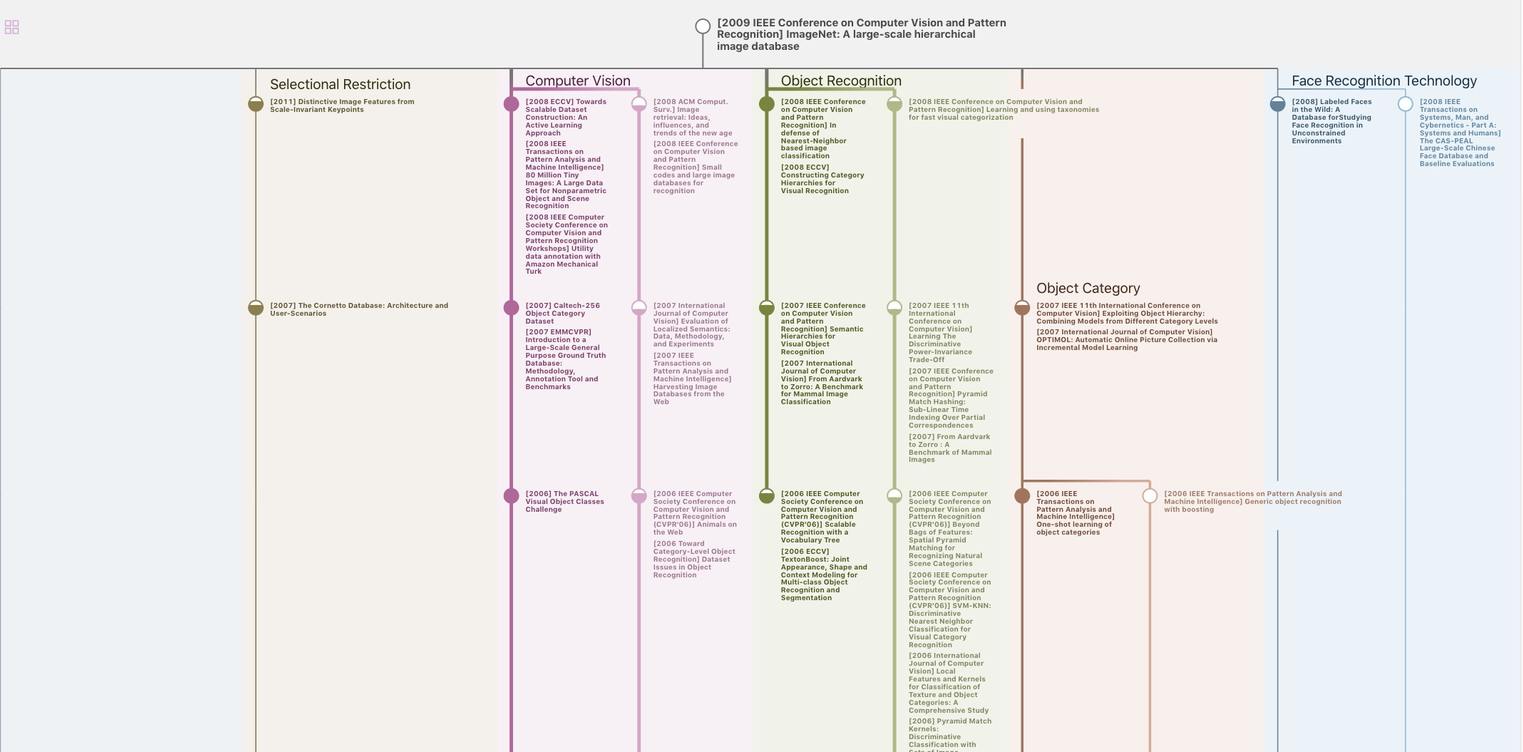
生成溯源树,研究论文发展脉络
Chat Paper
正在生成论文摘要