BiTimeBERT: Extending Pre-Trained Language Representations with Bi-Temporal Information
PROCEEDINGS OF THE 46TH INTERNATIONAL ACM SIGIR CONFERENCE ON RESEARCH AND DEVELOPMENT IN INFORMATION RETRIEVAL, SIGIR 2023(2023)
摘要
Time is an important aspect of documents and is used in a range of NLP and IR tasks. In this work, we investigate methods for incorporating temporal information during pre-training to further improve the performance on time-related tasks. Compared with common pre-trained language models like BERT which utilize synchronic document collections (e.g., BookCorpus and Wikipedia) as the training corpora, we use long-span temporal news article collection for building word representations. We introduce BiTimeBERT, a novel language representation model trained on a temporal collection of news articles via two new pre-training tasks, which harnesses two distinct temporal signals to construct time-aware language representations. The experimental results show that BiTimeBERT consistently outperforms BERT and other existing pre-trained models with substantial gains on different downstream NLP tasks and applications for which time is of importance (e.g., the accuracy improvement over BERT is 155% on the event time estimation task).(1)
更多查看译文
关键词
pre-trained language models,temporal information,news archive
AI 理解论文
溯源树
样例
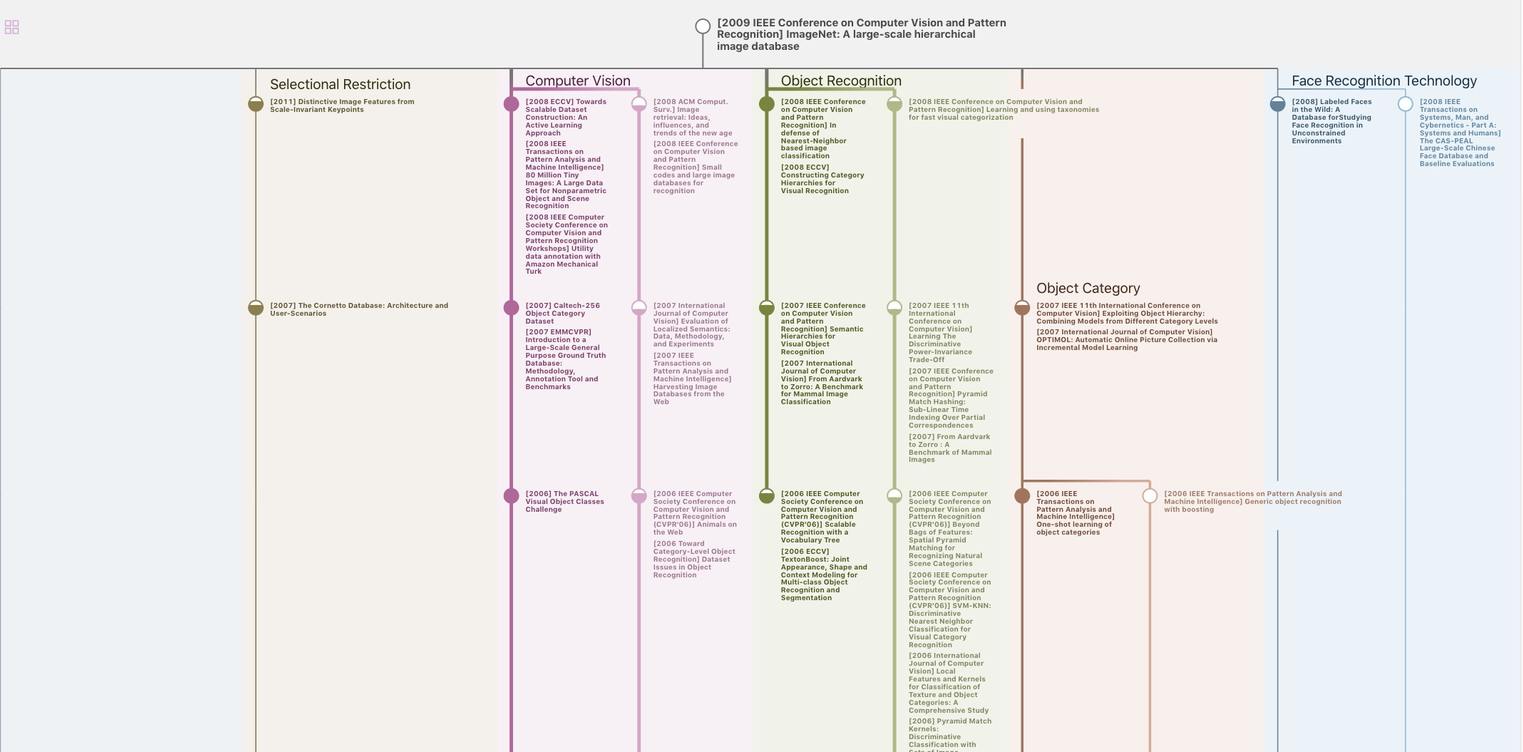
生成溯源树,研究论文发展脉络
Chat Paper
正在生成论文摘要