Multi-Task Learning-Based CSI Feedback Design in Multiple Scenarios
IEEE TRANSACTIONS ON COMMUNICATIONS(2023)
摘要
For frequency division duplex (FDD) systems, downlink channel state information (CSI) feedback is essential. Deep learning-based auto-encoder (AE) structures have shown promise in reducing feedback overhead. However, designing a super-large AE network to handle the CSI of all scenarios is not practical. A more practical approach is to divide the CSI dataset by region/scenario and use multiple simple AE networks. However, this method requires high memory capacity, making it unsuitable for low-end user equipment (UE). In this paper, we propose a new UE-friendly framework based on multi-tasking mode. Our framework, called single-encoder-to-multiple-decoders (S-to-M), uses multi-task-learning to design multiple independent AEs into a joint architecture with a shared encoder that corresponds to multiple task-specific decoders. We also integrate GateNet as a classifier to enable the base station to autonomously select the right task-specific decoder for the subregion. Our experiments on a simulated multi-scenario CSI dataset show that our proposed S-to-M framework outperforms other benchmark modes by significantly reducing model complexity and UE memory consumption.
更多查看译文
关键词
Massive MIMO,CSI feedback,deep learning,multitask learning,multi-scenario
AI 理解论文
溯源树
样例
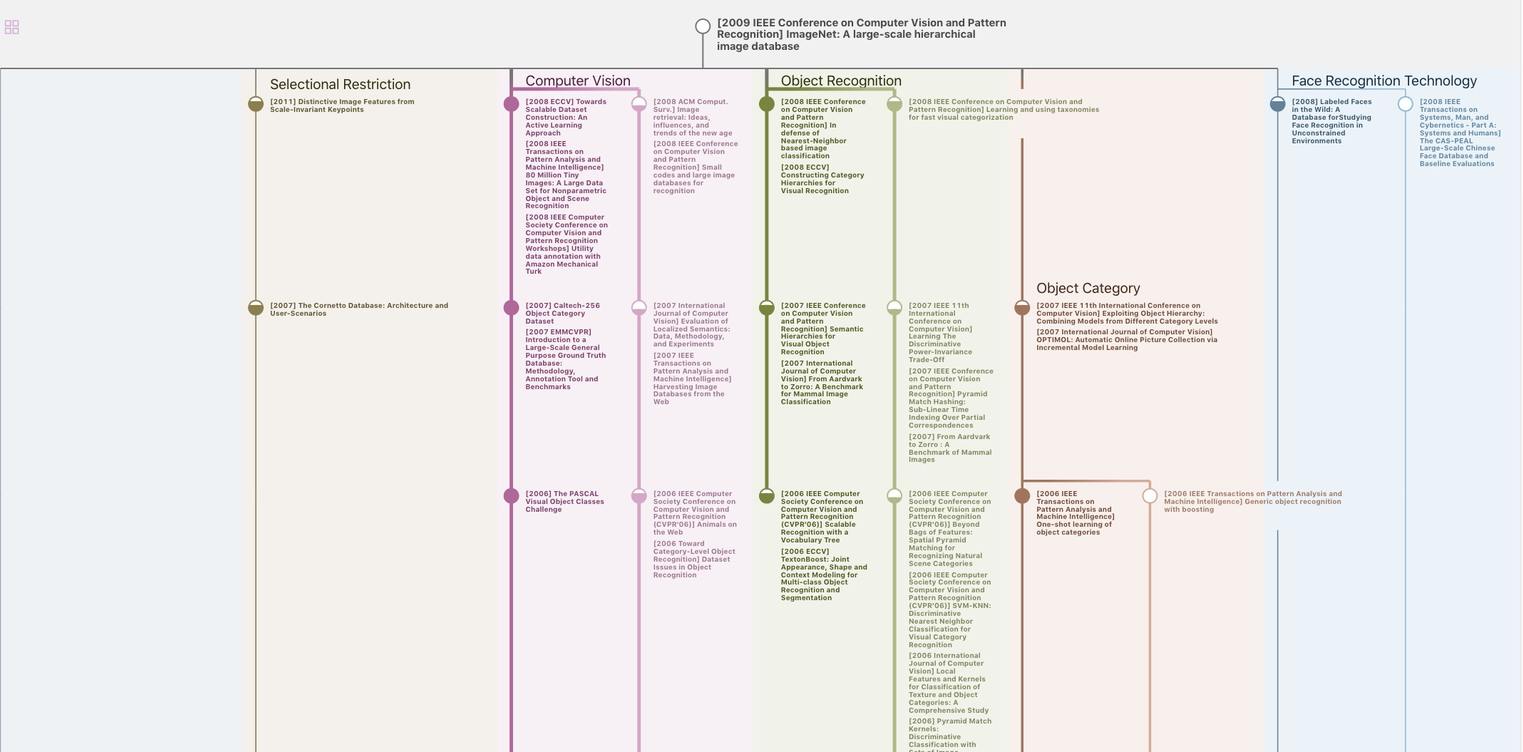
生成溯源树,研究论文发展脉络
Chat Paper
正在生成论文摘要