It Takes Two Flints to Make a Fire: Multitask Learning of Neural Relation and Explanation Classifiers
arxiv(2022)
摘要
We propose an explainable approach for relation extraction that mitigates the tension between generalization and explainability by jointly training for the two goals. Our approach uses a multi-task learning architecture, which jointly trains a classifier for relation extraction, and a sequence model that labels words in the context of the relation that explain the decisions of the relation classifier. We also convert the model outputs to rules to bring global explanations to this approach. This sequence model is trained using a hybrid strategy: supervised, when supervision from pre-existing patterns is available, and semi-supervised otherwise. In the latter situation, we treat the sequence model's labels as latent variables, and learn the best assignment that maximizes the performance of the relation classifier. We evaluate the proposed approach on the two datasets and show that the sequence model provides labels that serve as accurate explanations for the relation classifier's decisions, and, importantly, that the joint training generally improves the performance of the relation classifier. We also evaluate the performance of the generated rules and show that the new rules are great add-on to the manual rules and bring the rule-based system much closer to the neural models.
更多查看译文
关键词
multitask learning,neural
AI 理解论文
溯源树
样例
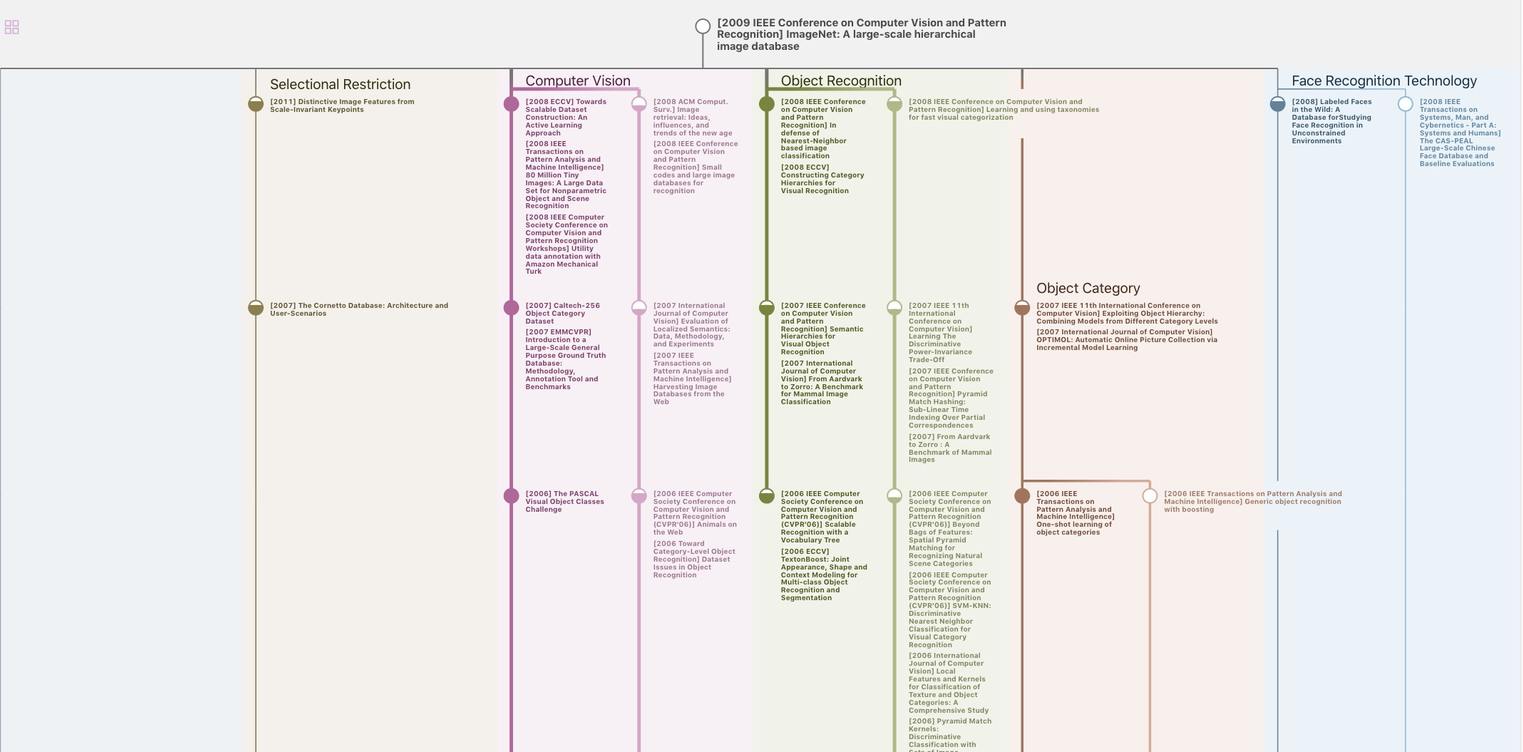
生成溯源树,研究论文发展脉络
Chat Paper
正在生成论文摘要