Deep Reinforcement Learning Using a Low-Dimensional Observation Filter for Visual Complex Video Game Playing
arxiv(2022)
摘要
Deep Reinforcement Learning (DRL) has produced great achievements since it was proposed, including the possibility of processing raw vision input data. However, training an agent to perform tasks based on image feedback remains a challenge. It requires the processing of large amounts of data from high-dimensional observation spaces, frame by frame, and the agent's actions are computed according to deep neural network policies, end-to-end. Image pre-processing is an effective way of reducing these high dimensional spaces, eliminating unnecessary information present in the scene, supporting the extraction of features and their representations in the agent's neural network. Modern video-games are examples of this type of challenge for DRL algorithms because of their visual complexity. In this paper, we propose a low-dimensional observation filter that allows a deep Q-network agent to successfully play in a visually complex and modern video-game, called Neon Drive.
更多查看译文
关键词
deep reinforcement learning,observation,low-dimensional
AI 理解论文
溯源树
样例
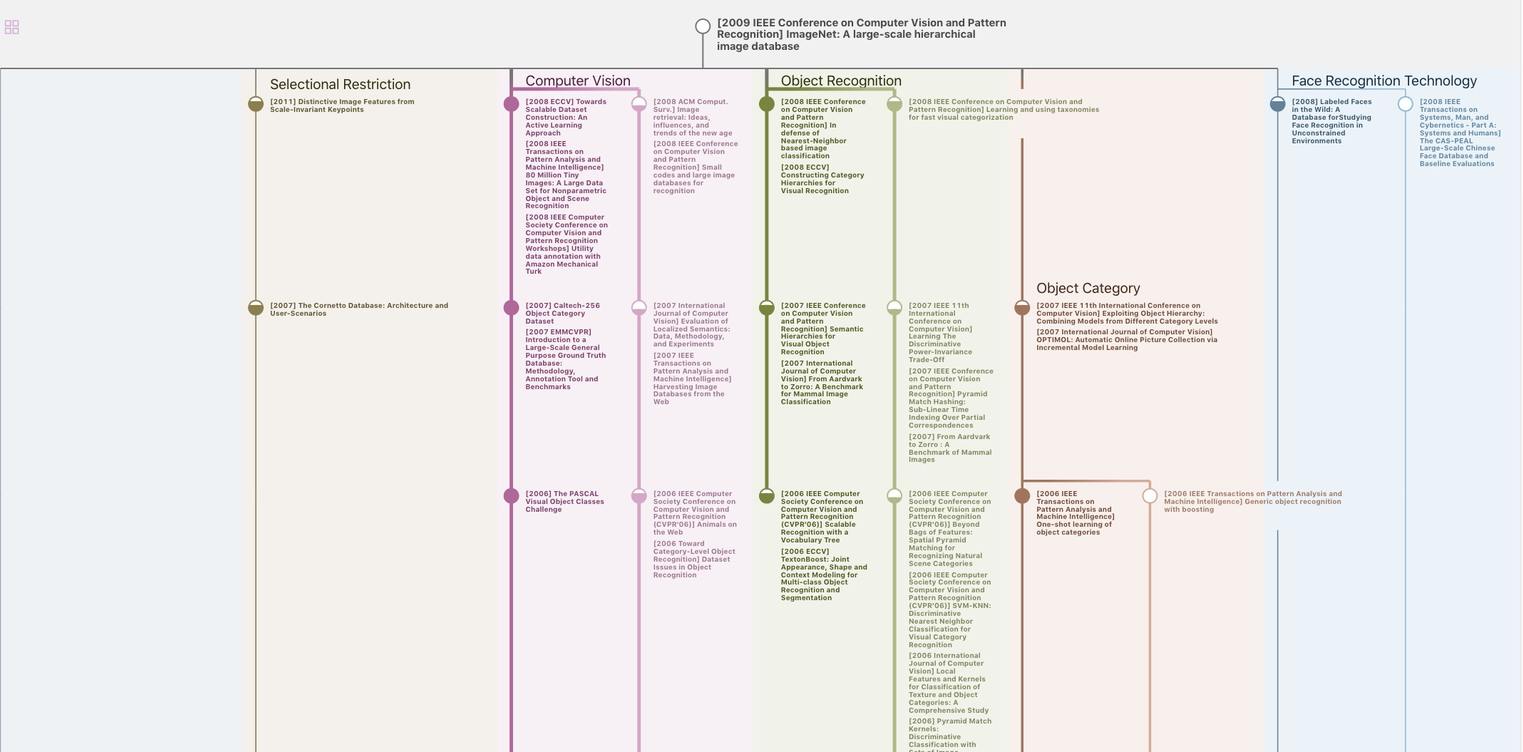
生成溯源树,研究论文发展脉络
Chat Paper
正在生成论文摘要