Generative Sampling in Bundle Tractography using Autoencoders (GESTA)
arxiv(2023)
摘要
Current tractography methods use the local orientation information to propagate streamlines from seed locations. Many such seeds provide streamlines that stop prematurely or fail to map the true white matter pathways because some bundles are "harder-to-track" than others. This results in tractography reconstructions with poor white and gray matter spatial coverage. In this work, we propose a generative, autoencoder-based method, named GESTA (Generative Sampling in Bundle Tractography using Autoencoders), that produces streamlines achieving better spatial coverage. Compared to other deep learning methods, our autoencoder-based framework uses a single model to generate streamlines in a bundle-wise fashion, and does not require to propagate local orientations. GESTA produces new and complete streamlines for any given white matter bundle, including hard-to-track bundles. Applied on top of a given tractogram, GESTA is shown to be effective in improving the white matter volume coverage in poorly populated bundles, both on synthetic and human brain in vivo data. Our streamline evaluation framework ensures that the streamlines produced by GESTA are anatomically plausible and fit well to the local diffusion signal. The streamline evaluation criteria assess anatomy (white matter coverage), local orientation alignment (direction), and geometry features of streamlines, and optionally, gray matter connectivity. GESTA is thus a novel deep generative bundle tractography method that can be used to improve the tractography reconstruction of the white matter.
更多查看译文
关键词
62M20,62M45,68T01,68U10,92C55
AI 理解论文
溯源树
样例
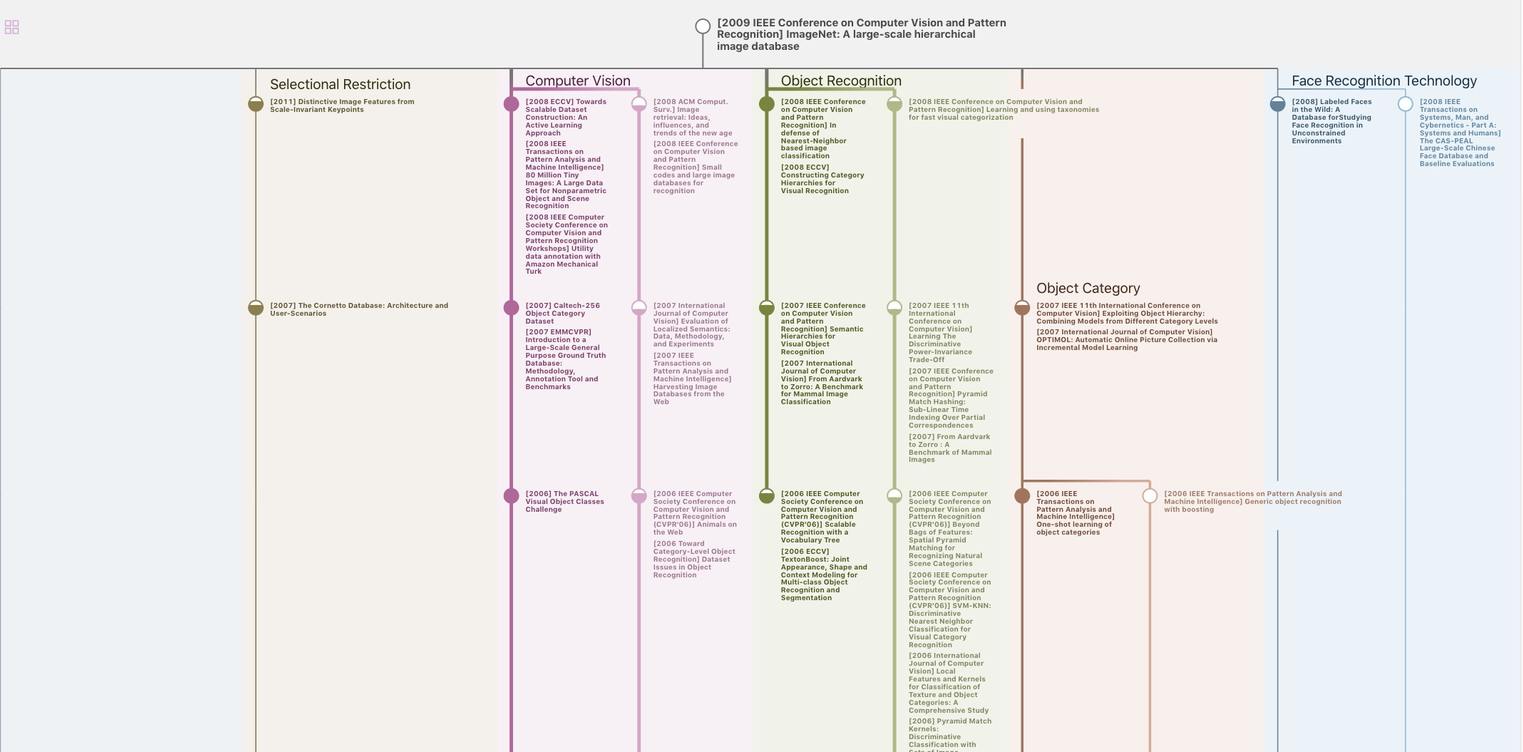
生成溯源树,研究论文发展脉络
Chat Paper
正在生成论文摘要