Neural Networks with Local Converging Inputs (NNLCI) for Solving Conservation Laws, Part II: 2D Problems
arxiv(2022)
摘要
In our prior work [arXiv:2109.09316], neural network methods with inputs based on domain of dependence and a converging sequence were introduced for solving one dimensional conservation laws, in particular the Euler systems. To predict a high-fidelity solution at a given space-time location, two solutions of a conservation law from a converging sequence, computed from low-cost numerical schemes, and in a local domain of dependence of the space-time location, serve as the input of a neural network. In the present work, we extend the methods to two dimensional Euler systems and introduce variations. Numerical results demonstrate that the methods not only work very well in one dimension [arXiv:2109.09316], but also perform well in two dimensions. Despite smeared local input data, the neural network methods are able to predict shocks, contacts, and smooth regions of the solution accurately. The neural network methods are efficient and relatively easy to train because they are local solvers.
更多查看译文
关键词
neural networks,conservation laws,local converging inputs,2d problems
AI 理解论文
溯源树
样例
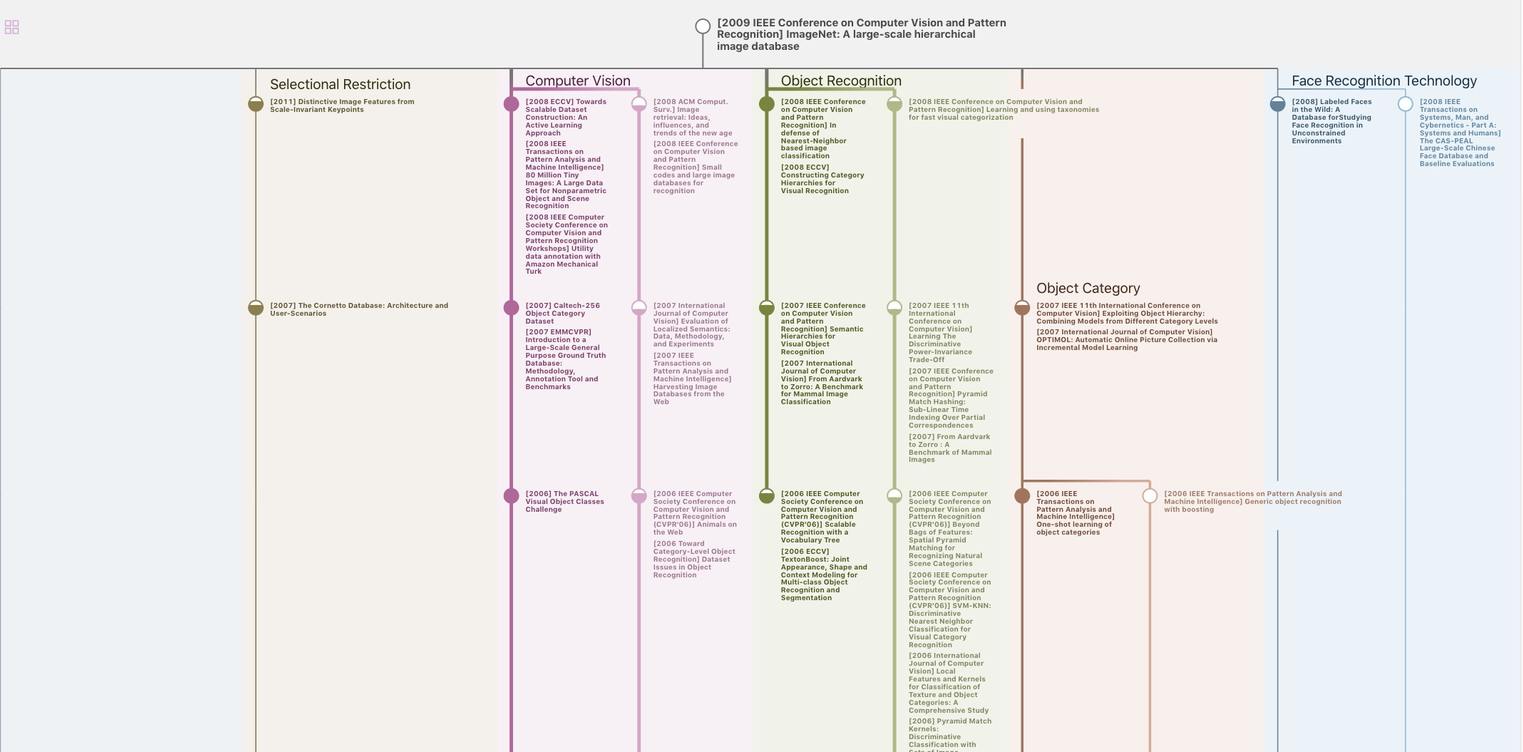
生成溯源树,研究论文发展脉络
Chat Paper
正在生成论文摘要