Discrete Infomax Codes for Supervised Representation Learning
ENTROPY(2022)
摘要
For high-dimensional data such as images, learning an encoder that can output a compact yet informative representation is a key task on its own, in addition to facilitating subsequent processing of data. We present a model that produces discrete infomax codes (DIMCO); we train a probabilistic encoder that yields k-way d-dimensional codes associated with input data. Our model maximizes the mutual information between codes and ground-truth class labels, with a regularization which encourages entries of a codeword to be statistically independent. In this context, we show that the infomax principle also justifies existing loss functions, such as cross-entropy as its special cases. Our analysis also shows that using shorter codes reduces overfitting in the context of few-shot classification, and our various experiments show this implicit task-level regularization effect of DIMCO. Furthermore, we show that the codes learned by DIMCO are efficient in terms of both memory and retrieval time compared to prior methods.
更多查看译文
关键词
infomax, discrete codes, representation learning, few-shot classification
AI 理解论文
溯源树
样例
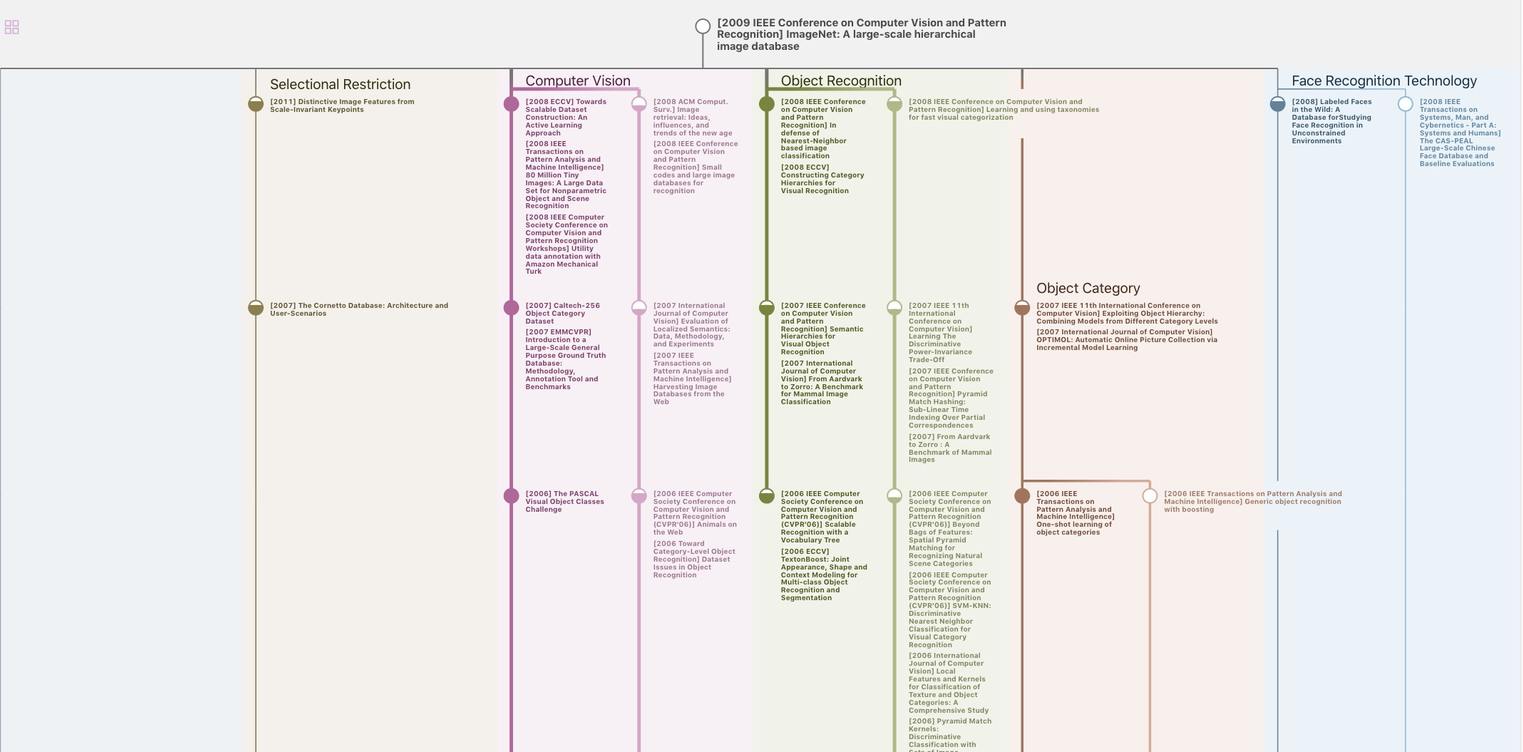
生成溯源树,研究论文发展脉络
Chat Paper
正在生成论文摘要