Physiologically-Informed Gaussian Processes for Interpretable Modelling of Psycho-Physiological States
IEEE journal of biomedical and health informatics(2023)
摘要
The widespread popularity of Machine Learning (ML) models in healthcare solutions has increased the demand for their interpretability and accountability. In this paper, we propose the
Physiologically-Informed Gaussian Process
(
PhGP
) classification model, an interpretable machine learning model founded on the Bayesian nature of Gaussian Processes (GPs). Specifically, we inject problem-specific domain knowledge of inherent physiological mechanisms underlying the psycho-physiological states as a prior distribution over the GP latent space. Thus, to estimate the hyper-parameters in
PhGP
, we rely on the information from raw physiological signals as well as the designed prior function encoding the physiologically-inspired modelling assumptions. Alongside this new model, we present novel interpretability metrics that highlight the most informative input regions that contribute to the GP prediction. We evaluate the ability of
PhGP
to provide an accurate and interpretable classification on three different datasets, including electrodermal activity (EDA) signals collected during emotional, painful, and stressful tasks. Our results demonstrate that, for all three tasks, recognition performance is improved by using the
PhGP
model compared to competitive methods. Moreover,
PhGP
is able to provide physiological sound interpretations over its predictions.
更多查看译文
关键词
Gaussian process,interpretable machine learning,electrodermal activity,bayesian learning,psycho-physiology
AI 理解论文
溯源树
样例
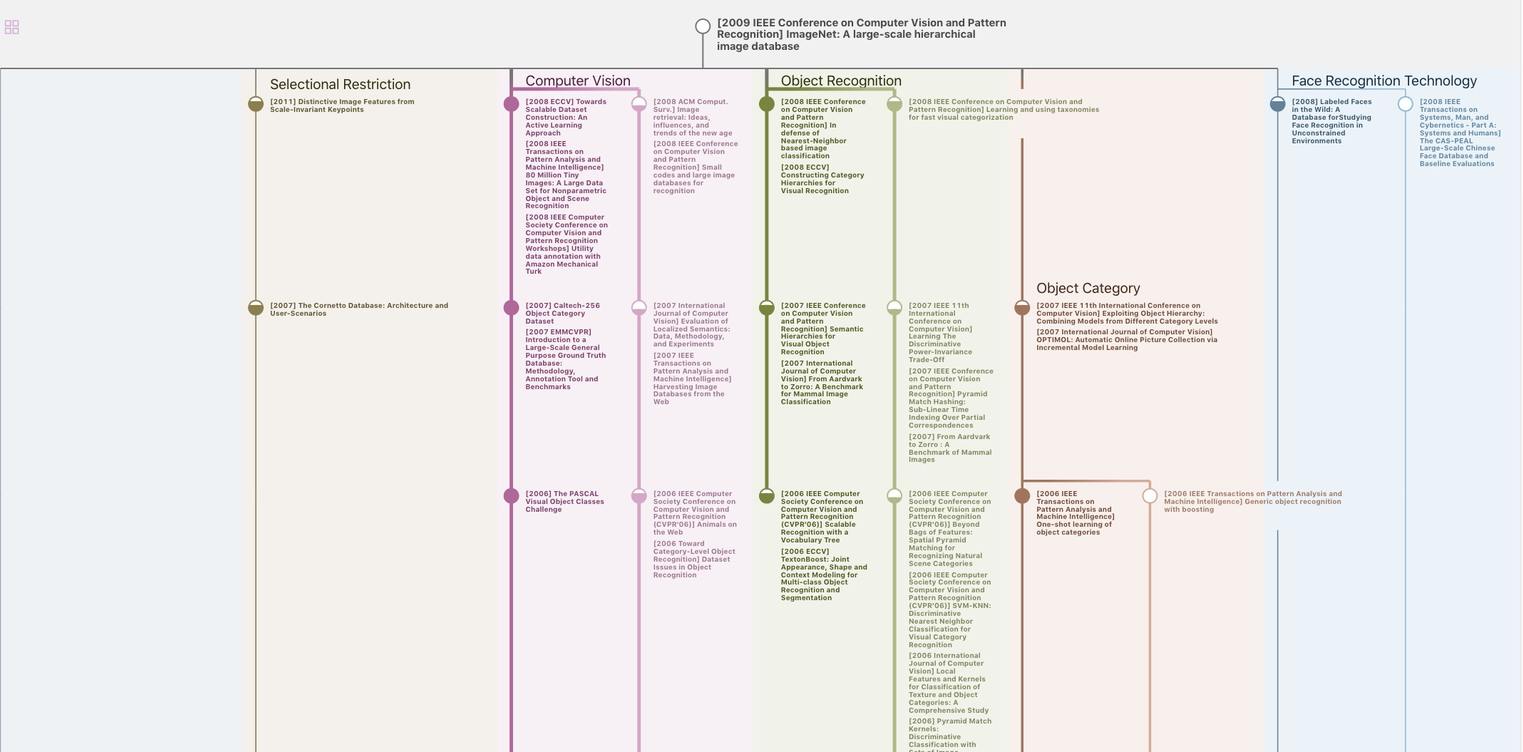
生成溯源树,研究论文发展脉络
Chat Paper
正在生成论文摘要