Learning to Fold Real Garments with One Arm: A Case Study in Cloud-Based Robotics Research
2022 IEEE/RSJ International Conference on Intelligent Robots and Systems (IROS)(2022)
摘要
Autonomous fabric manipulation is a longstanding challenge in robotics, but evaluating progress is difficult due to the cost and diversity of robot hardware. Using Reach, a cloud robotics platform that enables low-latency remote execution of control policies on physical robots, we present the first systematic benchmarking of fabric manipulation al-gorithms on physical hardware. We develop 4 novel learning-based algorithms that model expert actions, keypoints, reward functions, and dynamic motions, and we compare these against 4 learning-free and inverse dynamics algorithms on the task of folding a crumpled T-shirt with a single robot arm. The entire lifecycle of data collection, model training, and policy evaluation was performed remotely without physical access to the robot workcell. Results suggest a new algorithm combining imitation learning with analytic methods achieves human-level performance on the flattening task and 93% of human-level performance on the folding task. See https://sites.google.com/berkeley.edu/ cloudfolding for all data, code, models, and supplemental material.
更多查看译文
关键词
robotics research,real garments,learning,cloud-based
AI 理解论文
溯源树
样例
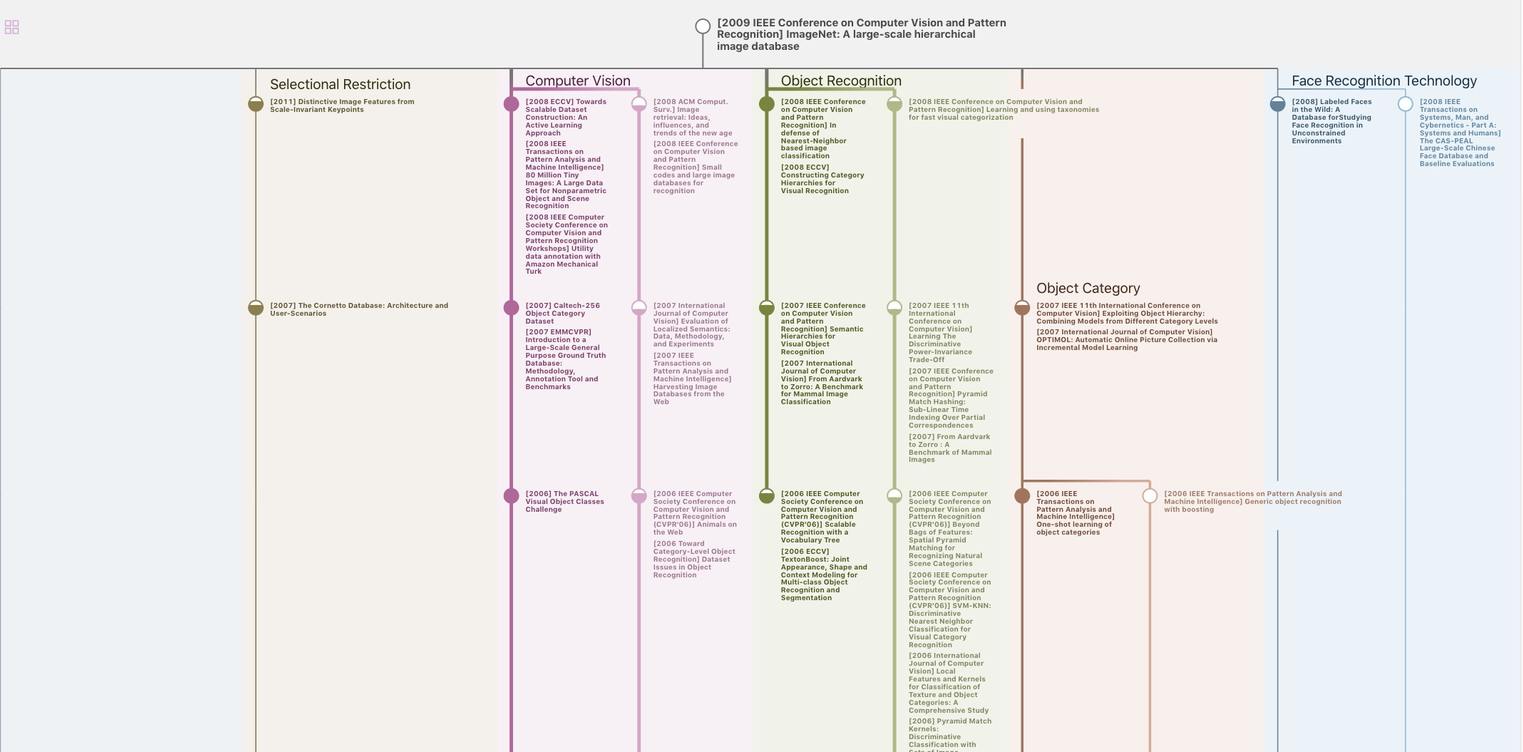
生成溯源树,研究论文发展脉络
Chat Paper
正在生成论文摘要