An Attention-Based Model for Predicting Contextual Informativeness and Curriculum Learning Applications
arxiv(2022)
摘要
Both humans and machines learn the meaning of unknown words through contextual information in a sentence, but not all contexts are equally helpful for learning. We introduce an effective method for capturing the level of contextual informativeness with respect to a given target word. Our study makes three main contributions. First, we develop models for estimating contextual informativeness, focusing on the instructional aspect of sentences. Our attention-based approach using pre-trained embeddings demonstrates state-of-the-art performance on our single-context dataset and an existing multi-sentence context dataset. Second, we show how our model identifies key contextual elements in a sentence that are likely to contribute most to a reader's understanding of the target word. Third, we examine how our contextual informativeness model, originally developed for vocabulary learning applications for students, can be used for developing better training curricula for word embedding models in batch learning and few-shot machine learning settings. We believe our results open new possibilities for applications that support language learning for both human and machine learners
更多查看译文
关键词
contextual informativeness,curriculum,learning,attention-based
AI 理解论文
溯源树
样例
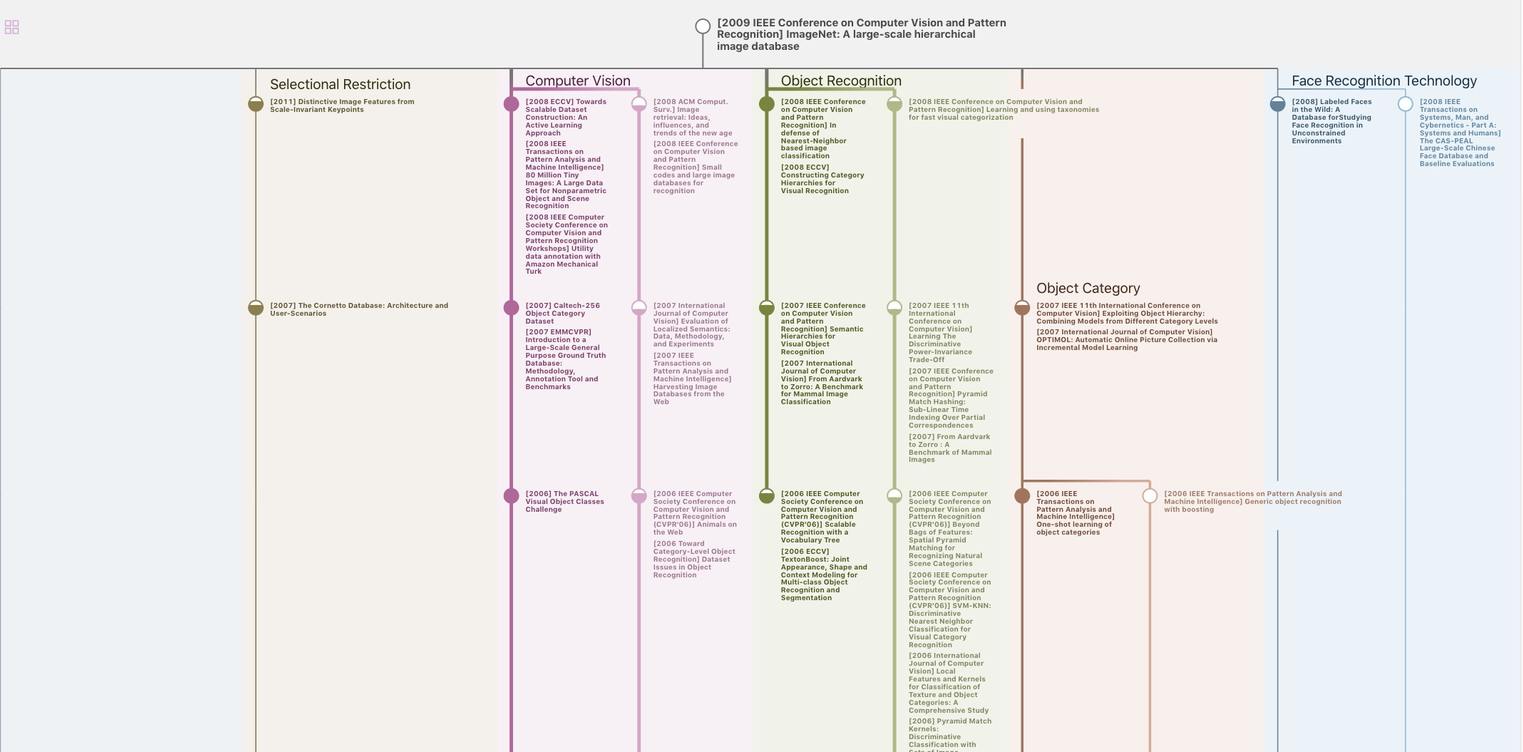
生成溯源树,研究论文发展脉络
Chat Paper
正在生成论文摘要