Distantly Supervised Named Entity Recognition via Confidence-Based Multi-Class Positive and Unlabeled Learning
PROCEEDINGS OF THE 60TH ANNUAL MEETING OF THE ASSOCIATION FOR COMPUTATIONAL LINGUISTICS (ACL 2022), VOL 1: (LONG PAPERS)(2022)
摘要
In this paper, we study the named entity recognition (NER) problem under distant supervision. Due to the incompleteness of the external dictionaries and/or knowledge bases, such distantly annotated training data usually suffer from a high false negative rate. To this end, we formulate the Distantly Supervised NER (DS-NER) problem via Multi-class Positive and Unlabeled (MPU) learning and propose a theoretically and practically novel CONFidence-based MPU (Conf-MPU) approach. To handle the incomplete annotations, Conf-MPU consists of two steps. First, a confidence score is estimated for each token of being an entity token. Then, the proposed Conf-MPU risk estimation is applied to train a multi-class classifier for the NER task. Thorough experiments on two benchmark datasets labeled by various external knowledge demonstrate the superiority of the proposed Conf-MPU over existing DS-NER methods. Our code is available at Github(1).
更多查看译文
关键词
named entity recognition,unlabeled learning,confidence-based,multi-class
AI 理解论文
溯源树
样例
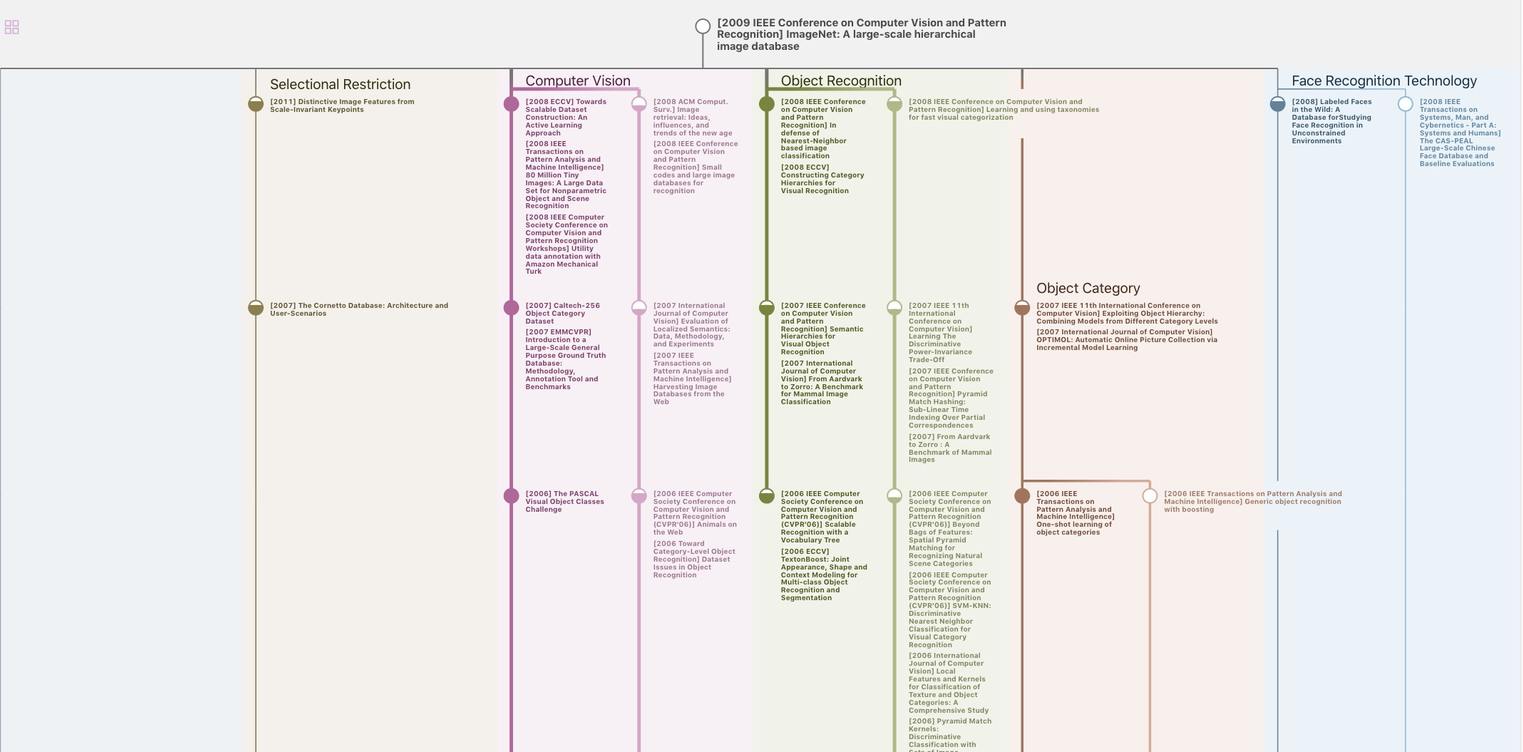
生成溯源树,研究论文发展脉络
Chat Paper
正在生成论文摘要